Modelling air pollution in Great Britain
- The text on this page is taken from an equivalent page of the IEHIAS-project.
Summary
A NO2 LUR Model for Great Britain (GB) was constructed on the basis of monitored data representing annual mean concentrations of NO2 for the year 2001, derived from routine measurement sites from the national air quality network. Predictor variables used for modelling related to traffic, land use, topography and population. Data on the predictor variables were integrated in a GIS, and converted to 100x100m grids (raster). Using a “supervised stepwise regression” approach a best model was constructed and applied to the relevant predictor grids and a NO2 map for GB was created. This example is part of a publication (Vienneau et al., 2009) which compares LUR models between Great Britain and the Netherlands.
For each of the predictors (roads, traffic, land cover, population), two sets of variables were obtained for use in the modelling. The first comprised ‘zero-centred’ variables, created by calculating the sum of each cell value in buffer zones of increasing radius around the air pollution monitoring sites (0-100 m, 0-200 m…) using the Focalsum command with the circle option in ArcGIS. In total this gave 90 predictor variables. The second comprised ‘ring’ variables. These were calculated, as required during modelling, by differencing to give the sums for each predictor variable for the intermediate or outer rings (e.g. 100-200 m, 100-300 m, 200-300 m...).
Data
Monitoring data
Monitoring data from the national network were supplemented with data from networks run by local authorities. This gave a database comprising 156 monitoring sites for NO2 of which 39 sites were rural, 64 urban and 53 traffic sites. The mean NO2 concentration measured was 41.1 µg/m3 (SD = 14.9 µg/m3, min = 12.1 µg/m3, max = 86.0 µg/m3).
Predictor variables
Altitude: Altitude data were taken from the 50 m Ordnance Survey PANORAMATM Digital Terrain Model (DTM). The mean altitude was calculated in ArcGIS by aggregating these raw data to a resolution of 100 m. Altitude was offered as v(nalt/max(nalt)), where nalt=altitude-min(altitude) (Beelen et al. 2009). Topographic exposure, or Topex, was also calculated to provide a measure of the openness of terrain. It was computed as the difference in altitude between each 100 m centroid and the mean altitude of the surrounding cells in either a 1000 or 6000 metre buffer.
Regional trend: To reflect broad scale trends in background concentrations of air pollution, the X and Y co-ordinates for the centroids of each grid cell were also included as potential predictor variables.
Land cover: Land cover data were derived from the CORINE Land Cover Map 2000. Data from the 1:100 000 country vector data sets were used, with a notional accuracy of ca. 100 metres. The original CORINE categories were regrouped by summation into six urban classes (high density residential, low density residential, industry, ports, urban green spaces, industry plus ports) and one rural class (semi-natural plus forested areas). The area (in m2) for the seven classes was calculated for each grid cell for buffers of 100, 200, 300, 500, 1000 and 3000m. The total area of built-up land (original CORINE classes 1-9) within a 20 km buffer was also calculated using the Focalsum function in ArcGIS to produce a variable representing urban influence.
Population: Population data comprised headcounts from the 2001 census, at postcode level (ONS 2004): a postcode comprises, on average 12-15 properties. Data were converted to 100 m grids by intersecting the postcode locations with the base grid and summing the population count within each grid cell for buffers of 100, 200, 300, 500, 1000 and 3000m.
Road and traffic data: The digital data on the road network was obtained from the Ordnance Survey 1:50 000 Meridian™ data set, and has a spatial accuracy of ca 1m. Roads were classified into four types: motorways, A-roads (single or dual carriageways with a speed limit of 60 or 70 mph, respectively), B roads (single carriageways with a speed limit of 60 mph) and minor roads (urban streets or country lanes). Traffic intensity data were obtained from the Department of Environment Food and Rural Affairs (DEFRA) in the form of total traffic counts (AADTF, Annual Average Daily Traffic Flows) for 2001, at point locations across the country, covering all motorways and A-roads. The traffic intensities were automatically matched to the digital road data, then gridded to a 100 m resolution for buffers of 100, 200 and 300m. The length of each road class within 100 m grids was also calculated for the same buffer sizes.
Regression modelling
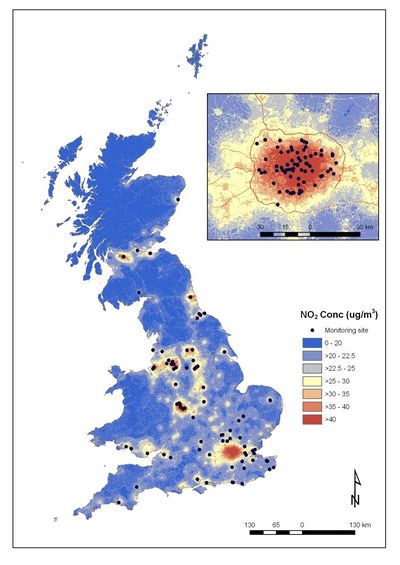
The following guidelines were used creating the LUR models. This process was done in SPSS.
- Enter explanatory variables in a ‘supervised-stepwise manner’, most important predictors first.
- The sign for each coefficient in the model must conform to the expected direction of effect.
- Each variable in the model should be significant (e.g. p < 0.05).
- Following point 1, variables entered later in the process should not be maintained if they cause variables already in the model to invalidate guidelines 2 or 3.
- Avoid double counting by excluding overlapping buffers. For example, including roads in 0-100m and 100-200m is valid, but including roads in 0-100m and 0-200m is not.
- Gaps in the buffers should be avoided. For example, roads in 100-200m should not be included unless roads in 0-100m is already in your model.
Results
The final NO2 model is shown in Table 1, also showing the increment in adjusted R2 of each added predictor variable. The LUR model was validated through cross validation, using the leave-one-out approach.
Model Building | Validation | |||||
Variables | β | p | Adj R2 | SEE | Adj R2 | RMSE |
(Constant) | 26.22 | 0.00 | ||||
Urban influence 20km | 1.30E-07 | 0.00 | 0.46 | |||
High density residential 0-3000m | 1.32E-06 | 0.00 | 0.54 | |||
Major road length (motor + A) 0-100m | 5.20E-02 | 0.00 | 0.61 | |||
Bus and HGV flow 0-300m | 2.07E-06 | 0.01 | 0.62 | |||
Altitude (transformed) | -2.36E+01 | 0.01 | 0.63 | 9.02 | 0.61 | 9.26 |
The final LUR model can then be applied using the Raster Calculator Tool in ArcGIS and the relevant grids held in the GIS to create a 100 x 100 m NO2 concentration map (see Figure 1). The range of predicted concentrations from the map was compared to that of the monitored data, and the spatial distributions of the predicted concentrations visually examined to assess the plausibility of the map and identify any extreme predictions.
References
- Vienneau, D., de Hoogh, K., Beelen, R., Fischer, P., Hoek, G. and Briggs, D. 2009 Comparison of land-use regression models between Great Britain and the Netherlands. Atmospheric Environment44, 688-696
- Beelen, R., Hoek, G., Pebesma, E., Vienneau, D., de Hoogh, K. and Briggs, D.J. 2009 Mapping of background air pollution at a fine spatial scale across the European Union. Science of the Total Environment 407(6), 1852-1867.