Water guide: Difference between revisions
(→Answer) |
|||
(92 intermediate revisions by 3 users not shown) | |||
Line 8: | Line 8: | ||
[[File:Water risk assessment.png|thumb|500px]] | [[File:Water risk assessment.png|thumb|500px]] | ||
'''Water guide - An assessment of the health impacts of water quality''' addresses the potential microbiological health risks of drinking water. These microbiological risks are due to the contamination of raw water with microbes that cause potential health problems for people using tap water, as well as the efficiency of drinking water treatment, which can be insufficient for removing harmful microbes from drinking water. The assessment will be used to determine, how high the health risk is from certain microbes in raw water. The assessment is based on a mathematical Water guide -model, which can be found below. | '''Water guide - An assessment of the health impacts of water quality''' addresses the potential microbiological health risks of drinking water. These microbiological risks are due to the contamination of raw water with microbes that cause potential health problems for people using tap water, as well as the efficiency of drinking water treatment, which can be insufficient for removing harmful microbes from drinking water. The assessment will be used to determine, how high the health risk is from certain microbes in raw water. The assessment is based on a mathematical Water guide -model, which can be found below. | ||
Line 17: | Line 16: | ||
==Answer== | ==Answer== | ||
<rcode graphics=1 | <rcode graphics=1 | ||
name="answer1" | name="answer1" | ||
label="Run model" | |||
variables=" | variables=" | ||
name:rawclass|description:Classification of raw water|type:selection| | name:rawclass|description:Classification of raw water|type:selection| | ||
Line 34: | Line 27: | ||
'Surface water - high contamination';Surface water - high contamination; | 'Surface water - high contamination';Surface water - high contamination; | ||
'Ground water - clean';Ground water - clean; | 'Ground water - clean';Ground water - clean; | ||
'Ground water - surface water contamination'; | 'Ground water - surface water contamination';Ground water - surface water contamination; | ||
'Hand input';I will give microbe concentrations of raw water by hand| | |||
default:'Hand input'| | |||
category:Situation| | category:Situation| | ||
name: | name:total_population|default:10000|description:Size of the exposed population| | ||
name:rawconsumption|default:0.25|description:The amount (in liters) of unboiled tap water consumed per person per day| | |||
name:Campylo|description:Campylobacter concentration (microbes/l)|default:0| | |||
category:Microbe concentrations of raw water| | |||
category_conditions:rawclass;'User'| | |||
name:E.coli|description:''E.coli'' O157:H7 concentration (microbes/l)|default:0| | |||
name:Rota|description:Rotavirus concentration (microbes/l)|default:0| | |||
name:Noro|description:Norovirus concentration (microbes/l)|default:0| | |||
name:Sapo|description:Sapovirus concentration (microbes/l)|default:0| | |||
name:Crypto|description:Cryptosporidium concentration (microbes/l)|default:0| | |||
name:Giardia|description:Giardia concentration (microbes/l)|default:0| | |||
name:treatment|description:Water cleaning methods in use|type:checkbox| | name:treatment|description:Water cleaning methods in use|type:checkbox| | ||
options: | options: | ||
' | 'Coagulation and flotation';Coagulation and flotation; | ||
'Slow sand filtration';Slow sand filtration; | 'Slow sand filtration';Slow sand filtration; | ||
'Limestone filtration';Limestone filtration; | 'Limestone filtration';Limestone filtration; | ||
'Active carbon filtration';Active carbon filtration | 'Active carbon filtration';Active carbon filtration| | ||
category:Water cleaning: Cleaning process and chlorination| | category:Water cleaning: Cleaning process and chlorination| | ||
name:chlorinedose|default:0|description:Chlorine dose (mg/l) | name:chlorinedose|default:0|description:Chlorine dose (mg/l)| | ||
name:ozonedose|default:0|description:Ozone dose (mg/l)| | |||
name:uvdose|default:0|description:UV dose (mW/cm^2)| | |||
name:uvtime|default:0|description:UV time (s) | |||
" | " | ||
> | > | ||
Line 60: | Line 65: | ||
library(OpasnetUtils) | library(OpasnetUtils) | ||
library(ggplot2) | library(ggplot2) | ||
objects.latest("Op_en6177", code_name="waterguide") # [[Water guide]] fetch the whole model | |||
# Create ovariables from user input data | # Create ovariables from user input data | ||
RawClass <- Ovariable("RawClass", data=data.frame(RawWaterClass = rawclass, Result=1 | RawClass <- Ovariable("RawClass", data=data.frame(RawWaterClass = rawclass, Result=1)) | ||
Treatment <- Ovariable("Treatment", data=data.frame(TreatmentMethod=c(treatment, "None"), Result=1)) | |||
Treatment <- Ovariable("Treatment", data=data.frame(TreatmentMethod=treatment, | |||
ChlorineDose <- Ovariable("ChlorineDose", data=data.frame(Result=chlorinedose)) | ChlorineDose <- Ovariable("ChlorineDose", data=data.frame(Result=chlorinedose)) | ||
UVdose <- Ovariable("UVdose", data=data.frame(Result=uvdose)) | |||
UVtime <- Ovariable("UVtime", data=data.frame(Result=uvtime)) | |||
OzoneDose <- Ovariable("OzoneDose", data=data.frame(Result=ozonedose)) | |||
RawConsumption <- Ovariable("RawConsumption", data=data.frame(Result=rawconsumption)) | |||
# divide the given population size to different age groups based on age distribution of all of Finland | |||
population <- Ovariable("population", | |||
dependencies=data.frame( | |||
Name=c("total_population") | |||
), | |||
formula=function (...) { | |||
population2 <- Ovariable( | |||
"population2", | |||
ddata="Op_en2949", subset="Population" | |||
) | |||
population2 <- EvalOutput(population2) | |||
population <- population2/oapply(population2, cols = "Age", FUN=sum) * total_population | |||
return(population) | |||
} | |||
) | |||
# if the raw concentrations were chosen to be given by hand, put them into an ovariable | |||
# if some raw water class was chosen, this isn't done, and instead the data for microbe concentrations for that class is used | |||
if (rawclass == "Hand input") { | |||
RawConcentration <- Ovariable( | |||
"RawConcentration", | |||
data=data.frame( | |||
Pathogen=c( | |||
"campylobacter", | |||
"E.coli O157:H7", | |||
"rotavirus", | |||
"norovirus", | |||
"sapovirus", | |||
"cryptosporidium", | |||
"giardia"), | |||
Result=c( | |||
Campylo, | |||
E.coli, | |||
Rota, | |||
Noro, | |||
Sapo, | |||
Crypto, | |||
Giardia) | |||
) | |||
) | |||
} | |||
BoDattr <- EvalOutput(BoDattr, verbose=FALSE, forceEval=TRUE) | |||
# calculate the number of cases | |||
cases <- BoDattr/case_burden | |||
cat("Pathogen concentrations in the raw water, microbes/l.\n") | |||
oprint(summary(RawConcentration)) | |||
cat("Exposure to people, number of microbes ingested in a day with drinking water.\n") | |||
oprint(summary(exposure)) | |||
cat("Disease burden, DALYs caused per day (DALY = disability adjusted life years) \n") | |||
oprint(summary(BoDattr, marginals=c("Response","Exposure_agent"))) | |||
cat("Number of illness cases caused daily by pathogens in drinking water. \n") | |||
oprint(summary(cases, marginals=c("Response","Exposure_agent"))) | |||
cat("exposure \n") | |||
colnames(exposure@output)[exposure@marginal] | |||
cat("MicrobeLogDecrease \n") | |||
colnames(MicrobeLogDecrease@output)[MicrobeLogDecrease@marginal] | |||
) | cat("OzoneEfficiency \n") | ||
colnames(OzoneEfficiency@output)[OzoneEfficiency@marginal] | |||
label <- oapply(BoDattr, c("Exposure_agent", "Iter"), sum) | |||
label <- oapply(unkeep(label, sources=TRUE), NULL, mean, "Iter")@output$BoDattrResult | |||
ggplot( | |||
oapply(unkeep(BoDattr, sources=TRUE), NULL, mean, "Iter")@output, | |||
aes(x=Exposure_agent, weight=BoDattrResult, fill=Response)) + | |||
geom_bar() + | |||
coord_flip() + theme_gray(base_size=24)+ | |||
labs( | |||
title="Disease burden of waterborne microbes", | |||
subtitle=paste("in a population of", format(total_population, scientific = F)), | |||
