From open assessment to shared understanding: practical experiences
This page is a nugget.
The page identifier is Op_en7836 | |
---|---|
Moderator:Jouni (see all) | |
| |
Upload data
|
Unlike most other pages in Opasnet, the nuggets have predetermined authors, and you cannot freely edit the contents. Note! If you want to protect the nugget you've created from unauthorized editing click here |
From open assessment to shared understanding: practical experiences is a manuscript of a scientific article. The main point is to offer a comprehensive summary of the methods developed at THL/environmental health to support informed societal decision making, and evaluate their use and usability in practical examples in 2006-2017. Manuscript is to be submitted to BMC Public Health as a technical advance article [1].
Title page
- Title: From open assessment to shared understanding: practical experiences
- List full names, institutional addresses and emails for all authors
- Jouni T. Tuomisto1, Mikko Pohjola2, Arja Asikainen1, Päivi Meriläinen1, Teemu Rintala3.
- National Institute for Health and Welfare, P.O.Box 95, 70701 Kuopio, Finland
- Santasport, Rovaniemi, Finland
- Aalto University, Espoo, Finland
- emails: jouni.tuomisto[]thl.fi, mikko.pohjola[]santasport.fi, arja.asikainen[]thl.fi, paivi.merilainen[]thl.fi, teemu.rintala.a[]gmail.com
- Corresponding author: Jouni Tuomisto
- Jouni T. Tuomisto1, Mikko Pohjola2, Arja Asikainen1, Päivi Meriläinen1, Teemu Rintala3.
TO DO
- Make a coherent ontology in Opasnet: Each term in the ontology should be found in Opasnet with a description. In addition, a specific link should be available to mean the object itself rather than its description. http://en.opasnet.org/entity/...? Make clear what things should link to Opasnet and what to Wikibase (if we get one running soon)
- Describe OPP using the new ontology and make a new graph out of that. Is this possible/necessary?
- All terms and principles should be described in Opasnet in their own pages. Use italics to refer to these pages.
- Upload Tuomstio 1999 thesis to Julkari. And Paakkila 1999
Letter to the Editor
Abstract
Background
Evidence-based decision making and better use of scientific information in societal decisions has been an area of development for decades but is still topical. A decision support work can be viewed from the perspective of information collection, synthesis, and flow between decision makers, experts, and stakeholders.
Methods
We give an overview of and describe practical experiences from the open policy practice method that has been developed in National Institute for Health and Welfare for more than a decade. Open assessments are online collaborative efforts to produce information for decision makers by utilising e.g. quantitative models, structured discussions, and knowledge crystals. Knowledge crystal is a web page that has a specific resarch question, and an answer is continually updated based on all available information. Shared understanding is used to motivate decision makers and stakeholders to common dialogue and inform about conclusions and remaining disagreements.
Results
Technically the methods and online tools work as expected, as demonstrated by the numerous assessments and policy support processes conducted. The approach improves the availability of information and especially of details. Acceptability of openness is ambivalent among experts: it is an important scientific principle, but it goes against many current publishing practices. However, co-creation and openness are megatrends that are changing decision making and the society at large. Against many experts' fears, open participation has not caused problems in performing high-quality assessments. In contrast, a key problem is to motivate more people, including experts and decision makers, to participate and share their views.
Conclusions
Shared understanding has proved to be a useful concept that guides policy processes toward more collaborative approach, whose purpose is wider understanding rather than winning. There is potential for merging open policy practice with other open science and open decision process tools. Active facilitation, community building and improving the user-friendliness of the tools were identified as key solutions for improving usability of the method in the future.
- Keywords
- environmental health, decision support, open assessment, open policy practice, shared understanding, modelling, online tools, policy, method development, evaluation
Background
In this article, we describe and evaluate open policy practice, a set of methods and tools for improving science-based policy making. They have been developed in the National Institute for Health and Welfare (THL, located in Finland) for more than 16 years especially to improve environmental health assessmentsa.
Science-based decision support has been a hot and evolving topic for a long time, and its importance is not diminishing any time soon. The area is complex, and all the key players – decision makers, experts, and citizens or other stakeholders – all have different views on the process, their own roles in it, and how information should be used in the process. For example, researchers often think of information as a way to find the truth, while politicians see information as one of the tools to promote political agendas ultimately based on values.[1] Therefore, any successful method should provide functionalities for each of the key groups.
In the 1970's, the focus was on scientific knowledge and an idea that political ambitions should be separated from objective assessments especially in the US. Since the 1980's, risk assessment has been a key method to assess human risks of environmental and occupational chemicals[2]. National Research Council specifically developed a process that could be used by all federal US agencies. Although it was generic in this sense, it typically focussed on a single chemical at a time and thus provided guidance for administrative permissions but less so to complex policy issues.
This shortcoming was tackled in another report that acknowledged this complexity and offered deliberation with stakeholders as a solution, in addition to scientific analysis[3]. However, despite these intentions, practical assessments have found it difficult to successfully perform deliberation on a routine basis[4]. On the contrary, citizens often complain that even if they have been formally heard during a process, they have not been listend to and their concerns have not affected decisions made.
In the early 2000's, several important books and articles were published about mass collaboration[5], wisdom of crowds[6], crowdsouring in the government[7], and co-creation[8]. A common idea of the authors was that voluntary, self-organised groups had knowledge and capabilities that could be much more effectively harnessed in the society than what was happening at the time.
These ideas were seen as potentially important for environmental health assessment in THL (at that time National Public Health Institute, KTL), and they were adopted in the work of the Centre of Excellence for Environmental Health Risk Analysis (2002-2007). A technical milestone was achieved in January 2006 when we launched our own wiki site Opasnet for environmental health assessments, inspired by the success of Wikipedia. This enabled the intertwining of both theoretical and practical work to improve assessment methods and test openness and co-creation as elementary parts of the previously closed expert work[9]. This research soon lead to a summary report about the new methods and tools developed to facilitate assessments[10].
The main ideas of our approach was to facilitate both the scientific work about policy-related facts and policy support about finding out what could and should be done and why. We identified three critical needs for development in the scientific enterprise, namely a) data sharing, b) criticism, and c) common platform for discussion, data collection, and modelling. For decision making, we identified needs to explicate better a) decision makers' values and objectives, b) connections between scientific and other relevant issues, and c) disagreements between individuals and their potential resolutions. Decision making was essentially seen as an art of balancing knowledge with values in a coherent and explicit way.
In this article, we will give the first comprehensive, peer-reviewed description about the methodology and tools of open policy practice. Case studies have been published along the way, and the key methods have been described in each article. Also, all methods and tools have been developed online and the full material have been available for interested readers since each piece was first written. However, there has not been a systematic description since the 2007 report[10], and a lot of development has taken place since.
We will also take a step back and critically evaluate the methods used during the last 16 years since the start of the Centre of Excellence.
Finally, we will discuss some of the main lessons learned and give guidance for further work to improve the use of scientific information in societal decision making.
Methods
Extended causal diagrams
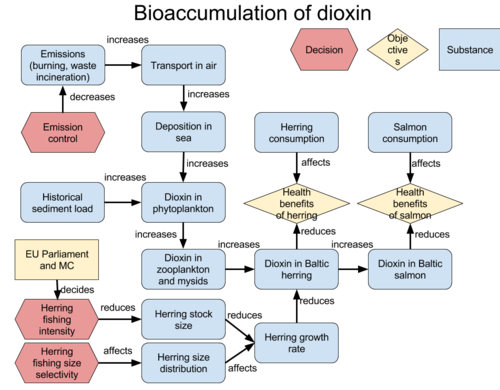
Policy issues are complex, which makes it difficult to understand, analyse, discuss and communicate them effectively. The first innovation that we implemented to tackle this difficulty was extended causal diagramb. Extended causal diagrams are based on an idea that irrespective of a decision situation at hand, core issues always include some actions based on the decision, and these actions have causal effects on some objectives. In a diagram, actions, objectives, and other issues are depicted with nodes (aka vertices), and their causal relations are depicted with arrows (aka arcs). For example, a need to reduce dioxins in human food may lead to a decision to clean up emissions from waste incineration (Figure 1.). Reduced emissions improve air quality and dioxin deposition into the Baltic Sea, which has a favourable effect on concentrations in the Baltic herring and thus human food and health, which is an ultimate objective (among others).
Causal modelling as such is an old idea, and there are various methods developed for it, both qualitative and quantitative. However, the additional ideas with extended causal diagrams were that a) they can effectively be used in communication especially with complex cases, and b) also all non-causal issues can and should be linked to the causal core in some way, if they are relevant to the decision. In other words, a participant in a policy discussion should be able to make a reasonable connection between what they are just saying and some node in an extended causal diagram developed for that policy issue.
The first implementations of extended causal diagrams were about toxicology of dioxins[12] and restoration of a closed asbestos mine area[13]c. In the early cases, the main purpose was to give structure to discussion and assessment rather than to be a backbone for quantitative models. In later implementations, such as in the composite traffic assessment[14] or BONUS GOHERR project[11], diagrams have been used in both purposes.
For more examples and the description of the current notation, see Appendix 1.
Knowledge crystals
Although an extended causal diagram provides a method to illustrate a complex decision situation, it offers little help in describing quantitative nuances within the nodes or arrows, such as functional or probabilistic relations or estimates. There are tools with such functionalities, e.g. Hugin (Hugin Expert A/S, Aalborg, Denmark) for Bayesian belief networks and Analytica® (Lumina Decision Systems Inc, Los Gatos, CA, USA) for Monte Carlo simulation. However, commercial tools are typically designed for a single desktop user rather than for open co-creation. In addition, they have limited possibilities for adding non-causal nodes and links or free-format discussions about the topics.
There was a clear need for theoretical and practical development in this area, so we combined three open source softwares into a single web-workspace named Opasnet for discussions, modelling and data storage of scientific and policy issues: Mediawiki, R statistical software, and MongoDB database. The workspace will be described in more detail in the next section and appendices 2–4. Here we will first look at how the information is structured in this system.
The second major innovation was the concept of a knowledge crystal. Its purpose is to offer a versatile information structure for a node in an extended causal diagram. It should be able to handle any topic and to systematically describe all causal and non-causal relations to other nodes, whether they are quantitative or qualitative. It should contain mathematics, discussions, illustrations, or other information as necessary. Also, it should handle both facts and values, and withstand misconceptions and fuzzy thinking as well. Its main structure should be universal and understandable for both a human and a computer. It should be manageable using scientific practices, notably criticism and openness, i.e. in a way that anyone can read and contribute to its content. And finally, it should be easy enough for an interested non-expert to find it online and to understand and use its main message.
After some experiments, we identified a few critical features a knowledge crystal should have to fulfil its objectives. First, a knowledge crystal is a web page with a permanent identifier or URL. Second, it has an explicit topic, which is described in the format of a research question. Importantly, the topic does not change over time (in practice, adjustments to the wording are allowed especially if the knowledge crystal is not yet established and used in several different assessments). This makes it possible for a user to come later to the same page and find an up-to-date version of the same topic.
Third, the purpose of a page is to give an informed answer to the question presented. The answer is expected to change as new information becomes available, and anyone is allowed to bring in new relevant information as long as certain rules of co-creation are followed. In a sense, the answer of a knowledge crystal is never final but it is always usable.
A standardised structure is especially relevant for the answer of a knowledge crystal, because it enables its direct use in assessment models or internet applications. So even though the content is updated as knowledge increases, the answer remains in the same, computer-readable format. So far, such interpretations of particular research topics have been very rare: open data contains little or no interpretation, and scientific reviews or articles are not machine-readable (at least not until artificial intelligence develops further).
Fourth, an answer is based on information, reasoning, and discussion presented on the page under the heading rationale. The purpose of rationale is to contain anything that is needed to convince a critical rational reader about the validity of the answer. It is also the place for new information and discussions that may change the answer.
Attribute | Description |
---|---|
Name | An identifier for the knowledge crystal. Each Opasnet page has two identifiers: the name of the page (e.g. Mercury concentrations in fish in Finland; for humans) and the page identifier (e.g. Op_en4004; for computers). |
Question | Gives the research question that is to be answered. It defines the scope of the knowledge crystal. When possible, the question should be defined in a way that it has relevance in many different situations, i.e. makes the page reusable. (For example, a page about mercury concentrations can be used in several assessments related to fish consumption.) |
Answer | Presents an understandable and useful answer to the question. It is the current best synthesis of all available data. Typically it has a descriptive easy-to-read summary and a detailed quantitative result published as open data. An answer may contain several competing hypotheses, if they all hold against scientific criticism. This way, it may include an accurate description of the uncertainty of the answer. |
Rationale | Contains any information that is necessary to convince a critical rational reader that the answer is credible and usable. It presents the reader the information required to derive the answer and explains how it is formed. It may have different sub-attributes depending on the page type, some examples are listed below. Rationale may also contain lengthy discussions about relevant details.
|
Other | In addition to attributes, it is practical to have clarifying subheadings on a knowledge crystal page. These include: See also, Keywords, References, Related files |
It is useful to compare a knowledge crystal to a scientific article, which is organised around a single dataset or an analysis and is expected to stay permanently unchanged after publication. Further, articles offer little room for deliberation about the interpretation or meaning of the results after a manuscript is submitted: reviewer comments are often not published, and further discussion about an article is rare and mainly occurs only if serious problems are found. Indeed, the current scientific publishing system is poor in correcting errors via deliberation[15].
