EU age/sex stratified population: 100 metre grid: Difference between revisions
No edit summary |
mNo edit summary |
||
(3 intermediate revisions by one other user not shown) | |||
Line 28: | Line 28: | ||
Data are based on ETRS89 Lambert Azimuthal Equal Area projection with parameters: latitude of origin 52° N, longitude of origin 10° E, false northing 3 210 000.0 m, false easting 4 321 000.0 m. | Data are based on ETRS89 Lambert Azimuthal Equal Area projection with parameters: latitude of origin 52° N, longitude of origin 10° E, false northing 3 210 000.0 m, false easting 4 321 000.0 m. | ||
* | If a country only has a single zip file (e.g. be.zip or dk_0.zip), you can simply unzip it and use. However, if a country is large, it has several zip files, which are zipped twice. First, you have to unzip all files of a country (e.g. at.zip.001.zip and at.zip.002.zip). This will produce several volumes of a larger zip file (e.g. at.zip which has volumes at.zip.001 and at.zip.002). Merge these volumes and then unzip the country file at.zip to produce the actual ascii files. | ||
* | |||
* | We recommend that you use 7zip program for unzipping! | ||
* | |||
* | * Austria {{#l:at.zip.001.zip}}{{#l:at.zip.002.zip}} | ||
* | * Belgium {{#l:be.zip}} | ||
* | * Czech Republic {{#l:cz_0.zip.001.zip}}{{#l:cz_0.zip.002.zip}} | ||
* | * Denmark {{#l:dk_0.zip}} | ||
* | * Estonia {{#l:ee_0.zip}} | ||
* | * Finland {{#l:fi_0.zip.001.zip}}{{#l:fi_0.zip.002.zip}}{{#l:fi_0.zip.003.zip}} | ||
* | * France {{#l:fr.zip.001.zip}}{{#l:fr.zip.002.zip}}{{#l:fr.zip.003.zip}}{{#l:fr.zip.004.zip}}{{#l:fr.zip.005.zip}}{{#l:fr.zip.006.zip}}{{#l:fr.zip.007.zip}}{{#l:fr.zip.008.zip}}{{#l:fr.zip.009.zip}}{{#l:fr.zip.010.zip}} | ||
* | * Germany {{#l:de.zip.001.zip}}{{#l:de.zip.002.zip}}{{#l:de.zip.003.zip}}{{#l:de.zip.004.zip}}{{#l:de.zip.005.zip}}{{#l:de.zip.006.zip}} | ||
* | * Great Britain {{#l:gb_0.zip.001.zip}}{{#l:gb_0.zip.002.zip}}{{#l:gb_0.zip.003.zip}} | ||
* | * Greece {{#l:gr_0.zip.001.zip}}{{#l:gr_0.zip.002.zip}} | ||
* | * Hungary {{#l:hu_0.zip.001.zip}}{{#l:hu_0.zip.002.zip}} | ||
* | * Ireland {{#l:ie_0.zip}} | ||
* | * Italy {{#l:it.zip.001.zip}}{{#l:it.zip.002.zip}}{{#l:it.zip.003.zip}}{{#l:it.zip.004.zip}}{{#l:it.zip.005.zip}} | ||
* | * Lithuania {{#l:lt_0.zip}} | ||
* | * Luxembourg {{#l:lu_0.zip}} | ||
* | * Malta {{#l:mt_0.zip}} | ||
* | * the Netherlands {{#l:nl_0.zip}} | ||
* | * Norway? Northern Ireland? {{#l:ni_0.zip}} | ||
* | * Poland {{#l:pl_0.zip.001.zip}}{{#l:pl_0.zip.002.zip}}{{#l:pl_0.zip.003.zip}}{{#l:pl_0.zip.004.zip}} | ||
* | * Portugal {{#l:pt_0.zip}} | ||
* Slovakia {{#l:sk.zip}} | |||
* Slovenia {{#l:si_0.zip}} | |||
* Spain {{#l:es_0.zip.001.zip}}{{#l:es_0.zip.002.zip}}{{#l:es_0.zip.003.zip}}{{#l:es_0.zip.004.zip}}{{#l:es_0.zip.005.zip}}{{#l:es_0.zip.006.zip}} | |||
* Sweden {{#l:se_0.zip.001.zip}}{{#l:se_0.zip.002.zip}}{{#l:se_0.zip.003.zip}} | |||
*[http://www.sciencedirect.com/science/article/pii/S0034425706005037 Dasymetric modelling of small-area population distribution using land cover and light emissions data. Remote Sensing of Environment.] Briggs D.J., Gulliver J., Fecht D. and Vienneau D.M. 2007. 108(4):451-466. | *[http://www.sciencedirect.com/science/article/pii/S0034425706005037 Dasymetric modelling of small-area population distribution using land cover and light emissions data. Remote Sensing of Environment.] Briggs D.J., Gulliver J., Fecht D. and Vienneau D.M. 2007. 108(4):451-466. | ||
*[http://link.springer.com/article/10.1007%2Fs11111-010-0108-y A population density grid of the European Union, Population and Environment]. Gallego F.J. 2010. 31: 460-473. | *[http://link.springer.com/article/10.1007%2Fs11111-010-0108-y A population density grid of the European Union, Population and Environment]. Gallego F.J. 2010. 31: 460-473. | ||
==See also== | |||
{{IEHIAS}} |
Latest revision as of 19:02, 25 September 2014
- The text on this page is taken from an equivalent page of the IEHIAS-project.