y = "Disease burden (DALY/day)", | |||
x = "Pathogen") + | |||
annotate("text", x = c(1:6), y = label, label = round(label,2), size=6) | |||
label2 <- oapply(cases, c("Exposure_agent", "Iter"), sum) | |||
label2 <- oapply(unkeep(label2, sources=TRUE), NULL, mean, "Iter")@output$Result | |||
ggplot( | |||
oapply(unkeep(cases, sources=TRUE), NULL, mean, "Iter")@output, | |||
aes(x=Exposure_agent, weight=Result, fill=Response)) + | |||
geom_bar() + | |||
coord_flip() + | |||
theme_gray(base_size=24)+ | |||
labs( | |||
title="Ilnesses caused by waterborne microbes", | |||
subtitle=paste("in a population of", format(total_population, scientific = F)), | |||
y = "Cases of illness", | |||
x = "Pathogen") + | |||
annotate("text", x = c(1:6), y = label2, label = round(label2,2), label=6) | |||
ggplot( | # cases as percent of beachgoers | ||
cases_percent <- cases*100/total_population | |||
label3 <- oapply(cases_percent, c("Exposure_agent", "Iter"), sum) | |||
label3 <- oapply(unkeep(label3, sources=TRUE), NULL, mean, "Iter")@output$Result | |||
ggplot( | |||
oapply(unkeep(cases_percent, sources=TRUE), NULL, mean, "Iter")@output, | |||
aes(x=Exposure_agent, weight=Result, fill=Response)) + | |||
geom_bar() + | geom_bar() + | ||
coord_flip() + | coord_flip() + | ||
theme_gray(base_size=24)+ | |||
labs( | labs( | ||
title=" | title="Proportion of the population that gets ill daily", | ||
subtitle=" | subtitle=paste("in a population of", format(total_population, scientific = F)), | ||
y = " | y = "Proportion of population (%)", | ||
) | x = "Pathogen") + | ||
annotate("text", x = c(1:6), y = label3, label = round(label3,2), label=6) | |||
cat(paste("In Finland, the illness cases due to drinking water should be on average less than 0.01% of the population per year, or 1 case for every 10 000 people. The WHO guidelines give a target of less than 10^-6 DALY per person per year, or 0.01 DALYs per 10 000 people. The graphs here show the case burdens and numbers of cases for each of the pathogens that are caused in one day in a population of", total_population, ", with the average daily cold water consumption of", rawconsumption, "liters per person. \n")) | |||
</rcode> | |||
The result page opens in a new tab by pressing the Run model -box. It will take approximately 40 seconds for the model to run, after which the results will appear on the result page. | |||
=== User instructions for the Water guide -model === | |||
{{greenbox| | |||
'''1. Choose classification of raw water''' | |||
*Ground water - Clean: clean ground water | |||
*Ground water - Surface water load: f.ex. shore infiltration | |||
*Surface water - Low load: relatively clean surface water | |||
*Surface water - Medium load: f.ex. low waste water load | |||
*Surface water - High load: f.ex. waste water load | |||
'''OR''' | |||
Choose 'I will determine microbe concentration by hand'. Write pathogen concentrations in their respective boxes. | |||
'''2. State the concumption of drinking water''' per 24 h in liters per day per person (default 1.153 l/d). | |||
'''3. State the population size of target area.''' (default 100000) | |||
'''4. Choose treatment processes used.''' Obs! You can choose multiple options. If none of the first six treatment processes are used, choose "None of the above cleaning methods". If neither UV nor ozone is used, choose "None of the above disinfection methods". | |||
'''5. Choose whether chlorination is used or not''' by giving the chlorine dose used (mg/l). If there is no chlorination, the dose will be 0 mg/l (default 0 mg/l). The model only calculates the effect of chlorine on the water in treatment plants, and ignores any further cleansing effects residual chlorine may have after the water moves onward to the pipe network. | |||
'''6. You can see the results in a new tab by pressing 'Run model'''' This opens a new tab, in which the results will appear after the model is done running. This takes approximately 45 seconds. | |||
}} | |||
== Rationale == | |||
[[File:Water guide.png|750px]] | |||
:''The model has been translated from Analytica to R. You can find the original model here (page in Finnish, link to the model code at the top): [[:op_fi:Tiedosto:Vesiopas.ANA]]. If the user does not give concentrations for the pathogens, the values on page [[Pathogen concentrations in raw water]] will be used. | |||
Health-based quality requirements and recommendations have been implanted on the quality of household water. In Finland, the ministry of social affairs and health is responsible for the legislation of the quality, and monitoring it is the responsibility of municipal health protection officials. Upholding and developing good water quality require high-standard research and cooperation between different parties. Ground waters and artificial ground waters are usually not disinfected. The chemicals and microbes in raw water and byproducts of disinfection produced in household water production can present health risks to the people using the household water. Water leaving the treatment plant can stay in the pipes for a long time. If the circumstances are favourable for microbial growth, the water can become low in quality and even detrimental to health while in the pipe network. | |||
Household water is produced from either ground or surface water. The problems of these types of waters as raw water are different. Using surface water always requires establishing an actual treatment plant and the associated knowledge, skills, technologies and educated monitoring personnel. Ground water on the other hand doesn't require very complex treatment, so not many treatment plant personnel are needed, and there is less monitoring. Additionally today there are many treatment plants that produce artificial ground water. The qualities of artificial ground water can be similar to good ground waters, but the quality of the water is often only be equal to medium quality surface water. | |||
Treatment of ground water usually includes increasing the pH and and hardness of water. This can be done using different techniques, of which the use of calcium hydroxide and carbon dioxide to regulate pH are the most common. Sometimes also precipitation of iron or manganese is required using strong oxidisers such as permanganate or chlorine. Low quality ground water is also disinfected. | |||
In order to make surface water drinkable, it has to be heavily treated. The hardest thing is to precipitate the humus. This is done by using not only different precipitation chemicals, also for example adjust pH and breaking down the humus with strong oxidisers such as ozone. On top of that, the precipitated chemicals (such as salts of aluminium and iron) need to be removed and water hardness raised so that the pipelines don't disintegrate over time. Also, the population has to be protected from the [https://en.wikipedia.org/wiki/Microorganism microbes] always present in surface waters, so the water needs to be disinfected to remove pathogens. | |||
The quality of water is also affected by the condition of the pipelines and the water spends in them. If there is a lot of coagulation in the pipelines, these dregs include not only different inorganic compounds but also a large amout of bacterial mass. The coagulates in the pipelines and their qualities have started to be researched in Finland only recently. | |||
The purpose of risk assessment is to produce information of the true size of the risk for decision-making. Risk assessment is a scientific process in which experts hold a key role. Because there are any kinds of risks to study, risk assessment processes can be very different. In environmental health risk assessment consists of four steps: | |||
# Identifying the danger (does the variable researched pose a danger to humans?) | |||
# Assessing the exposure-response function (how big a dose results in how big a response?) | |||
# Assessing exposure (how much are humans exposed?) | |||
# Characterising the risk (how high is the health risk?)<ref>http://wanda.uef.fi/tkk/avoin/ymp_terveys/</ref> | |||
All water treatment plants should periodically do risk assessment on on the household water they produce. It is done to identify actions to be taken in different crisis situations and prepare plans for different problematic situations and possible damage. Different treatment plants and for example vacation centers need different kinds of plans and implementations. | |||