In contrast, knowledge crystal is designed to support continuous discussion about the science (or values, depending on the topic) backing up conclusions, thus hopefully leading to improved understanding of the topic.
This process is similar to that in Wikipedia, but the information structure is different, as Wikipedia articles describe issues rather than answer specific questions. Another difference is that Wikipedia relies on established sources such as textbooks rather than interprets original results. There is a clear reason for this difference: knowledge crystals are about some details of a decision or an assessment, and there is no established textbook knowledge about most of the information needed.
There are different kinds of knowledge crystals for different uses. Variables contain substantive topics such as emissions of a pollutant, food consumption or other behaviour of an individual, or disease burden in a population (for examples, see Figure 1.) Assessments describe the information needs of particular decision situations and work processes designed to answer those needs. They also may describe whole models (consisting of variables) for simulating impacts of a decision. Methods describe specific procedures to organise or analyse information. The question of a method typically starts with "How to..." For a list of all knowledge crystal types used in Opasnet web-workspace, see Appendix 1.
Knowledge crystals are designed to be modular and reusable. This is important for the efficiency of the work. Variables are used in several assessments where possible, and methods are used to standardise and facilitate the work. For this reason, all software in Opasnet are widely used open source solutions. An R package OpasnetUtils was developed to contain the most important methods, functions, and information structures needed to use knowledge crystals in modelling (for details, see Appendix 2).
Opasnet web-workspace
In 2006, we set an objective to develop an assessment system that has a solid theoretial basis and coherent practices that are supported by functional tools. We assumed that the different parts need to be developed side by side so that theories are tested with practical work and assessment practices and tools are evaluated against the theoretical framework. This lead our team to simultaneously develop information structures, assessment methods, and evaluation criteria; perform assessment case studies; and build software libraries and online tools.
The first part of our web-workspace, namely a wiki, was launched in January, 2006. Its name Opasnet is a short version of Open Assessors' Network. The purpose was to test and learn co-creation among environmental health experts and also start opening the assessment process to interested stakeholders. There were several reasons to choose Mediawiki platform. First, it is the same as used by Wikipedia, so a large group of people had already tested and developed many novel practices and we could directly learn from them. Second, open code and wide use elsewhere would help our practices spread to new communities because the installation and learning costs were presumably low. Third, Mediawiki has necessary features to implement good research practices, such as talk pages to clearly separate content and discussions about the content, and automatic and full version control. And fourth, the maintenance and development of the software itself seemed to be certain for several years.
We launched several wiki instances for different projects, as participing researchers didn't want to write to a website that was visible to other projects as well. However, this caused extra maintenance burden and confusion with no real added value, so we soon started to discourage against this practice. Instead, we moved to a system with three wiki instances. English Opasnet (en.opasnet.org) contained all international projects and most scientific information. Finnish Opasnet (fi.opasnet.org) contained mostly project material for Finnish projects and pages targeted for Finnish audiences. Heande (short for Health, the Environment, and Everything) was a password-protected project wiki, which contained information that could not be published (yet) for a reason or another. We used a lot of effort to encourage researchers to write directly to open wikis, but most were hesitant to do so at the time (and many still are).
In the beginning, Opasnet was mainly used to document project content. All environmental health assessments were performed using commercial software, notably Analytica®. However, it was clear that the contents of all assessment models to the very detail should be published and opened up for public scrutiny. Although it was possible to upload model files for people to download and examine, this never actually happened in practice because any interested reader would have had to obtain, install, and learn the software first. So, it was necessary to switch to an open source modelling software that enabled online working with model code.
The statistical software R was chosen, as it was widely used, it had object-oriented approach (thus supporting modularity) and it enabled complex modelling with fairly simple code, thanks to hundreds of packages that volunteers had written and shared to enhance the functionalities of R. There was no inherent support for R in Mediawiki, so we had to write our own interface. As a result, we could write R code directly to a wiki page, save, and run it by clicking a button. The output of the code would appear as a separate web page, or embedded on the wiki page with the code. Resulting objects could also be stored to the server and fetched later by another code. This made it possible to run complex models online without installing anything on your own computer (except a web browser). It also enabled version control and archival of both the model code and the model results.
We also developed an R package OpasnetUtils (available from CRAN repository cran.r-project.org) to support knowledge crystals and decision support models. It has a special object type for knowledge crystals (called ovariable) that implements the functionalities described above. For example, the answer can be calculated based on data or functional dependencies; it "understands" its own dependencies and is able to fetch its causal parents to the model from separate Opasnet pages; a model can be adjusted afterwards by implementing one or more decision options to relevant parts of the model, and this is done on an Opasnet page with no changes to the model code; and if input values are uncertain, it automatically propagates uncertainties through the model using Monte Carlo simulation.
The modelling functionalities created a need to store data to the web-workspace. A database called Opasnet Base was created using MongoDB no-sql softwared. It has several user interfaces. A user can write a table directly on a wiki page, and the content will be uploaded to the database in a structured format. This is often used to give parameter values to variables in assessment models. A benefit is that the data is located in an intuitive place, typically under the Rationale subheading on a knowledge crystal page.
Another interface is especially used to upload large datasets (some population datasets used contain ca. 10 million rows) to Opasnet Base. It is noteworthy that each dataset must be linked to a single wiki page, which contains all the necessary descriptions and metadata about the data. All datasets are also downloadable to R for calculations irrespective of whether R is run from an Opasnet page or from user's own computer.
This data structure facilitates coherent practices of daily work with little or no extra effort needed to link datasets to relevant topics and document and archive them. Functionalities are deliberately organised in a way that all assessment-related work and research can be performed using the same tools.
Open assessment
Open assessment is a method for performing impact assessments using extended causal diagrams, knowledge crystals, and open online assessment tools. Here we use "assessment of impacts" for ex ante consideration about what will happen if a particular decision is made, and "evaluation of outcomes" for ex post consideration about what did happen after a decision was implemented. Open assessments are typically performed before a decision is made. The focus is necessarily on expert knowledge and how to organise that, although prioritisation is only possible if the objectives and valuations of the decision maker are known.
As a research topic, open assessment attempts to answer this research question: "How can scientific information and value judgements be organised for improving societal decision-making in a situation where open participation is allowed?" This question was in our minds when we developed many of the ideas presented in this article. As can be seen, openness, participation, and values are taken as given premises. In the early 2000's, this was far from common practice, although these ideas had been proposed before we started to develop practices based on them[3].
The main focus since the beginning was to think about information and information flows, rather than jurisdictions, roles, or hierarchies. So, we deliberately ignored questions like what kinds of scientific committees are needed to support relevant high-quality advice; or how expert opinions should be included in the work of e.g. a government, parliament, or municipality council. The idea was rather that if the information production process is completely open, it can include information from any committee or individual as long as the quality aspect is successfully resolved. And if all useful information related to a decision can be synthesised and made available to everyone, then any kind of decision-making body could use that information. Generic approach was chosen to be helpful irrespectice of the structure of the administration.
Of course, this does not mean that any kind of organisation or individual is equally prone or capable of using assessment information. It simply means that we considered it as a separate question. Having said that, there was also a thought that if a good assessment is able to produce some clear and unequivocal conclusion that the whole public can see and understand, it will become much harder for any decision maker to deviate from that conclusion.
Principles in open assessment
There are guidance about crowdsourced policymaking[16], and similar ideas have been utilised in open assessment. Openness, causality, knowledge crystals, and reuse are principles that have been built in the functionalities of the tools in aim to make it easy to obey them (Table 2). Drawing extended causal diagrams puts emphasis on causalities, and publishing them on open web-workspaces complies with openness automatically. Knowledge crystals promote reuse of information. Some other principles require more understanding and effort from the participants.
Intentionality is about making the values and objectives of decision makers visible and under scrutiny. There exist models for describing facts and values in a coherent dual system, and such methods should be encouraged[17]. However, this often requires extra effort, and also it may be tactically useful for a decision maker not to conceal all their values.
Criticism based on observations and rationality is a central idea in the scientific method, and therefore it is also part of open assessment. However, its implementation into the tools is difficult. For example, only in rare cases it is possible or practical to develop logical propositions that would automatically rule out a statement if another statement is found true. So, most critique is verbal or written discussion between participants and difficult to automate. Still, we have found some useful information structures for criticism.
Discussions can be organised according to pragma-dialectical argumentation rules[18], so that arguments form a hierarchical thread pointing to a main statement or statements. Attack arguments are used to invalidate arguments, and defends are used to prevent from attacks, while comments are used to clarify issues. At the moment, such hierarchical structures are built by hand based on what people say. But it has clear added value for a reader, because even a lengthy discussion can be summarised into a short statement after a resolution is found, and any thread can be individually scrutinised.
Grouping and respect are principles that aim to motivate and guide individuals to collaborate online. It has been found out that being part of an identifiable group clearly does both: people participate more actively and are more aware of what they can and are expected to do[7]. Respect includes the idea that merit based on contributions should be measured and evaluated, and respect should be given to participants based on these evaluations. Respect is needed also because systematic criticism easily affects people emotionally, even when the discussion is about substance rather than person. It is therefore important to show that all contributions and contributors are valued even when a contribution is criticised. Although these two principles have been identified as very important, they are currently only implemented in face-to-face meetings by facilitators giving direct feedback; Opasnet web-workspace does not have good functionalities for them.
Principle | Explanation |
---|---|
Intentionality | The decision maker explicates their objectives and decision options under consideration. All that is done aims to offer better understanding about impacts of the decision related to the objectives of the decision maker. Thus, the participation of the decision maker in the decision support process is crucial. |
Causality | The focus is on understanding and describing the causal relations between the decision options and the intended outcomes. The aim is to predict what impacts will occur if a particular decision option is chosen. |
Criticism | All information presented can be criticised based on relevance and accordance to observations. The aim is to reject ideas, hypotheses - and ultimately decision options - that do not hold against criticism. Criticism has a central role in the scientific method, and here we apply it in practical situations, because rejecting poor statements is much easier and more efficient than trying to prove statements true. |
Knowledge crystals | All information is shared using a systematic structure and a common workspace where all participants can work. Knowledge crystals are used for this. The structure of an assessment and its data is based on substance rather than on persons, organisations, or processes (e.g. data is not hidden in closed institute repositories; see also causality). Objectives determine the information needs, which are then used to define research questions to be answered in the assessment. The assessment work is collaboration aiming to answer these questions in a way that holds against critique. |
Openness | All work and all information is openly available to anyone interested for reading and contributing all the time. If there are exceptions, these must be publicly justified. Openness is crucial because a priori it is impossible to know who may have important information or value judgements about the topic. |
Reuse | All information is produced in a format that can easily be used for other purposes by other people. Open data principles are used when possible[19]. For example, some formats such as PDF files are not easily reusable. Reuse is facilitated by knowledge crystals and openness. |
Grouping | Facilitation methods are used to promote the participants' feeling of being an important member of a group that has a meaningful purpose. |
Respect | Contributions are systematically documented and their merit evaluated so that each participant receives the respect they deserve based on their contributions. |
Properties of good assessment
There is a need to evaluate the assessment work before, during, and after it is done[20]. First we take a brief look at what makes a good assessment and what criteria could be used (see Table 3)[21].
Quality of content refers to the output of an assessment, typically a report, model or summary presentation. Its quality is obviously an important property. If the facts are plain wrong, it is more likely to misguide than lead to good decisions. But it is more than that. Informativeness and calibration describe how large the remaining uncertainties are and how close the answers probably are to the truth (compared with some golden standard). In some statistical texts, similar concepts have been called precision and accuracy, respectively, although with assessments they should be understood in a flexible rather than strictly statistical sense.[22] Coherence means that the answers given are to the questions asked. Although not always easy to evaluate, coherence is an important property to keep in mind because lack of it is common: politician's are experts in answering other questions than asked, and researchers tend to do the same if the research funding is not sufficient to answer the actual, hard question.
Applicability is a large evaluation area. It looks at properties that affect how well the assessment work can and will be applied to support decisions. It is independent of the quality of content, i.e. despite high quality, an assessment may have very poor applicability. The opposite may also be true, as sometimes faulty assessments are actively used to promote policies. However, usability typically decreases rapidly if the target audience evaluates an assessment to be of poor quality.
As coherence evaluates whether the assessment question was answered, relevance asks whether a good question was asked in the first place. Understanding what the right question actually is is surprisingly hard, and often its identification requires lots of discussion and deliberation between different groups, including decision makers and experts. Typically there is always too little time available for such discussions, and online forums may potentially help in this.
Availability is more technical property and describes how easily a user can find the information when needed. A typical problem is that a potential user does not know that information exists even if it could be easily accessed. Usability may differ from user to user, depending on e.g. background knowledge, interest, or time available to learn the content.
Acceptability is a very complex issue and most easily detectable when it fails. A common situation is that stakeholders feel that they have not been properly heard and therefore any output from an assessment process is perceived faulty. Also doubts about the credibility of the assessor fall into this category.
Efficiency evaluates resource use when performing an assessment. Money and time are two common measures for this. Often it is most useful to evaluate efficiency before an assessment is started. Is it realistic to produce new important information given the resources and schedule available? If more (less) resources were available, what added (lost) value would occur? Another aspect in efficiency is that if assessments are done openly, reuse of information becomes easier and the marginal cost and time of a new assessment decrease.
