Training assessment: Difference between revisions
Jump to navigation
Jump to search
(→Rationale: No additional " " allowed in decision tables.) |
(→Calculations: Fixed) |
||
Line 89: | Line 89: | ||
library(xtable) | library(xtable) | ||
library(ggplot2) | library(ggplot2) | ||
net.cost <- new("ovariable", | net.cost <- new("ovariable", | ||
Line 230: | Line 111: | ||
) | ) | ||
decisions <- opbase.data("Op_en5677.decisions") | |||
decisions <- | |||
DecisionTableParser(decisions) | DecisionTableParser(decisions) | ||
Fetch2(data.frame(Name = "health.impact", Key = "iaTGnY1hgUP5tofT")) | Fetch2(data.frame(Name = "health.impact", Key = "iaTGnY1hgUP5tofT")) | ||
CheckDecisions(health.impact) | health.impact <- EvalOutput(health.impact) | ||
health.impact <- CheckDecisions(health.impact) | |||
colnames(health.impact@output) | |||
print(xtable(health.impact@output[1:20,]), type = "html") | |||
probabilities <- new("ovariable", | probabilities <- new("ovariable", | ||
Line 250: | Line 127: | ||
) | ) | ||
summary(endpoints) | #summary(endpoints) | ||
decisions | #decisions | ||
summary(probabilities) | #summary(probabilities) | ||
</rcode> | </rcode> |
Revision as of 12:53, 31 December 2012
Moderator:Jouni (see all) |
This page is a stub. You may improve it into a full page. |
Upload data
|
This is a training assessment about an imaginary, simple case. The purpose is to illustrate assessment functionalities.
Scope
Question
What decisions are worth implementing in the training assessment?
Boundaries
- Time: Year 2012 - 2020
Scenarios
- Factory can reduce emissions, or continue business as usual.
- School can increase health education, decrease it to save money, or continue business as usual.
Intended users
- Anyone who wants to learn to make open assessments.
Participants
- Main participants:
- YMAL,
- Summer workers of YMAL in 2012,
- Participants of [[Decision analysis and risk management 2013}}
Answer
Conclusions
Results
Not yet available.
Rationale
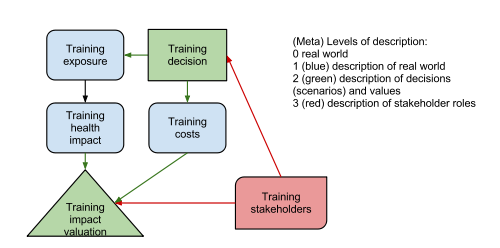
Assessment-specific data
- Decisions
Obs | Decisionmaker | Decision | Option | Variable | Cell | Change | Unit | Amount | Description |
---|---|---|---|---|---|---|---|---|---|
1 | Factory | Cleaning.policy | Reduce emissions | exposure | Year:2020 | Multiply | - | 0.5 | |
2 | School | Health.promotion | Increase health education | health.impact | Year:2020 | Multiply | - | 0.9 | |
3 | School | Health.promotion | Promotion budget reduced | health.impact | Year:2020 | Multiply | - | 1.1 |
- Probabilities
Obs | Stakeholder | Variable | Cell | Probability | Description |
---|---|---|---|---|---|
1 | City of Kuopio | exposure | Cleaning.policy: Reduce emissions | 0.8 | |
2 | City of Kuopio | exposure | Cleaning.policy: BAU | 0.2 | |
3 | Factoy | health.impact | Health.promotion: Increase health education | 0.1 | |
4 | Factoy | health.impact | Health.promotion: Promotion budget reduced | 0.4 | |
5 | Factoy | health.impact | Health.promotion: BAU | 0.5 |
- Endpoints
Obs | Stakeholder | Variable | Cell | Model | Result | Description |
---|---|---|---|---|---|---|
1 | City of Kuopio | health.impact | Year:2012 | Weighted sum | 1000 | |
2 | City of Kuopio | health.impact | Year:2020 | Weighted sum | 1000 | |
3 | Citizens | health.impact | Year:2012 | Weighted sum | 1000 | |
4 | Citizens | health.impact | Year:2020 | Weighted sum | 2000 | Future years are twice as important. |
- Variables
- exposure: Training exposure
- health.impact: Training health impact
- training.costs: Training costs
- Analyses
- Decision analysis on each policy: Which option minimises the health risks?
- Value of information (VOI) analysis for each policy about the major variables in the model and the total VOI.
Calculations
See also
Help pages | Wiki editing • How to edit wikipages • Quick reference for wiki editing • Drawing graphs • Opasnet policies • Watching pages • Writing formulae • Word to Wiki • Wiki editing Advanced skills |
Training assessment (examples of different objects) | Training assessment • Training exposure • Training health impact • Training costs • Climate change policies and health in Kuopio • Climate change policies in Kuopio |
Methods and concepts | Assessment • Variable • Method • Question • Answer • Rationale • Attribute • Decision • Result • Object-oriented programming in Opasnet • Universal object • Study • Formula • OpasnetBaseUtils • Open assessment • PSSP |
Terms with changed use | Scope • Definition • Result • Tool |
- Descriptions of a previous structure
- ----#: . Päätöksenteon sokea piste: se mitä ihmiset eivät näe mutta eivät myöskään huomaa etteivät näe. Kuitenkin tutkimalla sitä mitä mitä ihmiset eivät näe saadaan selville asioita sokeasta pisteesta. Ymmärtämällä sokeaa pistettä voidaan keksiä asioita jotka järjestelmällisesti jäävät huomaamatta ja asioita, joilla voidaan korjata järjestelmällisiä puutteita. Avoin arviointi on tämmöinen päätöksenteon järjestelmällisten puutteiden korjausmekanismi. --Jouni 08:55, 1 May 2012 (EEST) (type: truth; paradigms: science: comment)
References
Related files
<mfanonymousfilelist></mfanonymousfilelist>