=== Dependencies === | |||
{| {{prettytable}} | |||
|+ '''Pages containing the data and codes used by the model | |||
! Page !! What data or code the page has !! What is it used for !! Other observations | |||
|---- | |||
| Op_en2949 [[Population of Finland]] || Data on the age distribution of Finns || Age distribution is used, because susceptibility to diseases and their severity changes with age. The burden of disease and cases of illness are calculated for each age group, then summed. || | |||
|---- | |||
| Op_en7956 [[Drinking water chlorination efficiency]] || Log-decrease in pathogens due to chlorination, with different doses || The code on this page uses the data on the page and user-given dose for chlorine to calculate the case-specific decrease in pathogens due to chlorination. This is summed with log-decrease of other treatment and disinfection methods to produce the total log-decrease in pathogens from water treatment. || The model only takes into account any decrease in pathogens that happens in water treatment plants. Any further effect residual chlorine has after it enters the pipes is not included. | |||
|---- | |||
| Op_en7955 [[Drinking water disinfection efficiency]] || Log-decreases of pathogens due to ozonization and UV || If the user has chosen one or both of these disinfection methods, the decrease from the chosen one(s) is summed with the decrease due to chlorination and other treatment methods to produce the total log-decrease in pathogens from water treatment. || Currently the qualities of water, such as turbidity, are not taken into account in the efficiency of disinfection methods. The dose for both methods is also set. | |||
|---- | |||
| Op_en7954; [[Drinking water treatment efficiency]] || Log-decreases of pathogens due to different treatment methods. These methods are traditional cleaning, well working cleaning, enhanced cleaning, slow sand filtration, limestone filtration and active carbon filtration. || If the user has chosen one of multiple of these treatment methods, the decrease of pathogens they produce is summed with the decreases due to chlorination and other disinfection methods to produce the total log-decrease in pathogens from water treatment. || | |||
|---- | |||
| Op_en7953 [[Pathogen concentrations in raw water]] || The concentrations of pathogens of different raw water classes || If the user has chosen one of the raw water classes, the amounts of pathogens in that class and the total log-decrease of pathogens from treatment are used to calculate the amount of pathogens left in drinking water. If the user has given pathogen concentrations by hand, those values are used instead of the data on this page. || | |||
|---- | |||
| Op_en7947 [[Case burden of waterborne microbes]] || Case burdens of different pathogens, or how severe and long-lasting the diseases caused by them are || This data is used to calculate the health detriments caused by the pathogens in drinking water. || | |||
|---- | |||
| Op_en7957 [[Health impacts of waterborne microbes]] || Doesn't contain data, only code || These codes use water consumption given by the user, total decrease in pathogens and the pathogen concentrations in raw water to calculate the exposure to pathogens experienced by one individual, or how many of each pathogen an individual swallows daily with drinking water. || | |||
|---- | |||
| Op_en7948 [[ERF of waterborne microbes]] || Describes the exposure-response functions for the pathogens || These functions tell, how likely an individual is to get sick with different exposures. This and the exposure are used to estimate the cases of illness. || | |||
|---- | |||
| Op_en2261 [[HIA]] || Code that combines all of the above || The code on the page uses exposure, exposure-response functions and the case burdens to calculate the total burdens caused by pathogens in drinking water. From here it is also possible to calculate the number of people getting ill from drinking water. These are the final results of the model. || | |||
|} | |||
Table of decisions used in drawing plotly graphs. | |||
<t2b name="Decisions" index="Decision,Option,Variable,Cell,Change,Description" unit="-"> | |||
Adjust|Ground - none|RawConcentration|RawWaterClass: Ground water - surface water contamination|Remove|| | |||
Adjust|Ground - none|RawConcentration|||| | |||
</t2b> | |||
Well, this doesn't work at all. | |||
=== Calculations === | |||
==== Initiate model ==== | |||
<rcode name="waterguide" label="Initiate Water guide model" embed=0 graphics=1> | |||
# This is code Op_en6177/waterguide on page [[Water guide]] | |||
library(OpasnetUtils) | |||
library(ggplot2) | |||
openv.setN(1) | |||
# Create ovariables from user input data | |||
RawClass <- Ovariable("RawClass", data=data.frame(RawWaterClass = 'Surface water - low contamination', Result=1)) | |||
population <- Ovariable("population", data=data.frame(Result=10000)) | |||
Treatment <- Ovariable("Treatment", data=data.frame(TreatmentMethod=c('None'), Result=1)) | |||
ChlorineDose <- Ovariable("ChlorineDose", data=data.frame(Result=0)) | |||
) | |||
objects.latest("Op_en7957", code_name="exposure") | |||
#exposure <- EvalOutput(exposure) | |||
objects.latest("Op_en7947", code_name="caseburden") | |||
#case_burden <- EvalOutput(case_burden) | |||
incidence <- 1 # Number per 1py # Potentially everyone can get the disease. PAF is the fraction of this that actually occurs | |||
ERF <- Ovariable( | |||
"ERF", | |||
dependencies=data.frame(Name="ERF_micr",Ident="Op_en7948/ERF_micr2"), | |||
formula = function(...) return(ERF_micr) | |||
) | |||
) | ) | ||
objects.latest("Op_en2261", code_name="BoDattr2") # [[Health impact assessment]] BoDattr | |||
BoDattr <- EvalOutput(BoDattr, verbose=TRUE) | |||
oprint(summary(BoDattr, marginals=c("Response","Exposure_agent"))) | |||
objects.store(list=setdiff(ls(), "wiki_username")) | |||
cat("The whole model stored (", setdiff(ls(), "wiki_username"), ").\n") | |||
ggplot( | |||
oapply(unkeep(BoDattr, sources=TRUE), NULL, mean, "Iter")@output, | |||
aes(x=Exposure_agent, weight=BoDattrResult, fill=Response)) + | |||
geom_bar() + | |||
coord_flip() + | |||
labs( | |||
title="Disease burden of waterborne microbes", | |||
subtitle=paste("in a population of", sum(result(population))), | |||
y = "Disease burden (DALY/incident)" | |||
) | |||
if(FALSE) { | |||
######################## Diagnostics | |||
objects.latest("Op_en6007", code_name="diagnostics") | |||
oprint(showLoctable()) | |||
oprint(showind()) | |||
################ Insight network | |||
gr <- scrape(type="assessment") | |||
objects.latest("Op_en3861", "makeGraph") # [[Insight network]] | |||
gr <- makeGraph(gr) | |||
#export_graph(gr, "ruori.svg") | |||
#render_graph(gr) | |||
} | |||
</rcode> | |||
==== Plotly test ==== | |||
<rcode label="Code does not work in Opasnet"> | |||
library(OpasnetUtils) | |||
library(ggplot2) | |||
library(plotly) | |||
objects.latest("Op_en6177", code_name="waterguide") # [[Water guide]] fetch the whole model | |||
total_population <- 10000 | |||
# Create ovariables from user input data | |||
RawClass <- Ovariable("RawClass", data=data.frame(RawWaterClass = "Surface water - high contamination", Result=1)) | |||
Treatment <- Ovariable("Treatment", data=data.frame(TreatmentMethod=c("None"), Result=1)) | |||
RawConsumption <- Ovariable("RawConsumption", data=data.frame(Result=0.8)) | |||
# divide the given population size to different age groups based on age distribution of all of Finland | |||
population <- Ovariable("population", | |||
dependencies=data.frame( | |||
Name=c("total_population") | |||
), | |||
formula=function (...) { | |||
population2 <- Ovariable( | |||
"population2", | |||
ddata="Op_en2949", subset="Population" | |||