All properties of good assessment, not just efficiency, are meant to guide planning, execution, and evaluation of the whole assessment work. If they are kept in mind always, they can improve daily work.
Category | Property | Description | Question |
---|---|---|---|
Quality of content | Informativeness | Specificity of information, e.g. tightness of spread for a distribution. | How many possible worlds does the answer rule out? How few possible interpretations are there for the answer? |
Calibration | Exactness or correctness of information. In practice often in comparison to some other estimate or a golden standard. | How close is the answer to reality or real value? | |
Coherence | Correspondence between questions and answers. Also between sets of questions and answers. | How completely does the answer address the assessment question? Is everything addressed? Is something unnecessary? | |
Applicability | Relevance | Correspondence between output and its intended use. | How well does the information provided by the assessment serve the needs of the users? Is the assessment question good? |
Availability | Accessibility of the output to users in terms of e.g. time, location, extent of information, extent of users. | Is the information provided by the assessment available when, where and to whom is needed? | |
Usability | Potential of the information in the output to trigger understanding in its users about what it describes. | Can the users perceive and internalise the information provided by the assessment? Does users' understanding increase about the assessed issue? | |
Acceptability | Potential of the output being accepted by its users. Fundamentally a matter of its making and delivery, not its information content. | Is the assessment result (output), and the way it is obtained and delivered for use, perceived as acceptable by the users? | |
Efficiency | Intra-assessment efficiency | Resource expenditure of producing the assessment output. | How much effort is spent in the making of an assessment? |
Inter-assessment efficiency | Resource expenditure of producing assessment outputs in a series of assessments. | If another (somewhat similar) assessment was made, how much (less) effort would be needed? |
Open policy practice

During Intarese project (2005-2011), it became more and more clear to us based on literature and own practical experience that assessments themselves were not enough to convey the information to decision processes. The scientific and political realms are based on different premises and objectives, and we identified a need to evaluate when the information does not flow well and what are typical problems with it. So, new theoretical work was done on decision processes, roles of assessments and information in them, and guidance for participants (Figure 2).
Open policy practice is a method to support societal decision making in an open society, and it is the overarching concept covering all methods, tools, practices, and terms presented in this article[23]. One part of open policy practice is open assessment, which focuses on producing relevant information for decision making. Open policy practice is a larger concept and it is especially focused on promoting the use of information in the decision making process. It gives practical guidance for the whole decision process from initiation to decision support to actual decision making to implementation and finally to evaluation of outcomes. Our aim was that it is applicable to all kinds of societal decision situations in any administrative area or discipline.
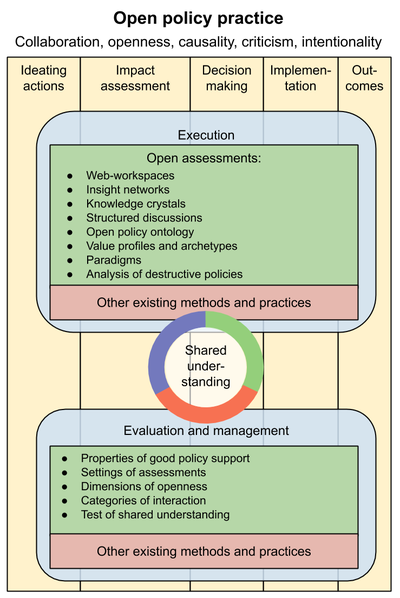
Open policy practice is divided into four main parts, which are brefly described here (see also Figure 3).
- Shared understanding (a structured documentation of different facts, values, and disagreements related to a decision situation) is the main objective of open policy practice. It is a product that guides the decision and also is basis for evaluation of outcomes. For more details, see the next section.
- The execution of decision support. It is mostly about collecting, organising and synthesising scientific knowledge and values in order to inform the decision maker to help them reach their objectives. In practice, most of open assessment work happens in this part. It also contains the execution of the decision process itself and the integration of these two processes.
- Evaluation and management of the work (of decision support and decision making). It focusses on looking at what is being done, whether the work produces the intended knowledge and helps to reach the objectives, and what needs to be changed. It continues all the way through the decision process (before, during, and after the actual execution).
- Co-creation skills and facilitation (sometimes known as interactional expertise). Information has to be collected, organised, and synthesised; and facilitators need to motivate and help people to share their information. This requires specific skills and work that are typically available neither among experts nor decision makers. It also contains specific practices and methods, such as motivating participation, facilitating discussions, clarifying and organising argumentation, moderating contents, using probabilities and expert judgement for describing uncertainties, or developing extended causal diagrams or quantitative models.
When we thought about the decision making process from planning to implementation and evaluation, we realised that the properties of good assessment (see previous section) can be easily adjusted to this wider context. Target object in this wider context is not an assessment report but a shared understanding about the decision option to be chosen and implemented. The evaluation criteria are valid in both contexts. The adjusted list of criteria is presented on Opasnet page Open policy practice. We have also developed other evaluation criteria for important aspects of open policy practice; the most important are described below.
Settings of assessments
All too often a decision making process or an assessment is launched without clear understanding, what should be done and why. An assessment may even be launched hoping that it will somehow reveal what the objectives or other important things are. Settings of assessments are a part of evaluation and management (Table 4). They try to help in explicating these things so that useful decision support can be provided[24]. Also the subattributes of an assessment scope help in this:
- Question: What is the actual research question?
- Boundaries: What are the limits within which the question is to be answered?
- Decisions and scenarios: What decisions and options will be assessed and what scenarios will be considered?
- Timing: What is the schedule of the assessment work?
- Participants: Who are the people who will or should contribute to the assessment?
- Users and intended use: Who is going to use the final assessment report and for what purpose?
Attribute | Guiding questions | Example categories |
---|---|---|
Impacts |
|
Environment, health, cost, equity |
Causes |
|
Production, consumption, transport, heating, power production, everyday life |
Problem owner |
|
Policy maker, industry, business, expert, consumer, public |
Target users |
|
Policy maker, industry, business, expert, consumer, public |
Interaction |
|
Isolated, informing, participatory, joint, shared |
Dimensions of openness
In open assessment, the method itself is designed to facilitate openness in all its dimensions. The dimensions of openness help to identify if and how the work deviates from the ideal of openness, so that the work can be improved in this respect (Table 5)[25]. However, it is important to notice that currently there is a large fraction of decision makers and experts who are not comfortable with open practices and openness as a key principle, and they would like to have a closed process. Dimensions of openness do not give direct tools to convince them. But it identifies issues where openness makes a difference and increases understanding about why there is a difference. This hopefully also leads to wider acceptance of openness.
Dimension | Description |
---|---|
Scope of participation | Who are allowed to participate in the process? |
Access to information | What information about the issue is made available to participants? |
Timing of openness | When are participants invited or allowed to participate? |
Scope of contribution | To which aspects of the issue are participants invited or allowed to contribute? |
Impact of contribution | How much are participant contributions allowed to have influence on the outcomes? In other words, how much weight is given to participant contributions? |
Openness can also be examined based on how intensive it is and what kind of collaboration is aimed at between decision makers, experts, and stakeholders. Different approaches are described in Table 6. During the last decades, the trend has been from isolated to more open approaches, but all categories are still in use.
Category | Explanation |
---|---|
Isolated | Assessment and use of assessment results are strictly separated. Results are provided to intended use, but users and stakeholders shall not interfere with making of the assessment. |
Informing | Assessments are designed and conducted according to specified needs of intended use. Users and limited groups of stakeholders may have a minor role in providing information to assessment, but mainly serve as recipients of assessment results. |
Participatory | Broader inclusion of participants is emphasized. Participation is, however, treated as an add-on alongside the actual processes of assessment and/or use of assessment results. |
Joint | Involvement of and exchange of summary-level information among multiple actors in scoping, management, communication and follow-up of assessment. On the level of assessment practice, actions by different actors in different roles (assessor, manager, stakeholder) remain separate. |
Shared | Different actors involved in assessment retain their roles and responsibilities, but engage in open collaboration upon determining assessment questions to address and finding answers to them as well as implementing them in practice. |

Shared understanding is a situation where all participants' views have been described and documented well enough so that people can know what facts, opinions, reasonings, and values exist about a particular topic; and what agreements and disagreements exist and why.
Shared understanding is always about a particular topic and produced by a particular group of participants. With another group it could be different, but with increasing number of participants, it should approach shared understanding of the whole society. Each participant should agree that the written description correctly contains their own thinking about the topic. Participants should even be able to correctly explain what other thoughts there are and how they differentiate from their own. Ideally any participant can learn, understand, and explain any thought represented in the group. Importantly, there is no need to agree on things, just to agree on what the disagreements are about. Therefore, shared understanding is not the same as consensus or agreement.
Shared understanding has potentially several purposes that all aim to improve the quality of societal decisions. It helps people understand complex policy issues. It helps people see their own thoughts from a wider perspective and thus increase acceptance of decisions. It improves trust in decision makers; but it may also deteriorate trust if the actions of a decision maker are not understandable based on shared understanding. It dissects each difficult detail into separate discussions and then collects resolutions into an overview; this directs the time resources of participants efficiently. It improves awareness of new ideas. It releases the full potential of the public to prepare, inform, and make decisions. How well these purposes have been fulfilled in practice in assessments will be discussed in Results.
Test of shared understanding can be used to evaluate how well shared understanding has been achieved. In a successful case, all participants of the decision process will give positive answers to the questions in Table 7. In a way, shared understanding is a metric for evaluating how well decision makers have embraced the knowledge base of the decision situation.
Question | Who is asked? |
---|---|
Is all relevant and important information described? | All participants of the decision processes. |
Are all relevant and important value judgements described? (Those of all participants, not just decision makers.) | |
Are the decision maker's decision criteria described? | |
Is the decision maker's rationale from the criteria to the decision described? |
Shared understanding may be viewed from two different levels of ambition. On an easier level, shared understanding is taken as general guidance and an attitude towards other people's opinions. Main points and disagreements are summarised in writing, so that an outsider is able to understand the overall picture.
On an ambitious level, the idea of documenting all opinions and their reasonings is taken literally. Participants' views are actively elicited and tested to see whether a facilitator is able to reproduce their thought processes. The objective here is to document the thinking in such a detailed way that a participant's response can be anticipated from the description they have given. The purpose is to enable effective participation via documentation, without a need to be present in any particular hearing or other meeting.
Written documentation with an available and usable structure is crucial in spreading shared understanding among those who were not involved in discussions. Online tools such as wikis are needed especially in complex topics, among large groups, or if the ambition level is high.
Good assessment models are able to quickly and easily incorporate new information or scenarios, so that they can be run again and again and learn from these changes. In a similar way, a comprehensive shared understanding can incorporate new information from the participants. A user should be able to quickly update the knowledge base, change the point of view, or reanalysise how the situation would look like with alternative valuations. Such level of sophistication necessarily requires a few concepts that have not yet been described.
Value profile
Value profile is a list of values, preferences, and choices made and documented by a participant. Voting advice applications produce a kind of value profiles. The candidates answer questions about their values, worldviews, or decisions they would do if elected. The public can then answer the same questions and analyse which candidates share their values. Nowadays, such applications are routinely developed by all major media houses for every national election in Finland. However, these tools are not used to collect value profiles from the public between elections although such information could be used in decision support. Value profiles are mydata, i.e. data about which an individual themself may decide who is allowed to see and use it. This requires trusted and secure information systems.
Archetype
Archetypes are collections of values that are shared by a group, so that an individual can explicate their own values by simply saying that they are equal to those of an archetype. For example, political parties can develop archetypes to describe their political agendas and programs. Of course, individuals may support an archetype in most issues but diverge in some and document those separately. In this way, people may easily document value profiles in a shared understanding system. Practical tools for this do not yet exist, so little is known about how practical and accepted they would be.
Archetypes aim to save effort in gathering value data from the public, as not everyone needs to answer all possible questions, when archetypes are used. It also increases security when there is no need to handle individual people's answers but open aggregated value data instead.
Paradigm
Paradigms are collections of rules to make inferences from data in the system. For example, scientific paradigm has rules about criticism and priority over hypotheses that are in accordance with data and rational reasoning. However, participants are free to develop paradigms with any rules of their choosing, as long as they can be documented and operationalised within the system. For example, a paradigm may state that in a conflict, priority is given to the opinion presented in a holy book. And in a hypothetical case where there is disagreement about what the holy book says, separate sub-paradigms may be developed for each interpretation. Mixture paradigms are also allowed. For example, a political party may follow the scientific paradigm in most cases but when economic assessments are ambiguous, the party will choose an interpretation that emphasises the importance of an economically active state (or alternatively market approach with a passive state).
This work is based on an idea that although the number of possible values and reasoning rules is very large, most of people's thinking can be covered with a fairly small amount of archetypes and paradigms. As a comparison, there are usually from two to a dozen parties in a democratic country rather than hundreds. In other words, it is putatively an efficient system. If this is true, resources can be used to get the critical issues precise and informative, and then apply these information objects in numerous practical cases.
It should be emphasised that although we have used wording such as "make inferences in the system", this approach does not need to rely on artificial intelligence. Rather, numerous contributors who co-create content and make inferences based on the rules described in paradigms, are the "system" we refer to. Parts of the work described here may be automated in the future, but the current system is mostly based on human work.