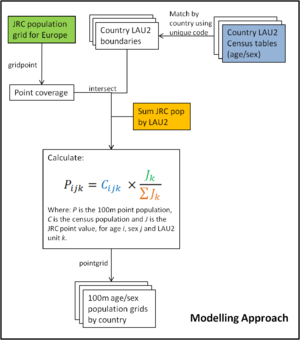
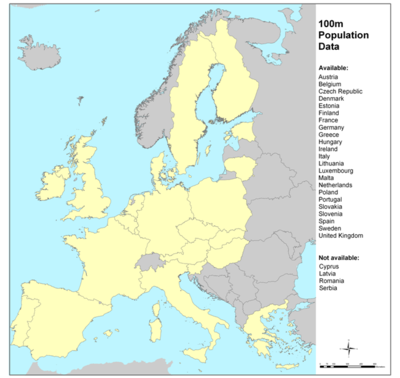
Even the highest resolution, LAU 2 level population data may be too coarse for some health risk assessments. Spatial modelling, therefore, may be required to disaggregate these totals to a finer grid. This has been done in several projects using land cover data as a basis for disaggregation (Briggs et al. 2007; Gallego 2010). Here the JRC population density grid (Gallego 2010) was enhanced by incorporating the age categories for both males and females from the census.
The modelling approach is illustrated below. Modelling involved proportioning the LAU2 level age/sex stratified data to the 100m cell on the basis of the JRC grid. Up to 28 grids (14 male and 14 female) were produced for each country depending on the structure of the age groups in the source tables.
Warning: These data are presented here at the 100m to offer flexibility in computing small area estimates at different spatial scales, however should not be taken to reflect population counts at the 100m level. Due to the lack of small area population data such as postcodes across Europe, these data were only validated for GB. Correlations (r) for total population were 0.92, 0.91 and 0.86 at the district, ward and 1km level, respectively. No correlation was found at the output area level. Similar modelling with validation found comparable results (Briggs et al. 2007), indicating that the 100m estimates could be reasonably aggregated to the 1km level.
Source / Reference
Three main sources of data were used to create these population grids:
- Small area census data
- Small area administrative boundaries (EuroBoundaryMap 1:1,000,000 January 2006 release)
- JRC population grid (version 4)
See also
Instructions:
Each country zip file contains up to 28 ascii files. To import into ArcGIS use the ASCII TO RASTER tool, or for ArcInfo use ASCIIGRID with the FLOAT option to create floating point grids.
Data are based on ETRS89 Lambert Azimuthal Equal Area projection with parameters: latitude of origin 52° N, longitude of origin 10° E, false northing 3 210 000.0 m, false easting 4 321 000.0 m.
If a country only has a single zip file (e.g. be.zip or dk_0.zip), you can simply unzip it and use. However, if a country is large, it has several zip files, which are zipped twice. First, you have to unzip all files of a country (e.g. at.zip.001.zip and at.zip.002.zip). This will produce several volumes of a larger zip file (e.g. at.zip which has volumes at.zip.001 and at.zip.002). Merge these volumes and then unzip the country file at.zip to produce the actual ascii files.
We recommend that you use 7zip program for unzipping!
- Austria
at.zip.001
at.zip.002
- Belgium
be
- Czech Republic
cz_0.zip.001
cz_0.zip.002
- Denmark
dk_0
- Estonia
ee_0
- Finland
fi_0.zip.001
fi_0.zip.002
fi_0.zip.003
- France
fr.zip.001
fr.zip.002
fr.zip.003
fr.zip.004
fr.zip.005
fr.zip.006
fr.zip.007
fr.zip.008
fr.zip.009
fr.zip.010
- Germany
de.zip.001
de.zip.002
de.zip.003
de.zip.004
de.zip.005
de.zip.006
- Great Britain
gb_0.zip.001
gb_0.zip.002
gb_0.zip.003
- Greece
gr_0.zip.001
gr_0.zip.002
- Hungary
hu_0.zip.001
hu_0.zip.002
- Ireland
ie_0
- Italy
it.zip.001
it.zip.002
it.zip.003
it.zip.004
it.zip.005
- Lithuania
lt_0
- Luxembourg
lu_0
- Malta
mt_0
- the Netherlands
nl_0
- Norway? Northern Ireland?
ni_0
- Poland
pl_0.zip.001
pl_0.zip.002
pl_0.zip.003
pl_0.zip.004
- Portugal
pt_0
- Slovakia
sk
- Slovenia
si_0
- Spain
es_0.zip.001
es_0.zip.002
es_0.zip.003
es_0.zip.004
es_0.zip.005
es_0.zip.006
- Sweden
se_0.zip.001
se_0.zip.002
se_0.zip.003
- Dasymetric modelling of small-area population distribution using land cover and light emissions data. Remote Sensing of Environment. Briggs D.J., Gulliver J., Fecht D. and Vienneau D.M. 2007. 108(4):451-466.
- A population density grid of the European Union, Population and Environment. Gallego F.J. 2010. 31: 460-473.