) | |||
population2 <- EvalOutput(population2) | |||
population <- population2/oapply(population2, cols = "Age", FUN=sum) * total_population | |||
return(population) | |||
} | |||
) | |||
# create data | |||
aval <- list() | |||
for(step in seq(0, 1, 0.1)){ | |||
ChlorineDose <- Ovariable("ChlorineDose", data=data.frame(Result=step)) | |||
BoDattr <- EvalOutput(BoDattr, verbose=FALSE, forceEval=TRUE) | |||
plotdata <- oapply(unkeep(BoDattr, sources=TRUE), NULL, mean, "Iter") | |||
plotdata <- oapply(plotdata, FUN=sum, cols="Age") | |||
# creates a loist of lists, each list containing the data for the grapsh with one of the chlorine doses | |||
aval[[((step+0.1)*10)]] <-list(visible = FALSE, | |||
name = paste0('dose = ', step), | |||
x=plotdata@output$BoDattrResult, | |||
y=plotdata@output$Exposure_agent, | |||
response=plotdata@output$Response) | |||
} | |||
aval[1][[1]]$visible = TRUE # the figure with clorine dose 0 is set to visible | |||
# this bit is to move the first dose to be the last, because the figure is for some reason | |||
# draws the last to be the first, and I don't understand, but like this they're in the right | |||
# order in the picture | |||
aval <- aval[c(2:length(aval), 1)] | |||
# create steps and plot all traces | |||
steps_micro <- list() | |||
p <- plot_ly(type = 'bar', orientation = "h") | |||
for (i in 1:length(aval)) { | |||
p <- add_trace(p, # add bars to bar graph p | |||
x=aval[i][[1]]$x, # x (DALYs) on x | |||
y=aval[i][[1]]$y, # y (exposure agent) on y | |||
visible = aval[i][[1]]$visible, # visibility was set earlier | |||
text = aval[i][[1]]$response, | |||
type="bar", | |||
hoverinfo = "text+x", # what the info shown when mouse on top of it says | |||
orientation="h") | |||
step <- list(args = list('visible', rep(FALSE, length(aval))), # set all steps to not visible | |||
method = 'restyle', | |||
label= (i-1)/10) # Sets the text label to appear on the slider | |||
step$args[[2]][i] = TRUE # set data on i step to be visible when step i is chosen | |||
steps_micro[[i]] = step # make a list with all the steps | |||
} | |||
# add slider control to plot | |||
p <- p %>% | |||
layout(sliders = list(list(active = 0, #Determines which button (by index starting from 0) is considered active. | |||
currentvalue = list(prefix = "Chlorine dose (mg/l): "), # the text under the graph that tells what the slider values mean | |||
steps = steps_micro)), | |||
barmode = 'stack', # stacked plot instead of each disease its own bar | |||
title = paste('Burdens of disease of drinking water in a population of', total_population), | |||
xaxis = list(title ="DALY"), | |||
yaxis = list(title ="")) | |||
p | |||
</rcode> | |||
==== Another plotly test ==== | |||
<rcode label="Run on own computer"> | |||
library(OpasnetUtils) | |||
library(ggplot2) | |||
library(plotly) | |||
# | objects.latest("Op_en6177", code_name="waterguide") # [[Water guide]] fetch the whole model | ||
total_population <- 10000 | |||
# Create ovariables from user input data | |||
RawClass <- Ovariable("RawClass", data=data.frame(RawWaterClass = "Surface water - high contamination", Result=1)) | |||
Treatment <- Ovariable("Treatment", data=data.frame(TreatmentMethod=c("None"), Result=1)) | |||
ChlorineDose <- Ovariable("ChlorineDose", data=data.frame(Chlorine=c("None","Low","High"), Result=c(0, 0.5, 1))) | |||
RawConsumption <- Ovariable("RawConsumption", data=data.frame(Result=0.8)) | |||
# divide the given population size to different age groups based on age distribution of all of Finland | |||
population <- Ovariable( | |||
"population", | |||
dependencies=data.frame( | |||
Name=c("total_population") | |||
), | |||
formula=function (...) { | |||
population2 <- Ovariable( | |||
"population2", | |||
ddata="Op_en2949", subset="Population" | |||
) | |||
population2 <- EvalOutput(population2) | |||
population <- population2/oapply(population2, cols = "Age", FUN=sum) * total_population | |||
return(population) | |||
} | |||
) | |||
# | BoDattr <- EvalOutput(BoDattr)#, verbose=FALSE), forceEval=TRUE) | ||
plotdata <- oapply(unkeep(BoDattr, sources=TRUE), NULL, mean, "Iter") | |||
plotdata <- oapply(plotdata, FUN=sum, cols="Age") | |||
# | plot_ly(plotdata@output, x = ~BoDattrResult, y = ~Exposure_agent, | ||
type = 'bar', | |||
orientation = "h", # bars vertically or horizontally | |||
color = ~Response, # what to use as a basis for colored groups | |||
colors=c("sandybrown", "gray50", "darkorchid1", "aquamarine1", "firebrick1", | |||
"cornflowerblue", "gold1", "chocolate3")) %>% # the colors used | |||
# in the order that the diseases appear in the table. not level order. table order. | |||
layout(barmode = 'stack', # stacked plot instead of each disease its own bar | |||
title = paste('Burdens of disease of drinking water in a population of', total_population), | |||
xaxis = list(title ="DALY"), | |||
yaxis = list(title ="")) | |||
steps <- list() | |||
pl <- plot_ly() | |||
for(i in levels(ChlorineDose$Chlorine)) { # add data for each slider step (=each chlorine level) | |||
tmp <- plotdata[plotdata$Chlorine==i , ] # Slice rows for each chlorine level at a time | |||
pl <- add_trace( # Add a separate dataset for each step | |||
pl, | |||
x = tmp$Exposure_agent, | |||
y = tmp$BoDattrResult, | |||
name = tmp$Response, | |||
type = "bar", | |||
# orientation = "h", | |||
visible = i==levels(ChlorineDose$Chlorine)[1] # Make only the first dataset visible by default SOMETHING STRANGE HERE | |||
) | |||
step <- list( | |||
args = list('visible', i==levels(ChlorineDose$Chlorine)), # Make all datasets invisible in this step except this one | |||
label = i, | |||
value = match(i,levels(ChlorineDose$Chlorine)), | |||
method = 'restyle' # I suppose this means that the graph is retyled when the step changes | |||
) | |||
steps[[match(i,levels(ChlorineDose$Chlorine))]] <- step | |||
} | |||
pl %>% layout( | |||
sliders = list(list( | |||
active = 0, # Make the first step active by default (counting starts from 0 in plotly) | |||
currentvalue = list(prefix = "Chlorine: "), | |||
steps = steps | |||
)), | |||
barmode="stack" | |||
) | |||
# calculate the number of cases | |||
cases <- BoDattr/case_burden | |||
cases <- oapply(unkeep(cases, sources=TRUE), NULL, mean, "Iter") | |||
cases <- oapply(cases, FUN=sum, cols="Age") | |||
plot_ly(cases@output, x = ~Result, y = ~Exposure_agent, | |||
type = 'bar', orientation = 'h', | |||
color = ~Response, width = 3, | |||
colors=c("sandybrown", "gray50", "darkorchid1", "aquamarine1", "firebrick1", | |||
"cornflowerblue", "gold1", "chocolate3")) %>% | |||
layout(barmode = 'stack', | |||
title = paste('Cases of disease from drinking water in a population of', total_population), | |||
xaxis = list(title ="tautitapaukset"), | |||
yaxis = list(title ="")) | |||
# | ############### | ||
doses <- c("None","Low","High") | |||
plotdata <- data.frame( | |||
Exposure_agent = rep(c("Noro","Ecoli"),each=3), | |||
Response = c("Gastroenteritis","Fever"), | |||
Chlorine = doses, | |||
BoDattrResult = c(3,2,1,6,5,4) | |||
) | |||
steps <- list() | |||
pl <- plot_ly() | |||
for(i in doses) { # add data for each slider step (=each chlorine level) | |||
tmp <- plotdata[plotdata$Chlorine==i , ] # Slice rows for each chlorine level at a time | |||
pl <- add_trace( # Add a separate dataset for each step | |||
pl, | |||
x = tmp$Exposure_agent, | |||
y = tmp$BoDattrResult, | |||
color = tmp$Response, | |||
name = tmp$Chlorine, | |||
type = "bar", | |||
# orientation = "h", | |||
visible = i==doses[1] # Make only the first dataset visible by default SOMETHING STRANGE HERE | |||
) | |||
step <- list( | |||
args = list('visible', i==dose), # Make all datasets invisible in this step except this one | |||
label = i, | |||
value = match(i,doses), | |||
method = 'restyle' # I suppose this means that the graph is retyled when the step changes | |||
) | |||
steps[[match(i,doses)]] <- step | |||
} | |||
pl %>% layout( | |||
sliders = list(list( | |||
active = 0, # Make the first step active by default (counting starts from 0 in plotly) | |||
currentvalue = list(prefix = "Chlorine: "), | |||