By now it seems clear that information in a description of shared understanding is very complex and often very large. So, a new research question emerges: how can all this information be written down and organised in such a way that it can easily be used and searched by both a human and a computer? A descriptive book would be too long for busy decision makers and unintelligible for computers. An encyclopedia would miss relevant links between items. A computer model would be a black box for humans.
Ontology
We suggest that in addition to all other information structures presented above, there is a need for an ontology that would acknowledge these information structures, special needs of decision situations, informal nature of public discussions, and computational needs of quantitative models. Such an ontology or vocabulary would go a long way in integrating readability for humans and computers.
World Wide Web Consortium has developed the concepts of open linked data and resource description framework[26]. These have been used as the main starting points for ontology development. This work is far from final, but we will present the current version.
Ontologies are based on vocabularies with specified terms and meanings. Also the relations of terms are explicit. Resource description framework is based on the idea of triples, which have three parts: subject, predicate, and object. These can be thought as sentences: an item (subject) is related to (predicate) another item or value (object), thus forming a claim. Claims can further be specified using qualifiers and backed up by references. Such a block of information is called a statement. Extended causal diagrams can be described automatically as triples, and vice versa. Triple databases enable wide, decentralised linking of various sources and information. There is an open source solution for linking such a database directly to wiki platform. This software is called Wikibase.
The current version of the open policy practice ontology focusses on describing all information objects and terms described above, and making sure that there is a relevant item or relation to every piece of information that is described in an extended causal diagram, open assessment, or shared understanding. However, the strategy here is not to describe all possible information with such a structure, but only the critical part related to the decision situation.
For example, Figure 1 shows that the EU Parliament and Ministry Council have a role in fishing regulation, but this graphical representation of the triple does not attempt to tell anything else about these large and complex organisations, except that they have a role in the case and they make a decision about herring fishing intensity. Also, in Figure A1-1 in appendix 1 there are two scientific articles listed. The content of these articles is not described, because already with little effort (connecting an article identifier and a topic) the availability and usability of the article increases a lot. In contrast, a further step of structuring the article content into the causal diagram would take much more work and give less added value, unless someone identifies an important new statement in the article, making the effort worth it.
Thus, the aim is that any publication or another piece of new information would fairly easily and with little work find a proper place within this comples network of triples. The internal structure of the information within each information piece is only documented as necessary.
For a full description of the current vocabulary in the ontology, see Appendix 1.
Results and evaluation
Topic | Assessment | Year | Project |
---|---|---|---|
Vaccine effectiveness and safety | Assessment of the health impacts of H1N1 vaccination[27] | 2011 | In-house, collaboration with Decision Analysis and Risk Management course |
Tendering process for pneumococcal conjugate vaccine[28] | 2014 | In-house, collaboration with the National Vaccination Expert Group | |
Energy production, air pollution and climate change | Helsinki energy decision[29] | 2015 | In-house, collaboration with city of Helsinki |
Climate change policies and health in Kuopio[30] | 2014 | Urgenche, collaboration with city of Kuopio | |
Climate change policies in Basel[31] | 2015 | Urgenche, collaboration with city of Basel | |
Availability of raw material for biodiesel production[21] | 2012 | Jatropha, collaboration with Neste Oil | |
Health impacts of small scale wood burning[32] | 2011 | Bioher, Claih | |
Health, climate, and economic effects of traffic | Gasbus - health impacts of Helsinki bus traffic[33] | 2004 | Collaboration with Helsinki Metropolitan Area |
Cost-benefit assessment on composite traffic in Helsinki[14] | 2005 | In-house | |
Risks and benefits of fish eating | Benefit-risk assessment of Baltic herring in Finland[34] | 2015 | Collaboration with Finnish Food Safety Authority |
Benefit-risk assessment of methyl mercury and omega-3 fatty acids in fish[35] | 2009 | Beneris | |
Benefit-risk assessment of fish consumption for Beneris[36] | 2008 | Beneris | |
Benefit-risk assessment on farmed salmon[37] | 2004 | In-house | |
Benefit-risk assessment of Baltic herring and salmon intake[11] | 2018 | BONUS GOHERR | |
Dioxins, fine particles | TCDD: A challenge to mechanistic toxicology[12] | 1999 | EC ENV4-CT96-0336 |
Comparative risk assessment of dioxin and fine particles[38] | 2007 | Beneris | |
Plant-based food supplements | Compound intake estimator[39] | 2014 | Plantlibra |
Health and ecological risks of mining | Paakkila asbestos mine[13] | 1999 | In-house |
Model for site-specific health and ecological assessments in mines[40] | 2013 | Minera | |
Risks of water from mine areas [41] | 2018 | Kaveri | |
Drinking water safety | Water guide[42] | 2013 | Conpat |
Organisational assessments | Analysis and discussion about research strategies or organisational changes within THL | 2017 | In-house |
Transport and communication strategy in digital Finland[43] | 2014 | Collaboration with the Ministry of Transport and Communications of Finland | |
Information use in government decision support | Case studies: Assessment of immigrants' added value; Real-time co-editing, Fact-checking, Information design[44] | 2016 | Yhtäköyttä, collaboration with Prime Miniter's Office |
The methods described above have been used in several research projects (see the funding declaration) and health assessments (some mentioned in Table 8) since 2004. They have also been taught on international Kuopio Risk Assessment Workshops for doctoral students in 2007, 2008, and 2009 and on a Master's course Decision Analysis and Risk Management (6 credit points), organised by the University of Eastern Finland (previously University of Kuopio) in 2011, 2013, 2015, and 2017.
As methods and tools were developed side by side with practical assessment work, there is extensive experience about some parts of the method. Some newer parts (e.g. value profiles) are merely ideas with no practical implementation yet. This evaluation is based on the experience accumulated during the scientific, expert, and teaching work. We will follow the properties of good assessment and apply them to the method itself.
Quality of content
Open policy practice does not restrict the use of any previous methods that are necessary for successful decision support. Therefore, it is safe to say that the quality of the produced information is at least the same as with some other method. We have noticed in numerous cases that the structures offered for e.g. assessments or knowledge crystals help in organising and understanding the content.
Criticism is emphasised in open policy practice as an integral part of the scientific method. Giving critique is made as easy as possible. Surprisingly, we still see fairly little of it in practical assessments. There seem to be several reasons: experts have little time to actually read other people's assessment and give detailed comments; people are reluctant to interfere with other people's work even within a joint project, so they rather keep to a strict division of tasks; there are no rewards or incentives for giving critique; and when an assessment spans several web pages, it is not clear where and how to contribute.
We have seen lack of criticism even in vaccine-related assessments that are potentially emotive. With active facilitation we were able to get comments and critique from both drug industry and vaccine citizen organisations, and they were all very matter-of-fact. This was interesting, as the same topics cause outrage in social media, but we did not see that on structured assessments. However, one of the most common objections and fears against open assessment is that outside contributions will be ill-informed and malevolent. They have never been in our assessments.
Lack of contributions limits the amount of new views and ideas that potentially could be identified with open participation. However, even if we do not see all potential of criticism, we don't think that the lack of open critique would hamper the quality of assessments, because all the common practices of e.g. experts' source-checking are still in place. Actually, the current practices in research and assessment are even worse in respect of open criticism: it rarely happens. Pre-publishing peer review is almost the only time when scientific work is criticised by people outside a research group, and those are typically not open. A minute fraction of published works are criticised openly in journals; a poor work is simply left alone and forgotten.
However, there are some active platforms for scientific discussion, such as pre-publishing physics forum ArXiv.org, and similar platforms are emerging on other disciplines. Such practice suits to continually updating knowledge crystals much better than what is typically done in most research areas. Open discussion is increasing, and knowledge crystals are one way to facilitate this positive trend.
Relevance
A major issue with relevance is that the communication between decision makers and experts is not optimal, and therefore experts don't have good knowledge about what questions need answers and how the information should be provided[1]. Also, decision makers prefer information that supports views that have already been selected on political grounds.
In open policy practice, the questions and structures aim to explicate relevant questions, so that experts get a clear picture what information is expected and needed. They also focus discussions and assessment work to questions that have been identified as relevant. On the other hand, shared understanding and other explicit documents of relevant information make it harder for a decision maker to pick only favourable pieces of information, at least if there is political pressure. The pneumococcal vaccine assessment (see Table 8) had clear political pressure, and it would have been difficult for the decision maker to deviate from the conclusions of the assessment. However, typically assessments are taken as just one piece of information rather than a normative guidance; this clearly depends on political culture, how strong the commitment to evidence-based decision making is, and how well an assessment succeeds in incorporating all relevant views.
Shared understanding and detailed assessments challenge participants to clarify what they mean and what is found relevant. For example, the composite traffic and biodiesel assessments were already quite developed, when discrepancies between objectives, data, and draft conclusions forced rethinking about the purposes of the assessments. In both cases, the main research questions were adjusted, and more relevant assessments and conclusions were produced. Political questions and arguments are also expected to clarify in a similar way when more of it is incorporated into systematic scrutiny.
Availability
A typical problem with availability is that a piece of information is designed for a specific user group and made available in a place targeted to that group. In contrast, open policy practice is designed with an idea that anyone can be a reader or contributor in a user group, so by default everything is made openly available on the Internet. To our experience, such approach works well in practice as long as there are seamless links to also repositories for non-publishable data and contributors know the open source tools such as wiki and R. Such openness is, however, a major perceived problem to many experts; this issue is discussed more under acceptability.
Another problem is that even if users find an assessment page, they are unsure about its status and whether some information is missing. This is because many pages are work in progress and not finalised for end users. We have tried to clarify this by adding status declarations on the tops of pages. Declaring drafts as drafts has also helped experts who are uncomfortable to show their own work before it is fully complete.
The information structure using knowledge crystals and one topic per page has proven a good one. Usually it is easy to find the page of interest even if there is only a vague idea of its name or specific content. Also, it is mostly straightforward to see which information belongs to which page.
There are some comments about not being able to find pages in Opasnet, but to our experience these problems are surpassed by benefits for people being able to easily find detailed material with e.g. search engines without prior knowledge about Opasnet. The material seems to be fairly well received, as there were 52000 visits to and 90000 pageviews of the Finnish and English Opasnet websites in 2017. The most interesting topics seemed to be ecological and health impacts of mining and drinking water safety. Also the pneumococcus vaccine assessment, Helsinki energy decision, and transport and communication strategy in digital Finland were popular pages when they were prepared.
Lack of participation among decision makers, stakeholders, and experts outside the assessment team is a constant problem in making existing information available. Assessments are still seen as a separate part from the decision process, and the idea that scientific assessments could contain value judgements from the public is unprecedented. The closest resemblance are environmental impact assessments, where the law requires public hearings, but many people are sceptic about the influence of comments given. Also Wikipedia has noticed that only a few percent of readers ever contribute, and the number of active contributors is even lower[45].
Experts are a special group of interest, as they possess vast amounts of relevant information that is not readily available to decision makers. Yet, we have found that experts are not easily motivated to policy support work.
This emphasises the need for facilitation and active invitations for people to express their views. Importantly, direct online participation is not an objective as such but one way among others to collect views and ideas. It is also more important to cover all major ideas than to represent every person individually. In any case, there is a clear need to inform people about new possibilities for participation in societal decisions. We have found it very useful to simply provide links to ongoing projects to many different kinds of user groups across and outside organisations.
Open policy practice was designed so that it could incorporate any relevant information from experts and stakeholders. In practice, it is not used for that purpose, because it does not have an established role in decision process. For many, non-public lobbying, demonstrations and even spreading faulty information are more effective ways of influencing the outcome of a decision. All these methods deviate from the ideal of evidence-based policy, and therefore further studies are needed specifically on how this information is better incorporated into and made available via shared understanding and whether that improves decision processes. If shared understanding is able to offer acceptable solutions to disagreeing parties, it reduces the need to use political force, but so far we have too little experience on that to make conclusions. We also don't yet know whether quiet people or marginal group have better visibility with such a web-workspace, but in theory, their capabilities are better.
It is also critical that the archival process is supported by the workspace. In Opasnet, it requires little extra work, as the information is produced in a proper format for archiving, backups are produced automatically, and it is easy to produce a snapshot of a final assessment. There is no need to copy information from one repository to another, but it is also easy to store final assessments in external open data repositories.
Usability
⇤--#: . Boundary object lisättävä. Myös viite.
Myös maininta erilaisista työkaluista, joita jo nyt on tarjolla (Appendix 4.) --Jouni (talk) 14:50, 24 January 2018 (UTC) (type: truth; paradigms: science: attack)
We have found it very useful to structure pages so that it starts with a summary, then describes the research question and gives a more detailed answer, and finally provides a user with relevant and increasingly detailed information in the rationale. On the other hand, some people have found this structure confusing as they don't expect to see all the details of an assessment. This emphasises the need to publish easy summaries in other places as well, e.g. in blogs, newsletters, or policy briefs.
A strength of shared understanding is in its capability of clarifying complex issues and eliciting implicit values and reasonings. It facilitates rational discussion about a decision, and it can also be used for creating policital pressure against options that are not well substantiated. For example, the shared understanding about the research strategy of THL (see Table 8.) was produced mostly based on critical discussions rather than scientific analysis. The process was well received and many participants found the discussions and syntheses illuminating.
Shared understanding can incorporate complex ideas from detailed discussions and also quantitative data from surveys. Often many different information sources and analyses are needed to produce a full picture. Indeed, open policy practice method was developed using many approaches simultaneously: information structures, online tools, and testing with actual cases and different pracices. This seemed to be a viable strategy. However, even more emphasis should have been put to usability and user friendliness of tools, and building user community.
To our experience, the slow development of the user community is partly but not mainly due to non-optimal usability of tools: both Mediawiki and especially R require some learning before they can be effectively used. All students in the courses have been able to learn and effectively operate in Opasnet, so the learning challenge can be overcome if users are motivated. A bigger problem has been to motivate people to change their practices about how to perform or participate in assessments.
Acceptability
A central theme related to acceptability is openness. Although it is a guiding principle in science, it is actually in conflict with many current practices. For example, it is common to hide expert work until it has been finalised and published, preferably in a peer-reviewed journal. Therefore, a demand to work openly and describe all reasoning and data already from the beginning is often seen as an unreasonable requirement, and it is a major reason for lack of participation. This observation has raised two opposite conclusions: either that openness should be promoted actively in all research and expert work, including decision support; or that openness as an objective is unnecessary and hinders expert work.
We have heard several objections against openness. People are concerned that expertise is not given proper weight, if open participation is allowed. People fear that strong lobbying groups hijack the process. People fear that self-organised groups produce low-quality information or even malevolent dis-information. Experts often demand the final say as the ultimate quality criteria, rather than trusting that data, reasoning, and criticism would do a better job. In brief, experts commonly think that it is simply easier and more efficient to produce high-quality information in closed groups.
An interesting example of this is the website Integrated Environmental Health Impact Assessment System (IEHIAS) that was created by two EU-funded projects, Intarese and Heimtsa. During the projects there was discussion about whether the website should be open (and integrated with Opasnet) or closed (and run separately) when it was built. Clear majority of researchers wanted to work in a closed system and especially retain control over their pages until they were finalised. But at the end of the projects, all content was unanimously published with an open license, and a few years later when the maintenance funding ended, all content was moved from IEHIAS website to Opasnet. In hindsight, developing two different websites with two different platforms and then finally merging them took a lot of extra resources and gave little added value.
The openness of scientific publishing is increasing and many resarch funders demand publishing of data, so this megatrend in the scientific society is changing common practices. It has already been widely acknowledged that the current mainstream of proprietary (as contrast to open access) scientific publishing is a hindrance to spreading ideas and ultimately science. In addition, Wikipedia has shown that self-organised groups can indeed produce high-quality content[46]. Our own experience is the same, and we have not seen hijacking, malevolent behaviour or low-quality junk contributions. We have, however, seen some robots producing unrelated advertisement material on Opasnet pages, but that is easy to identify and remove, and it has not become a problem.
All opinions are critically evaluated in open policy practice, and some of them will be found unsubstantiated. A decision maker is more likely to ignore them than without identification of their problems. However, shared understanding does not contain an idea that proponents of unsubstantiated thoughts should be forced or pressured to reject them, it merely points out these discrepancies and thus nudges participants away from them. It also aims to inform the public so that they can put political pressure against poor ideas. Indeed, one of the ideas behind the method is that it should be good in identifying poor ideas rather than having power to distinguish the best decision option from other good ones.
Sometimes people tend to stick to their original ideas. This is seen in politics, where a key purpose of information is to justify existing opinions rather than adjust them by learning[1]; and as acquiescence, i.e. situations where people know that their choice is irrational but they choose it anyway[47]. Shared understanding may help in this, as the content of an opinion and the reasons to reject it become more explicit and external, and thus the opinion may be more easily affected by the person themself or by others. Research should be performed on this particular topic.
Shared understanding has been a well accepted idea among decision makers in Finland. This was observed in collaboration with Prime Minister's Office of Finland, when they soon adopted the light version of the term and started to use it in their own discussions and publications[48][49]. Sometimes it is better to aim to understanding rather than consensus, and this idea was well accepted.
One of the criteria for development of open policy practice was that it should be versatile and usable for any expert or policy work. However, the implementation of the method can start gradually, like Prime Minister's Office started to use useful concepts and tested online editing but were reluctant to deeply commit to open assessments. Also, data visualisations showed immediate utility and were seen as an important way to communicate expert knowledge. In contrast, the full open policy practice method contains many parts that are clearly against the current practices and therefore need thorough testing and evaluation before they can be widely accepted and adopted. For example, the discussion about what parts of a law making process could be opened to the public has just started. The current default is that little is opened except some hearings that are required by law.
As mentioned before, accumulation of scientific merit is a key motivation, and established processes, journals, and scientific committees fulfil this purpose. To maximise the societal impact, an expert should possibly write to Wikipedia rather than a scientific specialist journal. However, such activity gives little merit. Indeed, only 7 % of people contributing to Wikipedia do it for professional reasons[50]. Open assessments seem to suffer a similar problem as non-established scientific endeavour.
Because presenting also controversial and unpopular ideas is a prerequisite for a complete shared understanding, it is very important that such activity is encouraged and respected. A community producing shared understanding should cherish such attitude and promote contributions even if they are incompatible with scientific or another paradigm. This is challenging to both someone presenting such opinions and someone else personally against the presented opinion. The situation requires that all parties have faith in the process and its capability to produce fair conclusions. Therefore, acceptability of open, editable pages and contributions to such pages should be promoted and motivated as a part of method development.
Efficiency
Resources needed to do a single assessment online are not that different from doing it offline. R is a powerful tool for modelling and code can be shared with those who understand it. A spreadsheet model would be readable by a larger group, but modelling, co-creation, and/or archiving functionalities are far behind from R. Emphasis must be put on documentation to ensure availability and usability of assessments, if the model code itself is not understandable to end users.
We have put effort in developing reusable modules and data sources for environmental modelling. The work has been successful in producing building blocks that have been used in several assessments about e.g. fish consumption and thus reduced the marginal costs of a new assessment about a related topic (see Table 8). However, the use of these modelling blocks has not been large outside THL, so there is much more potential for inter-assessment efficiency. Again, the main challenge is about building a community for assessments and decision support and also include people who can model.
A major issue in spreading practices to new users is that there is a lot to learn before these tools can be used. Even experts with substantive knowledge need time to familiarise with the modelling language used unless they know it already. This has lead to a situation where the lack of common modelling language hampers cooperation of experts on a detailed level. The most common solution that we have seen is strict division of tasks, specified data interfaces, and sequential work. In practice, one group produces data about e.g. emissions of a pollutant, another group takes that data and calculates exposures, and a third group estimates health impacts after the first two steps are finalised. Detailed understanding of and contributions to other groups' work remain low. On the other hand, most researchers are happy in their own niche and don't expect that other experts could or should learn the details of their work. Consequently, the need for shared tools is often considered low.
Due to these reasons, facilitation is necessary to increase understanding across disciplines and reuse of tools among experts. It is also necessary to improve the readability of the content, help non-experts to understand complex issues, and keep discussion on focus. All this increases the usability of open assessments and makes the next assessment easier.
So far, there is no data about how much archetypes and paradigms are able to summarise values and reasoning rules and thus make policy discussions more precise and efficient. The popularity of voting advice applications demonstrates that there is a societal need for value analysis and aggregation. Our hypothesis is that several archetypes that describe common and important values in the population will increase in popularity and form kind of virtual parties that represent the population well with key values, while less important values will not raise in a similar way.
Also, we hypothesise that only a few major paradigms will emerge, and those are ones whose applicability is wide and independent of the discipline. Scientific paradigm is expected to be one of them, and it will be interesting to see what else emerges. People commonly reason against some unintuitive rules of the scientific method (e.g. they try to prove a hypothesis right rather than wrong) but it is not clear whether this will cause a need to develop a paradigm for an alternative approach. It is even not clear whether people are willing to accept the idea that there could be different, competing rules for reasoning in a single assessment or decision support process.
Discussion
The experience about open policy practice demonstrates that the method works as expected if the participants are committed to the methods, practices, and tools. However, there have been less participants in most open assessments that what was hoped for, and the number of experts or decision makers who actively read from or contribute to Opasnet website has remained lower than expected, although 90000 pageloads per year is still a fair amount. This is partly due to insufficient marketing, as reader numbers have gone clearly up with assessments that have gained media coverage and public interest (e.g. the transport and communication strategy).
The principles behind open policy practice are not unique; on the contrary, they have mostly been borrowed from good practices of various disciplines. Many principles from the original collection[10] have increased in popularity. Openness in science is a current megatrend, and its importance has been accepted much more widely than what was the case in 2006 when Opasnet was launched. Not surprisingly, there are several other websites and organisations that promote one or more of the same principles. Some of them are described here.
Open Science Framework is a project that aims to increase reproducibility in science by developing structured protocols for reproducing research studies, documenting study designs and results online, and producing open source software and preprint services to support this[51]. The Framework maintains a web-workspace for documenting research as it unfolds rather than only afterwards in articles.
Omidyar Network is an organisation that gives grants to non-profit organisations and also invest in startups that promote e.g. governance and citizen engagement[52]. As an example, they support tools to improve discussion online with annotations[53], an objective similar to with structured discussions.
ArXiv.org is a famous example of preprint servers offering a place for publishing and discussing manuscripts before peer review[54]. Such websites, as well as open access journals, have increased during recent years as the importance of availability of scientific information has been understood. Using open data storages (openscience.fi/ida) for research results are often required by research funders. Also governments have been active in opening data and statistics to wide use (data.gov.uk). Governance practices have been developed towards openness and inclusiveness, promoted by international initiatives such as Open Government Partnership (www.opengovpartnership.org). In brief, facilities for openness and inclusiveness in science and governance are increasing rapidly, and with the current rate, the practices will change radically in the next ten years.
Also non-research domains show increasing openness. Shared online tools such as Google Drive (drive.google.com), Slack (slack.com), and others have familiarised people with online collaboration and idea that information is accessible from anywhere. Open platforms for deliberation of decisions are available (otakantaa.fi, kansalaisaloite.fi), and sharing of code is routinely done via large platforms (github.com, cran.r-project.org). The working environment has changed much faster than the practices in research and societal decision making.
As an extreme, a successful hedge fund Bridgewater Associates implements radical openness and continuous criticism of all ideas presented by its workers rather than letting organisational status determine who is heard[55]. In a sense, they are implementing the scientific method in much more rigorous way than what is typically done in science. All in all, despite challenges in practical implementation, the principles found in open policy practice have gained popularity and produced success stories in many areas, and they are more timely than before.
Despite all this progress, the websites and tools mentioned above do not offer a place for open topic-wise scientific information production and discussion that would also support decision making. This could be achieved by merging the functionalities of e.g. Opasnet, Open Science Framework, open data repositories, and discussion forums. Even if different tasks would happen on separate websites, they could form an integrated system to be used by decision makers, experts, stakeholders, and machines. Resource description framework and ontologies could be helpful in organising such a complex system.
To keep expert and decision making practices up to the recent progress, there is a need for tools and also training designed to facilitate a change. New practices could also be promoted by developing ways to give merit and recognition more directly based on participation in co-creation online. The current publication counts and impact factors are very indirect measures of societal or scientific importance of the information produced.
In this article, we have demonstrated methods and practices that have already been successfully used in decision support. However, there are many parts that have been thought as important parts of open policy practice but that have not yet been extensively tested. There is still a lot to learn about using co-created information in decision making. However, experiences so far have demonstrated that decision making can be more evidence-based than what it is today, and several tools promoting this change are available to us. This is expected to reduce the influence of a single leader or decision maker, resulting in more stable and predictable policies.
Conclusions
In conclusion, we have demontrated that open policy practice works technically as expected. Open assessments can be performed openly online. They do not fail due to reasons many people think they will, namely low quality contributions, malevolent attacks or chaos caused by too many uninformed participants; these phenomena are very rare. Shared understanding has proved to be a useful concept that guides policy processes toward more collaborative approach, whose purpose is wider understanding rather than winning.
However, open policy practice has not been adopted in expert work or decision support as expected. Key hindrances have been that it offers little added efficiency or quality of content for a single task by an expert or a decision maker, although its impacts on the overall process are positive. The increased availability, acceptability, and inter-assessment efficiency have not been recognised by the scientific or policy community.
Active facilitation, community building and improving the user-friendliness of the tools were identified as key solutions in improving usability of the method in the future.
List of abbreviations
- THL: National Institute for Health and Welfare (government research institute in Finland)
- IEHIAS: Integrated Environmental Health Impact Assessment System (a website)
Declarations
- Ethics approval and consent to participate: Not applicable
- Consent for publication: Not applicable
- Availability of data and materials: The datasets generated and/or analysed during the current study are available in the [NAME] repository, [PERSISTENT WEB LINK TO DATASETS] OPASNET AND ANOTHER
- Competing interests: The authors declare that they have no competing interests.