steps = steps | |||
)), | |||
barmode="stack" | |||
) | |||
</rcode> | |||
==== Plotly with two sliders ==== | |||
<rcode label="Run on own computer" name="plotlydata"> | |||
# This is code Op_en6177/plotlydata on page [[Water guide]] | |||
library(OpasnetUtils) | |||
library(ggplot2) | |||
library(plotly) | |||
openv.setN(100) | |||
objects.latest("Op_en6177", code_name="waterguide") # [[Water guide]] fetch the whole model | |||
total_population <- 10000 | |||
# Create ovariables | |||
RawConsumption <- Ovariable("RawConsumption", data=data.frame(Result=0.8)) | |||
# divide the given population size to different age groups based on age distribution of all of Finland | |||
population <- Ovariable("population", | |||
dependencies=data.frame( | |||
Name=c("total_population") | |||
), | |||
formula=function (...) { | |||
population2 <- Ovariable( | |||
"population2", | |||
ddata="Op_en2949", subset="Population" | |||
) | |||
population2 <- EvalOutput(population2) | |||
population <- population2/oapply(population2, cols = "Age", FUN=sum) * | |||
total_population | |||
return(population) | |||
} | |||
) | |||
ChlorineDose <- Ovariable("ChlorineDose", | |||
data=data.frame(ClDose=seq(0,0.5,0.1), | |||
Result=seq(0,0.5,0.1))) | |||
# Making the dataframes used in plots---- | |||
treatment <- list(c("None"), | |||
c("Slow sand filtration"), | |||
c("Slow sand filtration"), | |||
c("None"), | |||
c("None"), | |||
c("Slow sand filtration"), | |||
c("Slow sand filtration", "Active carbon filtration")) | |||
uvct <- c(0,0,10,0,10,10,10) | |||
rawclass <- c(rep("Ground water - clean", 3), rep("Surface water - high contamination", 4)) | |||
labels <- c("Ground - none", "Ground - sand", "Ground - sand+uv", "Surface - none", | |||
"Surface - uv", "Surface - sand+uv", "Surface - sand+carbon+uv") | |||
plotdata_illness <- data.frame(matrix(ncol=5, nrow=0)) | |||
colnames(plotdata_illness) <- c("Response", "ClDose", "RawWaterClass", "BoDattrResult", "label") | |||
plotdata_age <- data.frame(matrix(ncol=5, nrow=0)) | |||
colnames(plotdata_age) <- c("ClDose", "RawWaterClass", "Age", "BoDattrResult", "label") | |||
plotdata_path <- data.frame(matrix(ncol=5, nrow=0)) | |||
colnames(plotdata_path) <- c("Exposure_agent", "ClDose", "RawWaterClass", "BoDattrResult", "label") | |||
plotdata_path_gastro <- data.frame(matrix(ncol=6, nrow=0)) | |||
colnames(plotdata_path_gastro) <- c("Exposure_agent", "Response", "ClDose", "RawWaterClass", "BoDattrResult", "label") | |||
for(i in 1:length(treatment)){ | |||
RawClass <- Ovariable("RawClass", data=data.frame(RawWaterClass = rawclass[i], Result=1)) | |||
Treatment <- Ovariable("Treatment", data=data.frame(TreatmentMethod=treatment[[i]], Result=1)) | |||
UVCT <- Ovariable("UVCT", data=data.frame(Result=uvct[i])) | |||
BoDattr <- EvalOutput(BoDattr, forceEval=TRUE) | |||
plotdata <- oapply(BoDattr, c("Response","Exposure_agent","ClDose","RawWaterClass", "Iter", "Age"), sum) | |||
plotdata <- oapply(plotdata, FUN=mean, cols="Iter") | |||
cases <- BoDattr/case_burden | |||
casedata <- oapply(cases, c("Response","Exposure_agent","ClDose","RawWaterClass", "Iter", "Age"), sum) | |||
casedata <- oapply(casedata, FUN=mean, cols="Iter") | |||
plotdata_illness <- rbind(plotdata_illness, data.frame( | |||
oapply(plotdata, FUN=sum, cols=c("Exposure_agent", "Age"))@output, | |||
label = labels[i])) | |||
plotdata_age <- rbind(plotdata_age, data.frame( | |||
oapply(plotdata, FUN=sum, cols=c("Exposure_agent", "Response"))@output, | |||
label = labels[i])) | |||
plotdata_path <- rbind(plotdata_path, data.frame( | |||
oapply(plotdata, FUN=sum, cols=c("Response", "Age"))@output, | |||
label = labels[i])) | |||
plotdata_path_gastro <- rbind(plotdata_path_gastro, data.frame( | |||
oapply(casedata, FUN=sum, cols=c("Age"))@output, | |||
label = labels[i])) | |||
} | |||
# SUOMENNOKSET, JOS HALUTAAN SUOMENKIELISET KUVAT | |||
levels(plotdata_illness$Response) <- c("kliininen Guillian-Barrén oireyhtymä", "kuolema", | |||
"loppuvaiheen munuaistauti", "gastroenteriitti", | |||
"hemolyyttis-ureeminen oireyhtymä", "suolistoverenvuoto", | |||
"reaktiivinen niveltulehdus", | |||
"Guillian-Barrén jäännösoireet") | |||
levels(plotdata_path$Exposure_agent) <- c("kampylobakteeri", "kryptosporidium", "E. coli O157:H7", | |||
"giardia", "norovirus", "rotavirus") | |||
levels(plotdata_illness$label) <- c("pohjavesi - ei puhdistusta", "pohjavesi - hiekka", | |||
"pohjavesi - hiekka+uv", "pintavesi - ei puhdistusta", | |||
"pintavesi - uv", "pintavesi - hiekka+uv", | |||
"pintavesi - hiekka+aktiivihiili+uv") | |||
levels(plotdata_path$label) <- c("pohjavesi - ei puhdistusta", "pohjavesi - hiekka", | |||
"pohjavesi - hiekka+uv", "pintavesi - ei puhdistusta", "pintavesi - uv", | |||
"pintavesi - hiekka+uv", "pintavesi - hiekka+aktiivihiili+uv") | |||
levels(plotdata_age$label) <- c("pohjavesi - ei puhdistusta", "pohjavesi - hiekka", | |||
"pohjavesi - hiekka+uv", "pintavesi - ei puhdistusta", "pintavesi - uv", | |||
"pintavesi - hiekka+uv", "pintavesi - hiekka+aktiivihiili+uv") | |||
### DALYS CAUSED BY DISEASE ---- | |||
illness_data <- plotdata_illness | |||
colnames(illness_data) <- c("response", "cldose", "rawclass", "result", "label") | |||
illness_data$visible <- F | |||
illness_data$visible[illness_data$label==levels(illness_data$label)[1]] = T # clean ground water visible | |||
# | # create steps and plot all traces | ||
buttons_water1 <- list() | |||
illness_plot <- plot_ly() | |||
for (i in 1:length(treatment)) { | |||
illness_plot <- add_trace(illness_plot, # add traces to illness_plot | |||
type = "scatter", | |||
x=illness_data$result[illness_data$label==levels(illness_data$label)[i]], # x (BoD) on x | |||
y=illness_data$response[illness_data$label==levels(illness_data$label)[i]], # y (exposure agent) on y | |||
visible = illness_data$visible[illness_data$label==levels(illness_data$label)[i]], # is this trace visible | |||
# text = aval$pathogen[aval$rawclass==levels(aval$rawclass)[i]], # text to be used as a label | |||
mode="markers", | |||
marker = list(size=10, # marker size | |||
colorscale = list(list(0, 'rgba(200,200,200,0.2)'), list(1, 'rgba(0,181,204,1)')), # sets the colors used when color=0 and color=1 | |||
color = c(rep(1, 8), # the first 17 markers use color 1 | |||
rep(0, 8*5))), | |||
# opacity = c(rep(1, 8), # the first 17 markers use color 1 | |||
# rep(0.05, 8*5))), # the rest use color 0 | |||
hoverinfo = "x", # what is the info shown when mouse on top of marker | |||
showlegend=F) | |||
bw <- list(args = list('visible', rep(FALSE, length(treatment))), # set all steps to not visible | |||
method = 'restyle', | |||
label= levels(illness_data$label)[i]) # Sets the text label to appear on the menu | |||
bw$args[[2]][i] = TRUE # set data on i+1 step to be visible when step i is chosen | |||
buttons_water1[[i]] = bw # make a list with all the steps | |||
} | |||
steps_cl1 <- list(list(args = list('marker.color', # marker color is changed | |||
array(c(rep(1, 8), rep(0, 8*5)), dim=c(1, 102))), # these are the new markers with color 1 and 0 | |||
method = 'restyle', # restyling the markers | |||
label = "0"), # label on the slider | |||
list(args = list('marker.color', | |||
array(c(rep(0, 8), rep(1, 8), rep(0, 8*4)), dim=c(1, 102))), | |||
method = 'restyle', | |||
label = "0.1"), | |||
list(args = list('marker.color', | |||
array(c(rep(0, 8*2), rep(1, 8), rep(0, 8*3)), dim=c(1, 102))), | |||
method = 'restyle', | |||
label = "0.2"), | |||
list(args = list('marker.color', | |||
array(c(rep(0, 8*3), rep(1, 8), rep(0, 8*2)), dim=c(1, 102))), | |||
method = 'restyle', | |||
label = "0.3"), | |||
list(args = list('marker.color', | |||
array(c(rep(0, 8*4), rep(1, 8), rep(0, 8)), dim=c(1, 102))), | |||
method = 'restyle', | |||
label = "0.4"), | |||
list(args = list('marker.color', | |||
array(c(rep(0, 8*5), rep(1, 8)), dim=c(1, 102))), | |||
method = 'restyle', | |||
label = "0.5") | |||
) | |||
# add slider control to plot | |||
illness_plot <- illness_plot %>% | |||
layout(sliders = list(list(active = 0, #Determines which button (by index starting from 0) is active. | |||
currentvalue = list(prefix = "Klooriannos mg/l:"), #suomeksi | |||
# currentvalue = list(prefix = "Chlorine dose: "), # in English | |||
# the text under the graph that tells what the slider values mean | |||
steps = steps_cl1)), | |||
updatemenus = list(list(active = 0, | |||
buttons = buttons_water1, | |||
y = -0.15, | |||
x = -0.1, | |||
direction = "up")), | |||
# title = paste('Burdens of disease of drinking water in a population of', total_population), # in English | |||
title = paste("Juomaveden aiheuttama tautitaakka", total_population, "hengen populaatiossa"), # suomeksi | |||
xaxis = list(title ="DALY"), | |||
yaxis = list(title ="")) | |||
illness_plot | |||
# | |||
### DALYS BY AGE GROUP ---- | |||
# a data frame with the relevant columns | |||
age_data <- plotdata_age | |||
colnames(age_data) <- c("cldose", "rawclass", "age", "result", "label") | |||
age_data$visible <- F | |||
age_data$visible[age_data$label==levels(age_data$label)[1]] = T # ground water + none visible | |||
# ordering the age groups to be in the right order | |||
age_data$age <- factor(age_data$age, levels(age_data$age)[c(1,4,2,3,5,6)]) | |||
age_data <- age_data[order(as.numeric(age_data$age)),] | |||
# create steps and plot all traces | |||
buttons_water2 <- list() | |||
age_plot <- plot_ly() | |||
for (i in 1:length(treatment)) { | |||
age_plot <- add_trace(age_plot, # add traces to age_plot | |||
type = "scatter", | |||
x=age_data$result[age_data$label==levels(age_data$label)[i]], # x (BoD) on x | |||
y=age_data$age[age_data$label==levels(age_data$label)[i]], # y (exposure agent) on y | |||
visible = age_data$visible[age_data$label==levels(age_data$label)[i]], # is this trace visible | |||
# text = aval$pathogen[aval$rawclass==levels(aval$rawclass)[i]], # text to be used as a label | |||
mode="markers", | |||
marker = list(size=10, # marker size | |||
colorscale = list(list(0, 'rgba(200,200,200,0.2)'), list(1, 'rgba(0,181,204,1)')), # sets the colors used when color=0 and color=1 | |||
color = c(rep(c(1,0,0,0,0,0), 6))), | |||
hoverinfo = "x", # what is the info shown when mouse on top of marker | |||
showlegend=F) | |||
bw <- list(args = list('visible', rep(FALSE, length(treatment))), # set all steps to not visible | |||
method = 'restyle', | |||
label= levels(age_data$label)[i]) # Sets the text label to appear on the menu | |||
bw$args[[2]][i] = TRUE # set data on i+1 step to be visible when step i is chosen | |||
buttons_water2[[i]] = bw # make a list with all the steps | |||
} | |||
steps_cl2 <- list(list(args = list('marker.color', # marker color is changed | |||
array(c(rep(c(1,0,0,0,0,0), 6)), dim=c(1, 36))), # these are the new markers with color 1 and 0 | |||
method = 'restyle', # restyling the markers | |||
label = "0"), # label on the slider | |||
list(args = list('marker.color', | |||
array(c(rep(c(0,1,0,0,0,0), 6)), dim=c(1, 36))), | |||
method = 'restyle', | |||
label = "0.1"), | |||
list(args = list('marker.color', | |||
array(c(rep(c(0,0,1,0,0,0), 6)), dim=c(1, 36))), | |||
method = 'restyle', | |||
label = "0.2"), | |||
list(args = list('marker.color', | |||
array(c(rep(c(0,0,0,1,0,0), 6)), dim=c(1, 36))), | |||
method = 'restyle', | |||
label = "0.3"), | |||
list(args = list('marker.color', | |||
array(c(rep(c(0,0,0,0,1,0), 6)), dim=c(1, 36))), | |||
method = 'restyle', | |||
label = "0.4"), | |||
list(args = list('marker.color', | |||
array(c(rep(c(0,0,0,0,0,1), 6)), dim=c(1, 36))), | |||
method = 'restyle', | |||
label = "0.5") | |||
) | |||
######## | # add slider control to plot | ||
age_plot <- age_plot %>% | |||
layout(sliders = list(list(active = 0, #Determines which button (by index starting from 0) is active. | |||
currentvalue = list(prefix = "Klooriannos mg/l:"), #suomeksi | |||
# currentvalue = list(prefix = "Chlorine dose: "), #in English | |||
steps = steps_cl2)), | |||
updatemenus = list(list(active = 0, | |||
y = -0.15, | |||
x = -0.1, | |||
direction = "up", | |||
buttons = buttons_water2)), | |||
title = paste("Juomaveden aiheuttama tautitaakka", total_population, "hengen populaatiossa"), # suomeksi | |||
# title = paste('Burdens of disease of drinking water in a population of', total_population), # in English | |||
xaxis = list(title ="DALY"), | |||
yaxis = list(title ="")) | |||
age_plot | |||
# | |||
# | ### DALYS CAUSED BY PATHOGEN ---- | ||
# | # a data frame with the relevant columns | ||
pathogen_data <- plotdata_path | |||
colnames(pathogen_data) <- c("pathogen", "cldose", "rawclass", "result", "label") | |||
# | pathogen_data$visible <- F | ||
pathogen_data$visible[pathogen_data$label==levels(pathogen_data$label)[1]] = T # ground water + none visible | |||
# | #levels(pathogen_data$rawclass) <- c("clean", "almost", "high", "low", "medium") | ||
# | #pathogen_data$rawclass <- factor(pathogen_data$rawclass, levels(pathogen_data$rawclass)[c(2,4,5,3,1)]) | ||
#pathogen_data$visible[pathogen_data$rawclass==levels(pathogen_data$rawclass)[1]] = T # clean ground water visible | |||
# | # create steps and plot all traces | ||
# | buttons_water3 <- list() | ||
pathogen_plot <- plot_ly() | |||
for (i in 1:length(treatment)) { | |||
pathogen_plot <- add_trace(pathogen_plot, # add traces to illness_plot | |||
type = "scatter", | |||
x=pathogen_data$result[pathogen_data$label==levels(pathogen_data$label)[i]], # x (BoD) on x | |||
y=pathogen_data$pathogen[pathogen_data$label==levels(pathogen_data$label)[i]], # y (exposure agent) on y | |||
visible = pathogen_data$visible[pathogen_data$label==levels(pathogen_data$label)[i]], # is this trace visible | |||
mode="markers", | |||
marker = list(size=10, # marker size | |||
colorscale = list(list(0, 'rgba(200,200,200,0.2)'), list(1, 'rgba(0,181,204,1)')), # sets the colors used when color=0 and color=1 | |||
color = c(rep(1, 6), # the first 17 markers use color 1 | |||
rep(0, 6*5))), # the rest use color 0 | |||
hoverinfo = "x", # what is the info shown when mouse on top of marker | |||
showlegend=F) | |||
bw <- list(args = list('visible', rep(FALSE, length(treatment))), # set all steps to not visible | |||
method = 'restyle', | |||
label= levels(pathogen_data$label)[i]) # Sets the text label to appear on the menu | |||
bw$args[[2]][i] = TRUE # set data on i+1 step to be visible when step i is chosen | |||
buttons_water3[[i]] = bw # make a list with all the steps | |||
} | |||
steps_cl3 <- list(list(args = list('marker.color', # marker color is changed | |||
array(c(rep(1, 6), rep(0, 6*5)), dim=c(1, 36))), # these are the new markers with color 1 and 0 | |||
method = 'restyle', # restyling the markers | |||
label = "0"), # label on the slider | |||
list(args = list('marker.color', | |||
array(c(rep(0, 6), rep(1, 6), rep(0, 6*4)), dim=c(1, 36))), | |||
method = 'restyle', | |||
label = "0.1"), | |||
list(args = list('marker.color', | |||
array(c(rep(0, 6*2), rep(1, 6), rep(0, 6*3)), dim=c(1, 36))), | |||
method = 'restyle', | |||
label = "0.2"), | |||
list(args = list('marker.color', | |||
array(c(rep(0, 6*3), rep(1, 6), rep(0, 6*2)), dim=c(1, 36))), | |||
method = 'restyle', | |||
label = "0.3"), | |||
list(args = list('marker.color', | |||
array(c(rep(0, 6*4), rep(1, 6), rep(0, 6)), dim=c(1, 36))), | |||
method = 'restyle', | |||
label = "0.4"), | |||
list(args = list('marker.color', | |||
array(c(rep(0, 6*5), rep(1, 6)), dim=c(1, 36))), | |||
method = 'restyle', | |||
label = "0.5") | |||
) | |||
# add slider control to plot | |||
pathogen_plot <- pathogen_plot %>% | |||
layout(sliders = list(list(active = 0, #Determines which button (by index starting from 0) is active. | |||
currentvalue = list(prefix = "Klooriannos mg/l:"), # suomeksi | |||
# currentvalue = list(prefix = "Chlorine dose: "), # in English # the text under the graph that tells what the slider values mean | |||
steps = steps_cl3)), | |||
updatemenus = list(list(active = 0, | |||
y = -0.15, | |||
x = -0.1, | |||
direction = "up", | |||
buttons = buttons_water3)), | |||
title = paste("Juomaveden aiheuttama tautitaakka", total_population, "hengen populaatiossa"), # suomeksi | |||