- Funding: This work resulted from the BONUS GOHERR project (Integrated governance of Baltic herring and salmon stocks involving stakeholders, 2015-2018) that was supported by BONUS (Art 185), funded jointly by the EU, the Academy of Finland and and the Swedish Research Council for Environment, Agricultural Sciences and Spatial Planning. Previous funders of the work: Centre of Excellence for Environmental Risk Analysis 2002-2007 (Academy of Finland), Beneris 2006-2009 (EU FP6 Food-CT-2006-022936), Intarese 2005-2011 (EU FP6 Integrated project in Global Change and Ecosystems, project number 018385), Heimtsa 2007-2011 EU FP6 (Global Change and Ecosystems project number GOCE-CT-2006-036913-2), Plantlibra 2010-2014 (EU FP7-KBBE-2009-3 project 245199), Urgenche 2011-2014 (EU FP7 Call FP7-ENV-2010 Project ID 265114), Finmerac 2006-2008 (Finnish Funding Agency for Innovation TEKES), Minera 2010-2013 (European Regional Development Fund), Scud 2005-2010 (Academy of Finland, grant 108571), Bioher 2008-2011 (Academy of Finland, grant 124306), Claih 2009-2012 (Academy of Finland, grant 129341), Yhtäköyttä 2015-2016 (Prime Minister's Office, Finland).
- Authors' contributions: JT and MP jointly developed the open assessment method and open policy practice. JT launched Opasnet web-workspace and supervised its development. TR developed OpasnetUtils software package from an original idea by JT and implemented several assessment models. All authors participated in several assessments and discussions aout methods. JT wrote the first manuscript draft based on materials from MP, PM, AA, and TR. All authors read and approved the final manuscript.
- Acknowledgements: We thank Einari Happonen and Juha Villman for their work in developing Opasnet; and John S. Evans, Alexanda Gens, Patrycja Gradowska, Päivi Haapasaari, Sonja-Maria Ignatius, Suvi Ignatius, Matti Jantunen, Anne Knol, Sami Majaniemi, Kaisa Mäkelä, Raimo Muurinen, Jussi Nissilä, Juha Pekkanen, Mia Pihlajamäki, Teemu Ropponen, Simo Sarkki, Marko Tainio, Peter Tattersall, Jouko Tuomisto, and Matleena Tuomisto for crucial and inspiring discussions about methods and their implementation, and promoting these ideas on several forums.
Endnotes
a This paper has its foundations on environmental health, but the idea of decision support necessarily looks at aspects seen relevant from the point of view of the decision maker, not from that of an expert in a particular field. Therefore, this article and also the method described are deliberately taking a wide view and covering all areas of expertise. However, all practical case studies have their main expertise needs in public health, and often specifically in environmental health. b Whenever this article presents a term in italic (e.g. open assessment), it indicates that there is a page on the Opasnet web-workspace describing that term and that it can be accessed using a respective link (e.g. http://en.opasnet.org/w/Open_assessment). c Extended causal diagram was originally called pyrkilo; the word was invented in 1997. It is Finnish and a free translation is "an object or process that tends to produce or aims at producing certain kinds of products." The reasoning for using the word was that pyrkilo diagrams tend to improve understanding and thus decisions. The first wiki website was also called Pyrkilo, but the name was soon changed to Opasnet. d The database consists of two parts: MongoDB contains the actual data, and a related MySQL database contains metadata about all tables. The first version of the database used only MySQL but it was not optimal for data with no predefined structure.
References and notes
- ↑ 1.0 1.1 1.2 Jussila H. Päätöksenteon tukena vai hyllyssä pölyttymässä? Sosiaalipoliittisen tutkimustiedon käyttö eduskuntatyössä. [Supporting decision making or sitting on a shelf? The use of sociopolitical research information in the Finnish Parliament.] Helsinki: Sosiaali- ja terveysturvan tutkimuksia 121; 2012. http://hdl.handle.net/10138/35919. Accessed 24 Jan 2018. (in Finnish)
- ↑ National Research Council. Risk Assessment in the Federal Government: Managing the Process. Washington DC: National Academy Press; 1983.
- ↑ 3.0 3.1 National Research Council. Understanding risk. Informing decisions in a democratic society. Washington DC: National Academy Press; 1996.
- ↑ Pohjola MV, Leino O, Kollanus V, Tuomisto JT, Gunnlaugsdóttir H, Holm F, Kalogeras N, Luteijn JM, Magnússon SH, Odekerken G, Tijhuis MJ, Ueland O, White BC, Verhagen H. State of the art in benefit-risk analysis: Environmental health. Food Chem Toxicol. 2012;50:40-55.
- ↑ Tapscott D, Williams AD. Wikinomics. How mass collaboration changes everything. USA: Portfolio; 2006. ISBN 1591841380
- ↑ Surowiecki J. The Wisdom of Crowds: Why the Many Are Smarter Than the Few and How Collective Wisdom Shapes Business, Economies, Societies and Nations. USA: Doubleday; Anchor; 2004. ISBN 9780385503860
- ↑ 7.0 7.1 Noveck, BS. Wiki Government - How Technology Can Make Government Better, Democracy Stronger, and Citizens More Powerful. Brookings Institution Press; 2010. ISBN 9780815702757
- ↑ Co-creation: Mauser W, Klepper G, Rice M, Schmalzbauer BS, Hackmann H, Leemans R, Current HM. Transdisciplinary global change research: the co-creation of knowledge for sustainability. Opinion in Environmental Sustainability 2013;5:420–431; doi:10.1016/j.cosust.2013.07.001
- ↑ Pohjola M. Assessments are to change the world. Prerequisites for effective environmental health assessment. Helsinki: National Institute for Health and Welfare Research 105; 2013. http://urn.fi/URN:ISBN:978-952-245-883-4
- ↑ 10.0 10.1 10.2 Tuomisto JT, Pohjola M, editors. Open Risk Assessment. A new way of providing scientific information for decision-making. Helsinki: Publications of the National Public Health Institute B18; 2007. http://urn.fi/URN:ISBN:978-951-740-736-6.
- ↑ 11.0 11.1 11.2 Assessment: http://en.opasnet.org/w/Benefit-risk_assessment_of_Baltic_herring_and_salmon_intake. Accessed 24 Jan 2018.
- ↑ 12.0 12.1 Tuomisto JT. TCDD: a challenge to mechanistic toxicology [Dissertation]. Kuopio: National Public Health Institute A7; 1999.
- ↑ 13.0 13.1 Tuomisto JT, Pekkanen J, Alm S, Kurttio P, Venäläinen R, Juuti S et al. Deliberation process by an explicit factor-effect-value network (Pyrkilo): Paakkila asbestos mine case, Finland. Epidemiol 1999;10(4):S114.
- ↑ 14.0 14.1 Tuomisto JT; Tainio M. An economic way of reducing health, environmental, and other pressures of urban traffic: a decision analysis on trip aggregation. BMC PUBLIC HEALTH 2005;5:123. http://biomedcentral.com/1471-2458/5/123/abstract Assessment: http://en.opasnet.org/w/Cost-benefit_assessment_on_composite_traffic_in_Helsinki. Accessed 24 Jan 2018.
- ↑ Allison DB, Brown AW, George BJ, Kaiser KA. Reproducibility: A tragedy of errors. Nature 2016;530:27–29. doi:10.1038/530027a
- ↑ Aitamurto T, Landemore H. Five design principles for crowdsourced policymaking: Assessing the case of crowdsourced off-road traffic law in Finland. Journal of Social Media for Organizations. 2015;2:1:1-19.
- ↑ von Winterfeldt D. Bridging the gap between science and decision making. PNAS 2013;110:3:14055-14061. http://www.pnas.org/content/110/Supplement_3/14055.full
- ↑ Eemeren FH van, Grootendorst R. A systematic theory of argumentation: The pragma-dialectical approach. Cambridge: Cambridge University Press; 2004.
- ↑ Open Knowledge International. The Open Definition. http://opendefinition.org/. Accessed 24 Jan 2018.
- ↑ Pohjola MV, Pohjola P, Tainio M, Tuomisto JT. Perspectives to Performance of Environment and Health Assessments and Models—From Outputs to Outcomes? (Review). Int. J. Environ. Res. Public Health 2013;10:2621-2642 doi:10.3390/ijerph10072621
- ↑ 21.0 21.1 Sandström V, Tuomisto JT, Majaniemi S, Rintala T, Pohjola MV. Evaluating effectiveness of open assessments on alternative biofuel sources. Sustainability: Science, Practice & Policy 2014;10;1. doi:10.1080/15487733.2014.11908132 Assessment: http://en.opasnet.org/w/Biofuel_assessments. Accessed 24 Jan 2018.
- ↑ Cooke RM. Experts in Uncertainty: Opinion and Subjective Probability in Science. New York: Oxford University Press; 1991.
- ↑ Tuomisto JT, Pohjola M, Pohjola P. Avoin päätöksentekokäytäntö voisi parantaa tiedon hyödyntämistä. [Open policy practice could improve knowledge use.] Yhteiskuntapolitiikka 2014;1:66-75. http://urn.fi/URN:NBN:fi-fe2014031821621 (in Finnish)
- ↑ Pohjola MV. Assessment of impacts to health, safety, and environment in the context of materials processing and related public policy. In: Bassim N, editor. Comprehensive Materials Processing Vol. 8. Elsevier Ltd; 2014. pp 151–162. doi:10.1016/B978-0-08-096532-1.00814-1
- ↑ Pohjola MV, Tuomisto JT. Openness in participation, assessment, and policy making upon issues of environment and environmental health: a review of literature and recent project results. Environmental Health 2011;10:58 http://www.ehjournal.net/content/10/1/58.
- ↑ W3C. Resource Description Framework (RDF). https://www.w3.org/RDF/. Accessed 24 Jan 2018.
- ↑ Assessment: http://en.opasnet.org/w/Assessment_of_the_health_impacts_of_H1N1_vaccination. Accessed 24 Jan 2018.
- ↑ Assessment: http://en.opasnet.org/w/Tendering_process_for_pneumococcal_conjugate_vaccine. Accessed 24 Jan 2018.
- ↑ Tuomisto JT, Rintala J, Ordén P, Tuomisto HM, Rintala T. Helsingin energiapäätös 2015. Avoin arviointi terveys-, ilmasto- ja muista vaikutuksista. [Helsinki energy decision 2015. An open assessment on health, climate, and other impacts]. Helsinki: National Institute for Health and Welfare. Discussionpaper 24; 2015. http://urn.fi/URN:ISBN:978-952-302-544-8 Assessment: http://en.opasnet.org/w/Helsinki_energy_decision_2015. Accessed 24 Jan 2018.
- ↑ Asikainen A, Pärjälä E, Jantunen M, Tuomisto JT, Sabel CE. Effects of Local Greenhouse Gas Abatement Strategies on Air Pollutant Emissions and on Health in Kuopio, Finland. Climate 2017;5(2):43; doi:10.3390/cli5020043 Assessment: http://en.opasnet.org/w/Climate_change_policies_and_health_in_Kuopio. Accessed 24 Jan 2018.
- ↑ Tuomisto JT, Niittynen M, Pärjälä E, Asikainen A, Perez L, Trüeb S, Jantunen M, Künzli N, Sabel CE. Building-related health impacts in European and Chinese cities: a scalable assessment method. Environmental Health 2015;14:93. doi:10.1186/s12940-015-0082-z Assessment: http://en.opasnet.org/w/Climate_change_policies_in_Basel. Accessed 24 Jan 2018.
- ↑ Taimisto P, Tainio M, Karvosenoja N, Kupiainen K, Porvari P, Karppinen A, Kangas L, Kukkonen J, Tuomisto JT. Evaluation of intake fractions for different subpopulations due to primary fine particulate matter (PM2.5) emitted from domestic wood combustion and traffic in Finland. Air Quality Atmosphere and Health 2011;4:3-4:199-209. doi:10.1007/s11869-011-0138-3 Assessment: http://en.opasnet.org/w/BIOHER_assessment. Accessed 24 Jan 2018.
- ↑ Tainio M, Tuomisto JT, Hanninen O, Aarnio P, Koistinen, KJ, Jantunen MJ, Pekkanen J. Health effects caused by primary fine particulate matter (PM2.5) emitted from buses in the Helsinki metropolitan area, Finland. RISK ANALYSIS 2005;25:1:151-160. Assessment: http://en.opasnet.org/w/Gasbus_-_health_impacts_of_Helsinki_bus_traffic. Accessed 24 Jan 2018.
- ↑ Tuomisto JT, Niittynen M, Turunen A, Ung-Lanki S, Kiviranta H, Harjunpää H, Vuorinen PJ, Rokka M, Ritvanen T, Hallikainen A. Itämeren silakka ravintona – Hyöty-haitta-analyysi. [Baltic herring as food - a benefit-risk analysis] ISBN 978-952-225-141-1. Helsinki: Eviran tutkimuksia 1; 2015 (in Finnish). Assessment: http://fi.opasnet.org/fi/Silakan_hy%C3%B6ty-riskiarvio. Accessed 24 Jan 2018.
- ↑ Leino O, Karjalainen AK, Tuomisto JT. Effects of docosahexaenoic acid and methylmercury on child's brain development due to consumption of fish by Finnish mother during pregnancy: A probabilistic modeling approach. Food Chem Toxicol. 2013;54:50-8. doi:10.1016/j.fct.2011.06.052. Assessment: http://en.opasnet.org/w/Benefit-risk_assessment_of_methyl_mercury_and_omega-3_fatty_acids_in_fish. Accessed 24 Jan 2018.