# title = paste('Burdens of disease of drinking water in a population of', total_population), # in English | |||
xaxis = list(title ="DALY"), | |||
yaxis = list(title ="")) | |||
pathogen_plot | |||
### DALYs BY PATHOGEN, ONLY GASTROENTERITIS ---- | |||
# a data frame with the relevant columns | |||
pathogen_gastro_data <- plotdata_path_gastro[plotdata_path_gastro$Response == "gastroenteritis",] | |||
colnames(pathogen_gastro_data) <- c("response", "pathogen", "cldose", "rawclass", "result", "label") | |||
pathogen_gastro_data$visible <- F | |||
pathogen_gastro_data$visible[pathogen_gastro_data$label==levels(pathogen_gastro_data$label)[1]] = T # ground water + none visible | |||
#levels(pathogen_data$rawclass) <- c("clean", "almost", "high", "low", "medium") | |||
#pathogen_data$rawclass <- factor(pathogen_data$rawclass, levels(pathogen_data$rawclass)[c(2,4,5,3,1)]) | |||
#pathogen_data$visible[pathogen_data$rawclass==levels(pathogen_data$rawclass)[1]] = T # clean ground water visible | |||
''' | # create steps and plot all traces | ||
buttons_water3 <- list() | |||
path_gastro_plot <- plot_ly() | |||
for (i in 1:length(treatment)) { | |||
path_gastro_plot <- add_trace(path_gastro_plot, # add traces to illness_plot | |||
type = "scatter", | |||
x=pathogen_gastro_data$result[pathogen_gastro_data$label==levels(pathogen_gastro_data$label)[i]], # x (BoD) on x | |||
y=pathogen_gastro_data$pathogen[pathogen_gastro_data$label==levels(pathogen_gastro_data$label)[i]], # y (exposure agent) on y | |||
visible = pathogen_gastro_data$visible[pathogen_gastro_data$label==levels(pathogen_gastro_data$label)[i]], # is this trace visible | |||
mode="markers", | |||
marker = list(size=10, # marker size | |||
colorscale = list(list(0, 'rgba(200,200,200,0.2)'), list(1, 'rgba(0,181,204,1)')), # sets the colors used when color=0 and color=1 | |||
color = c(rep(1, 6), # the first 17 markers use color 1 | |||
rep(0, 6*5))), # the rest use color 0 | |||
hoverinfo = "x", # what is the info shown when mouse on top of marker | |||
showlegend=F) | |||
bw <- list(args = list('visible', rep(FALSE, length(treatment))), # set all steps to not visible | |||
method = 'restyle', | |||
label= levels(pathogen_gastro_data$label)[i]) # Sets the text label to appear on the menu | |||
bw$args[[2]][i] = TRUE # set data on i+1 step to be visible when step i is chosen | |||
buttons_water3[[i]] = bw # make a list with all the steps | |||
} | |||
'''4. | steps_cl3 <- list(list(args = list('marker.color', # marker color is changed | ||
array(c(rep(1, 6), rep(0, 6*5)), dim=c(1, 36))), # these are the new markers with color 1 and 0 | |||
method = 'restyle', # restyling the markers | |||
label = "0"), # label on the slider | |||
list(args = list('marker.color', | |||
array(c(rep(0, 6), rep(1, 6), rep(0, 6*4)), dim=c(1, 36))), | |||
method = 'restyle', | |||
label = "0.1"), | |||
list(args = list('marker.color', | |||
array(c(rep(0, 6*2), rep(1, 6), rep(0, 6*3)), dim=c(1, 36))), | |||
method = 'restyle', | |||
label = "0.2"), | |||
list(args = list('marker.color', | |||
array(c(rep(0, 6*3), rep(1, 6), rep(0, 6*2)), dim=c(1, 36))), | |||
method = 'restyle', | |||
label = "0.3"), | |||
list(args = list('marker.color', | |||
array(c(rep(0, 6*4), rep(1, 6), rep(0, 6)), dim=c(1, 36))), | |||
method = 'restyle', | |||
label = "0.4"), | |||
list(args = list('marker.color', | |||
array(c(rep(0, 6*5), rep(1, 6)), dim=c(1, 36))), | |||
method = 'restyle', | |||
label = "0.5") | |||
) | |||
# Add marker line | |||
line <- list( | |||
type = "line", | |||
line = list(color = "red"), | |||
xref = "x", | |||
yref = "y" | |||
) | |||
lines <- list() | |||
line[c("x0", "x1")] <- 1 | |||
line["y0"] <- -0.5 | |||
line["y1"] <- 5.5 | |||
lines <- c(lines, list(line)) | |||
# add slider control to plot | |||
path_gastro_plot <- path_gastro_plot %>% | |||
layout(sliders = list(list(active = 0, #Determines which button (by index starting from 0) is active. | |||
currentvalue = list(prefix = "Klooriannos mg/l:"), # suomeksi | |||
# currentvalue = list(prefix = "Chlorine dose: "), # in English the text under the graph that tells what the slider values mean | |||
steps = steps_cl3)), | |||
updatemenus = list(list(active = 0, | |||
y = -0.15, | |||
x = -0.1, | |||
direction = "up", | |||
buttons = buttons_water3)), | |||
shapes = lines, | |||
title = paste("Juomaveden päivässä aiheuttamat suolistotautitapaukset", total_population, "hengen populaatiossa"), # suomeksi | |||
# title = paste('Number of gastroenteritis cases caused daily by drinking water in a population of', total_population), # in English | |||
xaxis = list(title ="Tautitapaukset"), | |||
yaxis = list(title ="")) | |||
path_gastro_plot | |||
# | |||
#### STEPS TO CHANGE SIZE ---- | |||
#steps_ch <- list(list(args = list('marker.size', array(c(rep(13, 17), rep(3, 17*5)), dim=c(1, 102))), | |||
# method = 'restyle', | |||
# label = "0"), | |||
# list(args = list('marker.size', array(c(rep(3, 17), rep(13, 17), rep(3, 17*4)), dim=c(1, 102))), | |||
# method = 'restyle', | |||
# label = "0.1"), | |||
# list(args = list('marker.size', array(c(rep(3, 17*2), rep(13, 17), rep(3, 17*3)), dim=c(1, 102))), | |||
# method = 'restyle', | |||
# label = "0.2"), | |||
# list(args = list('marker.size', array(c(rep(3, 17*3), rep(13, 17), rep(3, 17*2)), dim=c(1, 102))), | |||
# method = 'restyle', | |||
# label = "0.3"), | |||
# list(args = list('marker.size', array(c(rep(3, 17*4), rep(13, 17), rep(3, 17)), dim=c(1, 102))), | |||
# method = 'restyle', | |||
# label = "0.4"), | |||
# list(args = list('marker.size', array(c(rep(3, 17*5), rep(13, 17)), dim=c(1, 102))), | |||
# method = 'restyle', | |||
# label = "0.5") | |||
#) | |||
# Add this to the plot, if you use the size: | |||
# marker = list(size = c(rep(13, 17), rep(3, 17*5)), | |||
# color = 'rgba(128,0,128,0.7)'), | |||
# PUSH INDICATOR GRAPH ---- | |||
pushIndicatorGraph(illness_plot, 188) | |||
pushIndicatorGraph(age_plot, 189) | |||
pushIndicatorGraph(pathogen_plot, 190) | |||
</rcode> | |||
== See also == | == See also == | ||
Line 642: | Line 1,093: | ||
* [[:op_fi:Mikrobien määrä juomavedessä]] | * [[:op_fi:Mikrobien määrä juomavedessä]] | ||
* [[:op_fi:Mikrobien määrä pohjavedessä]] | * [[:op_fi:Mikrobien määrä pohjavedessä]] | ||
*[[Bathing water guide]] | |||
* WHO: [https://apps.who.int/iris/bitstream/handle/10665/246195/9789241565370-eng.pdf?sequence=1 Quantitative Microbial Risk Assessment: Application for Water Safety Management] | |||
*http://www.fao.org/docrep/006/y4666e/y4666e06.htm#TopOfPage | *http://www.fao.org/docrep/006/y4666e/y4666e06.htm#TopOfPage | ||
*http://wiki.camra.msu.edu/index.php?title=Main_Page | *http://wiki.camra.msu.edu/index.php?title=Main_Page | ||
*http://www.medicina.fi/index.php?option=com_content&view=article&id=48&Itemid=56#I | *http://www.medicina.fi/index.php?option=com_content&view=article&id=48&Itemid=56#I | ||
* [http://en.opasnet.org/en-opwiki/index.php?title=Water_guide&oldid=43130#Answer Archived model version]: | |||
** Split version: a corrected model 19.7.2019. | |||
** Old version: The original 2012 version that was the starting point for development | |||
** New version: An intermediate version with bugs | |||
** Model run 12.7.2019 [http://en.opasnet.org/en-opwiki/index.php?title=Special:RTools&id=kNQ3D6fOLcfq08II] | |||
=== Municipality-specific data === | === Municipality-specific data === |
Latest revision as of 14:33, 23 March 2020
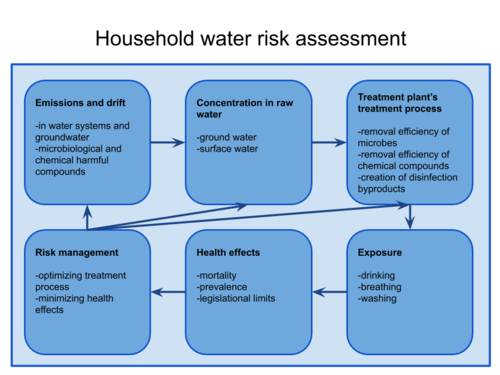
Water guide - An assessment of the health impacts of water quality addresses the potential microbiological health risks of drinking water. These microbiological risks are due to the contamination of raw water with microbes that cause potential health problems for people using tap water, as well as the efficiency of drinking water treatment, which can be insufficient for removing harmful microbes from drinking water. The assessment will be used to determine, how high the health risk is from certain microbes in raw water. The assessment is based on a mathematical Water guide -model, which can be found below.