- ↑ Gradowska PL. Food Benefit-Risk Assessment with Bayesian Belief Networks and Multivariable Exposure-Response. Delft: Delft University of Technology (doctoral dissertation); 2013. https://repository.tudelft.nl/islandora/object/uuid:9ced4cb2-9809-4b58-af25-34e458e8ea23/datastream/OBJ Assessment: http://en.opasnet.org/w/Benefit-risk_assessment_of_fish_consumption_for_Beneris. Accessed 24 Jan 2018.
- ↑ Tuomisto JT, Tuomisto J, Tainio M, Niittynen M, Verkasalo P, Vartiainen T et al. Risk-benefit analysis of eating farmed salmon. Science 2004;305(5683):476. Assessment: http://en.opasnet.org/w/Benefit-risk_assessment_on_farmed_salmon. Accessed 24 Jan 2018.
- ↑ Leino O, Tainio M, Tuomisto JT. Comparative risk analysis of dioxins in fish and fine particles from heavy-duty vehicles. Risk Anal. 2008;28(1):127-40. Assessment: http://en.opasnet.org/w/Comparative_risk_assessment_of_dioxin_and_fine_particles. Accessed 24 Jan 2018.
- ↑ Assessment: http://en.opasnet.org/w/Compound_intake_estimator. Accessed 24 Jan 2018.
- ↑ Kauppila T, Komulainen H, Makkonen S, Tuomisto JT, editors. Metallikaivosalueiden ympäristöriskinarviointiosaamisen kehittäminen: MINERA-hankkeen loppuraportti. [Summary: Improving Environmental Risk Assessments for Metal Mines: Final Report of the MINERA Project.] Helsinki: Geology Survey Finland, Research Report 199; 2013. 223 p. ISBN 978-952-217-231-0. Assessment: http://fi.opasnet.org/fi/Minera-malli. Accessed 24 Jan 2018.
- ↑ Assessment: http://fi.opasnet.org/fi/Kaivosvesien_riskit_(KAVERI-malli). Accessed 24 Jan 2018.
- ↑ Assessment: http://en.opasnet.org/w/Water_guide. Accessed 24 Jan 2018.
- ↑ Liikenne ja viestintä digitaalisessa Suomessa. Liikenne- ja viestintäministeriön tulevaisuuskatsaus 2014 [Transport and and communication in digital Finland] Helsinki: Ministry of Transport and Communication; 2014. http://urn.fi/URN:ISBN:978-952-243-420-3 Assessment: http://fi.opasnet.org/fi/Liikenne_ja_viestint%C3%A4_digitaalisessa_Suomessa_2020. Accessed 24 Jan 2018.
- ↑ Tuomisto JT, Muurinen R, Paavola J-M, Asikainen A, Ropponen T, Nissilä J. Tiedon sitominen päätöksentekoon. [Binding knowledge to decision making] Helsinki: Publications of the Government's analysis, assessment and research activities 39; 2017. ISBN 978-952-287-386-6 http://tietokayttoon.fi/julkaisu?pubid=19001. Assessment: http://fi.opasnet.org/fi/Maahanmuuttoarviointi. Accessed 24 Jan 2018.
- ↑ Wikipedia: Wikipedians. https://en.wikipedia.org/wiki/Wikipedia:Wikipedians. Accessed 24 Jan 2018
- ↑ Giles J. Internet encyclopaedias go head to head. Nature 2005;438:900–901 doi:10.1038/438900a
- ↑ Walco DK, Risen, JL. The Empirical Case for Acquiescing to Intuition. PSYCHOLOGICAL SCIENCE 2017;28:12:1807-1820. doi:10.1177/0956797617723377
- ↑ Dufva M, Halonen M, Kari M, Koivisto T, Koivisto R, Myllyoja J. Kohti jaettua ymmärrystä työn tulevaisuudesta [Toward a shared understanding of the future of work]. Helsinki: Prime Minister's Office: Publications of the Govenrment's analysis, assessment and research activities 33; 2017. (in Finnish) http://tietokayttoon.fi/julkaisu?pubid=18301. Accessed 24 Jan 2018.
- ↑ Oksanen K. Valtioneuvoston tulevaisuusselonteon 1. osa. Jaettu ymmärrys työn murroksesta [Government Report on the Future Part 1. A shared understanding of the transformation of work] Prime Minister’s Office Publications 13a; 2017. (in Finnish) http://urn.fi/URN:ISBN:978-952-287-432-0. Accessed 24 Jan 2018.
- ↑ Pande M. Wikipedia editors do it for fun: First results of our 2011 editor survey. 2011. https://blog.wikimedia.org/2011/06/10/wikipedia-editors-do-it-for-fun-first-results-of-our-2011-editor-survey/. Accessed 24 Jan 2018.
- ↑ Open Science Framework. https://osf.io/. Accessed 24 Jan 2018.
- ↑ Omidyar Network. A world of positive returns. http://www.omidyar.com. Accessed 24 Jan 2018.
- ↑ Hypothesis. Annotate the web, with anyone, anywhere. https://web.hypothes.is/. Accessed 24 Jan 2018.
- ↑ Cornell University Library. arXiv.org. https://arxiv.org/. Accessed 24 Jan 2018.
- ↑ Dalio R. Principles: Life and work. New York: Simon & Shuster; 2017. ISBN 9781501124020
Figures and tables
Move them here for submission.
Appendix 1: Objects and ontologies
-
Figure A1-1. Extended causal diagram about dioxins in Baltic fish. The focus is on reasoning and value judgements and their connections to causal chains about dioxins and health.
-
Figure A1-2. Legend for different objects used in extended causal diagrams.
- From Universal object
⇤--#: . Määritelmät uusittava. Myös muut oliot --Jouni (talk) 15:11, 8 January 2018 (UTC) (type: truth; paradigms: science: attack)
We wanted to develop a simple first classification for information objects and concluded that all relevant information falls into the following broad topical information areas. First, there are substantive information about the actions, their impacts, and finally objectives. Second, there is information about the decision process, e.g. about who has the power to decide and when. Third, there are stakeholders, i.e. participants and other people and organisations that are relevant in the decision process or information production. Fourth, tasks are performed to produce all the information needed for a good decision. Fifth, there are information methods such as open assessment or statistical analyses that describe how the information work should be performed. Finally, if none of these topical areas fits, then the piece is probably irrelevant to the decision. However, sometimes this irrelevance is important to document explicitly to discourage its unsubstantiated use.
Object | Topical information areas | Description |
---|---|---|
Assessment | Tasks | Assessment is a process for describing a particular piece of reality in aim to fulfil a certain information need in a decision-making situation. The word assessment can also mean the end product of this process, i.e. some kind of assessment report. Often it is clear from the context whether assessment means the doing of the report or the report itself. Methodologically, these are two different objects, called the assessment process and the assessment product, respectively. Unlike other universal objects, assessments are discrete objects having defined starting and ending points in time and specific contextually and situationally defined goals. Decisions included in an assessment are described within the assessment, and they are no longer described as variables. In R, there was previously an S4 object called oassessment, but that is rarely if at all used nowadays. |
Variable | Substance | Variable is a description of a particular piece of reality. It can be a description of physical phenomena, or a description of value judgments. Variables are continuously existing descriptions of reality, which develop in time as knowledge about them increases. Variables are therefore not tied into any single assessment, but instead can be included in other assessments. Variable is the basic building block of describing reality. In R, variables are implemented using an S4 object called ovariable. |
Method | Methods | Method is a systematic procedure for a particular information manipulation process that is needed as a part of an assessment work. Method is the basic building block for describing the assessment work (not reality, like the other universal objects). In practice, methods are "how-to-do" descriptions about how information should be produced, collected, analysed, or synthesised in an assessment. Some methods can be about managing other methods. Typically, methods contain a software code or another algorithm to actually perform the method easily. Previously, there was a subcategory of method called tool, but the difference was not clear and the use of tool is depreciated as a formal object. In R, methods are typically ovariables that contain dependencies and formulas for computing the result, but some context-specific information about dependencies are missing. Therefore, the result cannot be computed until the method is used within an assessment. |
Study | Substance | Study is an information object that describes a research study and its answers, i.e. observational or other data obtained. The study methods are described as the rationale of the object. Unlike traditional research articles, there is little or no discussion, because the interpretation of the results happens in other objects, typically in variables for which the study contains useful information. A major difference to a variable is that the rationale of the study is fixed after the research plan has been fixed and work done, and also the answer including the study results is fixed after the data has been obtained and processed. The question (or scope) of a study reflects the generalisability of the study results, and it is open to discussion and subject to change also after the study has been finished. In contrast, in a variable the question is typically fixed, and the answer and rationale change as new information comes up. |
Lecture | Substance | Lecture contains a piece of information that is to be mediated to a defined audience and with a defined learning objective. It can also be seen as a process during which the audience learns, instead of being a passive recipient of information. |
Encyclopedia article | Substance | Encyclopedia articles are objects that do not attempt to answer a specific research question. Instead, they are general descriptions about a topic. They do not have a universal attribute structure. |
Nugget | Substance | Nugget is an object that was originally designed to be written by a dedicated (group of) person(s). Nuggets are not freely editable by others. Also, they do not have a universal structure. |
Decision process | ||
Discussion | Any | |
User pages | Stakeholders | |
Irrelevant issues |
Label | Description | Properties or research question |
---|---|---|
open policy practice | framework for planning, making, and implementing decisions | |
open assessment | method, information object | How can information be organised for support societal decision making when open participation is allowed? |
variable | information object | |
method | information object | |
question | part of information object | |
answer | part of information object | |
rationale | part of information object | |
knowledge crystal | information object | |
discussion | information object | |
statement | part of discussion | |
resolution | part of discussion | |
argumentation | part of discussion | |
argument | part of argumentation | |
shared understanding | state of the world, information object, phase in information work | |
intentionality | principle in execution | |
causality | principle in execution | |
criticism | principle in execution | |
shared information objects | principle in execution | |
openness | principle in execution | |
reuse | principle in execution | |
execution | phase in information work | |
evaluation and management | phase in information work | |
co-creation and facilitation | phase in information work | |
decision support | step in decision process | |
decision making | step in decision process | |
implementation | step in decision process | |
properties of good assessment | evaluation criterion, part of evaluation and management | |
setting of assessment | evaluation criterion, part of evaluation and management | |
dimension of openness | evaluation criterion, part of evaluation and management | |
category of interaction | evaluation criterion, part of evaluation and management | |
quality of content | property of decision support | Contains specificity, exactness and correctness of information in relation to the research question. |
applicability | property of decision support | Contains properties in relation to the user needs in a decision process |
efficiency | property of decision support | Contains properties in relation to expenditure of producing the assessment output either in one assessment of in a series of assessments |
relevance | part of applicability | |
availability | part of applicability | |
usability | part of applicability | |
acceptability | part of applicability | |
impacts | setting of assessment | |
causes | setting of assessment | |
problem owner | setting of assessment | |
target users | setting of assessment | |
interaction | setting of assessment | |
scope of participation | dimension of openness | |
access to information | dimension of openness | |
timing of openness | dimension of openness | |
scope of contribution | dimension of openness | |
impact of contribution | dimension of openness | |
isolated | category of interaction | |
informing | category of interaction | |
participatory | category of interaction | |
joint | category of interaction | |
shared | category of interaction | |
assessor | a role in decision process | |
manager | a role in decision process | |
stakeholder | a role in decision process |
Appendix 2: Workspace tools: OpasnetUtils package and Opasnet Base
- From Ovariable
This structure is used in R statistical software when building impact assessment models.
Question
What is the structure of an ovariable such that
- it complies with the requirements of variable and
- it is able to implement probabilistic descriptions of multidimensional variables and
- it is able to implement different scenarios?
Answer
The ovariable is a class S4 object defined by OpasnetUtils in R software system. Its purpose is to contain the current best answer in a machine-readable format (including uncertainties when relevant) to the question asked by the respective variable. In addition, it contains information about how to derive the current best answer. The respective variable may have an own page in Opasnet, or it may be implicit so that it is only represented by the ovariable and descriptive comments within a code.
It is useful to clarify terms here. Answer is the overall answer to the question asked, so it is the reason for producing the Opasnet page in the first place. This is why it is typically located near the top of an Opasnet page. The answer may contain text, tables, or graphs on the web page. It typically also contains an R code with a respective ovariable, and the code produces these representations of the answer when run. (However, the ovariable is typically defined and stored under Rationale/Calculations, and the code under Answer only evaluates and plots the result.) Output is the key part (or slot) of the answer within an ovariable. All other parts of the ovariable are needed to produce the output, and the output contains what the reader wants to know about the answer. Finally, Result is the key column of the Output table (or data.frame) and contains the actual numerical values for the answer.
OpasnetUtils Contains tools for open assessment and modelling in Opasnet, a wiki-based web site and workspace for societal decision making (see <http://en.opasnet.org/w/Main_Page> for more information). The core principle of the workspace is maximal openness and modularity. Variables are defined on public wiki pages using wiki inputs/tables, databases and R code. This package provides the functionality to download and use these variables. It also contains health impact assessment tools such as spatial methods for exposure modelling.
Slots
An ovariable has the following separate slots that can be accessed using X@slot (where X is the name of the ovariable):
- @name
- Name of <self> (the ovariable object) is a requirement since R doesn't support self reference.
- @output
- The current best answer to the question asked.
- A single data.frame (a 2D table type in R)
- Not defined until <self> is evaluated.
- Possible types of columns:
- Result is the column that contains the actual values of the answer to the question of the variable. There is always a result column, but its name may vary; it is of type ovariablenameResult.
- Indices are columns that define or restrict the Result in some way. For example, the Result can be given separately for males and females, and this is expressed by an index column Sex, which contains values Male and Female. So, the Result contains one row for males and one for females.
- Iter is a special kind of index used in Monte Carlo simulations. Iter contains the number of the iteration.
- Unit contains the unit of the Result. It may be the same for all rows, but it may also vary from one row to another. Unit is not an index.
- Other, non-index columns can exist. Typically, they are information that were used for some purpose during the evolution of the ovariable, but they may be useless in the current ovariable. Due to these other columns, the output may sometimes be a very wide data.frame.
- @data
- A single data.frame that defines <self> as such.
- data slot answers this question: What measurements are there to answer the question? Typically, when data is used, the result can be directly derived from the information given (with possibly some minimal manipulation such as dropping out unnecessary rows).
- May include textual regular expressions that describe probability distributions which can be interpreted by OpasnetUtils/Interpret.
- @marginal
- A logical vector that indicates full marginal indices (and not parts of joint distributions, result columns or other row-specific descriptions) of @output.
- @formula
- A function that defines <self>.
- Should return either a data.frame or an ovariable.
- @formula and @dependencies slots are always used together. They answer this question: How can we estimate the answer indirectly? This is the case if we have knowledge about how the result of this variable depends on the results of other variables (called parents). The @dependencies is a table of parent variables and their identifiers, and @formula is a function that takes the results of those parents, applies the defined code to them, and in this way produces the @output for this variable.
- @dependencies
- A data.frame that contains names and Rtools or Opasnet tokens/identifiers of variables required for <self> evaluation (list of causal parents). The following columns may be used:
- Name: name of an ovariable or a constant (one-row numerical vector) found in the global environment (.GlobalEnv).
- Key: the run key (typically a 16-character alphanumeric string) to be used in objects.get() function where the run contains the dependent object.
- Ident: Page identifier and rcode name to be used in objects.latest() function where the newest run contains the dependent object. Syntax: "Op_en6007/answer".
- A way of enabling references in R (for in ovariables at least) by virtue of OpasnetUtils/ComputeDependencies which creates variables in .GlobalEnv so that they are available to expressions in @formula.
- Variables are fetched and evaluated (only once by default) upon <self> evaluation.
- @ddata
- A string containing an Opasnet identifier e.g. "Op_en1000". May also contain a subset specification e.g. "Op_en1000/dataset".
- This identifier is used to download data from the Opasnet database for the @data slot (only if empty by default) upon <self> evaluation.
- By default, the data defined by @ddata is downloaded when an ovariable is created. However, it is also possible to create and save an ovariable in such a way that the data is downloaded only when the ovariable is evaluated.
- $meta
- A list of descriptive information of the object. Typical information include date created, username of the creator, page identifier for the Opasnet page with the ovariable code, and identifier of the model run where the object was created.
Decisions and other upstream orders
The general idea of ovariables is such that they should not be modified to match a specific model but rather define the variable in question as extensively as possible under it's scope. In other words, it should answer its question in a reusable way so that the question and answer would be useful in many different situations. (Of course, this should be kept in mind already when the question is defined.) To match the scope of specific models, ovariables can be modified by supplying orders upstream. Evaluating a latent ovariable first triggers the evaluation of its unevaluated parent ovariables (listed in @dependencies) since their results are needed to evaluate the child. This chain of evaluation calls forms a recursion tree in which each upstream variable is evaluated exactly once (cyclical dependencies are not allowed). Decision orders about upstream variables are checked and applied upon their evaluation and propagate downstream to the first variable being evaluated. For example decisions in decision analysis can be supplied this way:
- pick an endpoint
- make decision variables for any upstream variables (this means that you create new scenarios with particular deviations from the actual or business-as-usual answer of that variable)
- evaluate endpoint
- optimize between options defined in decisions.
⇤--#: . Recursion must be explained properly. --Jouni (talk) 15:11, 8 January 2018 (UTC) (type: truth; paradigms: science: attack)
- ----#: . I modified the description above slightly. Perhaps it is more clear. --Teemu R (talk) 11:26, 14 February 2018 (UTC) (type: truth; paradigms: science: comment)
Other orders include: collapse of marginal columns by sums, means or sampling to reduce data size and passing input from model level without redefining the whole variable. It is also possible to redefine any specific variable before starting the recursive evaluation, in which case the recursion stops at the defined variable (dependencies are only fetched if they do not already exist; this is to avoid unnecessary computation).
Opasnet Base
⇤--#: . No references to Opasnet Base 1. --Jouni (talk) 15:11, 8 January 2018 (UTC) (type: truth; paradigms: science: attack)
- From Opasnet Base
How to improve the existing Opasnet Base? Following issues of Opasnet Base 1 must be resolved:
- Opasnet Base 1 structure makes filtering by location very slow on big data
- Opasnet Base 1 structure is perhaps unnecessarily complex = queries are hard to adapt and read
- MySQL is not ideal for storing huge amounts of data, or at least this is how we assume?
- MySQL tables have fixed column types = difficult to store data objects with varying types of indices into one data table
- Multiple languages (localization) not supported
Answer
MySQL is good on relations but weak on dynamic big data. Let's keep the basic "scaffold" in MySQL and store the big data (locations and results) into noSQL-base. After few days of research the best candidate for noSQL-base seems to be MongoDB. Combining relational MySQL and non-relational MongoDB will be the foundation for the new Opasnet Base 2.
⇤--#: . Ahjo is not a decision support tool. It is only a decision document archive and retrieval system. And it is not open source code. --Jouni (talk) 15:04, 21 December 2017 (UTC) (type: truth; paradigms: science: attack)
----#: . Add a Type for managing the decision process. E.g. Ahjo belongs to that category, not decision support --Jouni (talk) 09:24, 22 December 2017 (UTC) (type: truth; paradigms: science: comment)
----#: . Add Open Science Framework, look at en:Project Management Body of Knowledge, en:Comparison of project management software. Omidyar Network. RDF database should also be linked to kansalaisaloite.fi and kuntakuuleminen (?) --Jouni (talk) 09:24, 22 December 2017 (UTC) (type: truth; paradigms: science: comment)
Priority is given to open source solutions and finnish examples
Tietotyyppi | Toiminnallisuus tai työvaihe | Olemassaoleva työkalu |
---|---|---|
Kokonaisuuden hallinta | Kokonaisvaltainen tietotuki päätösvalmistelussa. | Ei olemassaolevaa työkalua kaikkien toiminnallisuuksien yhteenvetämiseen. Kehitystyölle olisi tarvetta. |
Asiakysymykset | Aloitteenteko | Avoin ministeriö, Kansalaisaloite, Nuortenideat, Kuntalaisaloite. Aloitteentekomahdollisuus kansalaisille. Vastaava järjestelmä voisi olla myös valtioneuvoston tai eduskunnan sisältä tulevien ehdotusten esittelyyn. |
Asianhallinta | Diaari, asianhallintajärjestelmät. Avoimen koodin ratkaisu puuttuu. VAHVA-hanke tuottaa tietoa ja järjestelmiä asianhallinnan tueksi. | |
Tutkimustiedonhallinta | Avaa, Ida, Etsin ja muut CSC:n tarjoamat datamanagerointityökalut auttavat hallitsemaan tutkimustiedoa datantuotannosta ja analyyseistä aina arkistointiin asti. Avoin data, tietoaineistojen avoin julkaisualusta. Erityisohjelmia on lukuisia, esimerkiksi QGIS paikkatiedon käsittelyyn. Useilla tieteenaloilla on omat tutkimustietokantansa kuten ArXiv.org (fysiikan artikkeleita) ja lukuisat biologiset tietokannat. | |
Julkinen keskustelu, väitteet ja lausunnot | Otakantaa, Yrpri.org, Facebook, Twitter, blogit, verkkomediat ja muut foorumit keskusteluun. Tiedon tarkistamisen sivustoja: Faktabaari, Fullfact, Need to know -projekti faktantuottamiseen. Agoravoting on avoin sähköinen äänestysjärjestelmä. Lausuntopalvelu, joka Otakantaa-sivuston kanssa on osa tulevaa valtionhallinnon yhteistä asianhallintaa. | |
Uutiset | Uutisseuranta: avoimen koodin vaihtoehtoja esim. CommaFeed tai Tiny Tiny RSS. Semanttiset, automatisoidut tietohaut: esim Leiki. | |
Päätöstilanteiden ja syysuhteiden kuvaus ja arviointi | Opasnet avointen arviointien ja vaikutusmallien toteuttamiseen. Tietokiteet mallien ja kuvausten rakenneosina. Simantics System Dynamics semanttisiin malleihin. Wikidata, Wikipedia rakenteisen tiedon varastoina. | |
Kytkennät lakeihin ja muihin päätöksiin | Semanttinen Finlex sisältää Suomen koko lainsäädännön ja mm. korkeimman oikeuden päätökset rakenteisessa muodossa. | |
Menetelmät | Asiakirjojen valmistelu, yhteiskirjoittaminen | Ahjo-päätösvalmistelumenetelmänä ja yhteiskirjoitustyökalut, esim. Hackpad, MS Office365, Google Docs, Etherpad, Dropbox Paper, MediaWiki ja Git, mahdollistavat valmistelun avaamisen. |
Hyvien käytäntöjen kehittäminen ja levitys | Innokylä auttaa kehittämään toimintaa nopeammin, kun voi oppia toisilta. Freecoin on lohkoketjuihin perustuva palkitsemisjärjestelmä. | |
Keskustelun ja tietojen jäsennysmenetelmät | Decentralised social networking protocol Activitypub
Työkaluja: faktantarkistusmenetelmä Compendium. Kommenttien ja arvojen koneellinen analysointi: Happy City. Sanastoja ja rakenteisen tiedon työkaluja: Resourse Description Framework (RDF), ArgDF (argumentaatio-ontologia), Legal Knowledge Interchange Format (LKIF). Toimivat perustana keskustelun ja tiedon tiivistämiselle, jäsentämiselle ja jakamiselle. | |
Informaatiomuotoilu ja visualisaatiot | Interaktiiviset ja staattiset visualisaatiot monimutkaisesta datasta. Työkaluja esim. Shiny, Gapminder, Lucify | |
Työt | Päätös-, tutkimus- ja muiden prosessin seuranta ja dokumentointi | Ahjo-päätöksenteon hallintajärjestelmä, Hankegalleria VN-TEAS-hankkeille. Projektinhallintatyökaluja: OpenProject, Git. Mahdollistavat tutkimus- ja päätösprosessien ristiinraportoinnin.
Solved. Tutustu ja mieti soveltuvuutta. Fingertip. Suomalaisfirma jolla pystytään kuvaamaan koko prosessi ja näkyvää kuka teki mitä. T sampo alusta. Suomalainen tutustu. |
Kokeilujen kehittäminen, rahoitus ja hallinta | Kokeilun paikka edistää kokeiluja silloin, kun tapaukseen soveltuvaa tietoa ei ole tarjolla. | |
Osaajat | Asiantuntijuuspalvelut | Network of innovators, ResearchGate ja muut asiantuntijoiden verkostot. Kuuma linja (luku 5.5.2) auttaa löytämään asiantuntijoita tai suoraan vastauksia päätöksenteon kysymyksiin. |
See also
- Shared understanding
- Structure of shared understanding
- Open assessment
- Open policy practice
- Properties of good assessment
- op_fi:Yhtäköyttä-hankkeen loppuraportti
- op_fi:Valassafari.
- op_fi:Hiilineutraali Helsinki 2035
- Help:Extended causal diagram
- Open policy practice
- Shared understanding
- Discussion
- Properties of good assessment
- Evaluating performance of environmental health assessments
- Benefit-risk assessment of Baltic herring and salmon intake
- op_fi:Jaetun ymmärryksen menetelmä
- op_fi:Yhtäköyttä-hankkeen loppuraportti
- en:Graph theory
- Helsinki climate program 2035
- Daniel K. Walco, Jane L. Risen. The Empirical Case for Acquiescing to Intuition [2]
- [www.opasnet.org/testiwiki/index.php/Item:Q18 Testi-DRF:action]
- Study: Wikipedia as accurate as Britannica. The journal Nature says the open-access encyclopedia is about as accurate as the old standby.[3]
- Tutkijat pelkäävät jakaa dataansa, vaikka avoimuus voisi lievittää pelkoa [4]
- Laine, H., (2017). Afraid of Scooping – Case Study on Researcher Strategies against Fear of Scooping in the Context of Open Science. Data Science Journal. 16, p.29. http://doi.org/10.5334/dsj-2017-029
- MILDRED. The research data infrastructure for open data in the Univesity of Helsinki [5]
- The Availability of Research Data Declines Rapidly with Article Age [6]
- This is Not a Boundary Object: Reflections on the Origin of a Concept. Susan Leigh Star [7]
- There's no such thing as 'sound science' [8]
- Metayliopisto [9]
- File:Use of risk assessment in the society.ppt
- The politics of evidence revisited [10]