Question
How to assess the microbiological risks of drinking water as well as their health effects? Information of water treatment plants must be possible to use as raw data.
Answer
The result page opens in a new tab by pressing the Run model -box. It will take approximately 40 seconds for the model to run, after which the results will appear on the result page.
User instructions for the Water guide -model
1. Choose classification of raw water
- Ground water - Clean: clean ground water
- Ground water - Surface water load: f.ex. shore infiltration
- Surface water - Low load: relatively clean surface water
- Surface water - Medium load: f.ex. low waste water load
- Surface water - High load: f.ex. waste water load
OR
Choose 'I will determine microbe concentration by hand'. Write pathogen concentrations in their respective boxes.
2. State the concumption of drinking water per 24 h in liters per day per person (default 1.153 l/d).
3. State the population size of target area. (default 100000)
4. Choose treatment processes used. Obs! You can choose multiple options. If none of the first six treatment processes are used, choose "None of the above cleaning methods". If neither UV nor ozone is used, choose "None of the above disinfection methods".
5. Choose whether chlorination is used or not by giving the chlorine dose used (mg/l). If there is no chlorination, the dose will be 0 mg/l (default 0 mg/l). The model only calculates the effect of chlorine on the water in treatment plants, and ignores any further cleansing effects residual chlorine may have after the water moves onward to the pipe network.
6. You can see the results in a new tab by pressing 'Run model' This opens a new tab, in which the results will appear after the model is done running. This takes approximately 45 seconds.
Rationale
- The model has been translated from Analytica to R. You can find the original model here (page in Finnish, link to the model code at the top): op_fi:Tiedosto:Vesiopas.ANA. If the user does not give concentrations for the pathogens, the values on page Pathogen concentrations in raw water will be used.
Health-based quality requirements and recommendations have been implanted on the quality of household water. In Finland, the ministry of social affairs and health is responsible for the legislation of the quality, and monitoring it is the responsibility of municipal health protection officials. Upholding and developing good water quality require high-standard research and cooperation between different parties. Ground waters and artificial ground waters are usually not disinfected. The chemicals and microbes in raw water and byproducts of disinfection produced in household water production can present health risks to the people using the household water. Water leaving the treatment plant can stay in the pipes for a long time. If the circumstances are favourable for microbial growth, the water can become low in quality and even detrimental to health while in the pipe network.
Household water is produced from either ground or surface water. The problems of these types of waters as raw water are different. Using surface water always requires establishing an actual treatment plant and the associated knowledge, skills, technologies and educated monitoring personnel. Ground water on the other hand doesn't require very complex treatment, so not many treatment plant personnel are needed, and there is less monitoring. Additionally today there are many treatment plants that produce artificial ground water. The qualities of artificial ground water can be similar to good ground waters, but the quality of the water is often only be equal to medium quality surface water.
Treatment of ground water usually includes increasing the pH and and hardness of water. This can be done using different techniques, of which the use of calcium hydroxide and carbon dioxide to regulate pH are the most common. Sometimes also precipitation of iron or manganese is required using strong oxidisers such as permanganate or chlorine. Low quality ground water is also disinfected.
In order to make surface water drinkable, it has to be heavily treated. The hardest thing is to precipitate the humus. This is done by using not only different precipitation chemicals, also for example adjust pH and breaking down the humus with strong oxidisers such as ozone. On top of that, the precipitated chemicals (such as salts of aluminium and iron) need to be removed and water hardness raised so that the pipelines don't disintegrate over time. Also, the population has to be protected from the microbes always present in surface waters, so the water needs to be disinfected to remove pathogens.
The quality of water is also affected by the condition of the pipelines and the water spends in them. If there is a lot of coagulation in the pipelines, these dregs include not only different inorganic compounds but also a large amout of bacterial mass. The coagulates in the pipelines and their qualities have started to be researched in Finland only recently.
The purpose of risk assessment is to produce information of the true size of the risk for decision-making. Risk assessment is a scientific process in which experts hold a key role. Because there are any kinds of risks to study, risk assessment processes can be very different. In environmental health risk assessment consists of four steps:
- Identifying the danger (does the variable researched pose a danger to humans?)
- Assessing the exposure-response function (how big a dose results in how big a response?)
- Assessing exposure (how much are humans exposed?)
- Characterising the risk (how high is the health risk?)[1]
All water treatment plants should periodically do risk assessment on on the household water they produce. It is done to identify actions to be taken in different crisis situations and prepare plans for different problematic situations and possible damage. Different treatment plants and for example vacation centers need different kinds of plans and implementations.
Dependencies
Page | What data or code the page has | What is it used for | Other observations |
---|---|---|---|
Op_en2949 Population of Finland | Data on the age distribution of Finns | Age distribution is used, because susceptibility to diseases and their severity changes with age. The burden of disease and cases of illness are calculated for each age group, then summed. | |
Op_en7956 Drinking water chlorination efficiency | Log-decrease in pathogens due to chlorination, with different doses | The code on this page uses the data on the page and user-given dose for chlorine to calculate the case-specific decrease in pathogens due to chlorination. This is summed with log-decrease of other treatment and disinfection methods to produce the total log-decrease in pathogens from water treatment. | The model only takes into account any decrease in pathogens that happens in water treatment plants. Any further effect residual chlorine has after it enters the pipes is not included. |
Op_en7955 Drinking water disinfection efficiency | Log-decreases of pathogens due to ozonization and UV | If the user has chosen one or both of these disinfection methods, the decrease from the chosen one(s) is summed with the decrease due to chlorination and other treatment methods to produce the total log-decrease in pathogens from water treatment. | Currently the qualities of water, such as turbidity, are not taken into account in the efficiency of disinfection methods. The dose for both methods is also set. |
Op_en7954; Drinking water treatment efficiency | Log-decreases of pathogens due to different treatment methods. These methods are traditional cleaning, well working cleaning, enhanced cleaning, slow sand filtration, limestone filtration and active carbon filtration. | If the user has chosen one of multiple of these treatment methods, the decrease of pathogens they produce is summed with the decreases due to chlorination and other disinfection methods to produce the total log-decrease in pathogens from water treatment. | |
Op_en7953 Pathogen concentrations in raw water | The concentrations of pathogens of different raw water classes | If the user has chosen one of the raw water classes, the amounts of pathogens in that class and the total log-decrease of pathogens from treatment are used to calculate the amount of pathogens left in drinking water. If the user has given pathogen concentrations by hand, those values are used instead of the data on this page. | |
Op_en7947 Case burden of waterborne microbes | Case burdens of different pathogens, or how severe and long-lasting the diseases caused by them are | This data is used to calculate the health detriments caused by the pathogens in drinking water. | |
Op_en7957 Health impacts of waterborne microbes | Doesn't contain data, only code | These codes use water consumption given by the user, total decrease in pathogens and the pathogen concentrations in raw water to calculate the exposure to pathogens experienced by one individual, or how many of each pathogen an individual swallows daily with drinking water. | |
Op_en7948 ERF of waterborne microbes | Describes the exposure-response functions for the pathogens | These functions tell, how likely an individual is to get sick with different exposures. This and the exposure are used to estimate the cases of illness. | |
Op_en2261 HIA | Code that combines all of the above | The code on the page uses exposure, exposure-response functions and the case burdens to calculate the total burdens caused by pathogens in drinking water. From here it is also possible to calculate the number of people getting ill from drinking water. These are the final results of the model. |
Table of decisions used in drawing plotly graphs.
Obs | Decision | Option | Variable | Cell | Change | Description | Result |
---|---|---|---|---|---|---|---|
1 | Adjust | Ground - none | RawConcentration | RawWaterClass: Ground water - surface water contamination | Remove | ||
2 | Adjust | Ground - none | RawConcentration |
Well, this doesn't work at all.
Calculations
Initiate model
Plotly test
Another plotly test
Plotly with two sliders
See also
Moderator:Päivi Meriläinen (see all) |
|
Upload data
|
- op_fi:Arviointi pohjavesilaitoksen mikrobiologisista riskeistä
- op_fi:Arviointi juomaveden kloorifenolien terveysvaikutuksista
- heande:Kuovesi
- op_fi:Mikrobien määrä juomavedessä
- op_fi:Mikrobien määrä pohjavedessä
- Bathing water guide
- WHO: Quantitative Microbial Risk Assessment: Application for Water Safety Management
- http://www.fao.org/docrep/006/y4666e/y4666e06.htm#TopOfPage
- http://wiki.camra.msu.edu/index.php?title=Main_Page
- http://www.medicina.fi/index.php?option=com_content&view=article&id=48&Itemid=56#I
- Archived model version:
- Split version: a corrected model 19.7.2019.
- Old version: The original 2012 version that was the starting point for development
- New version: An intermediate version with bugs
- Model run 12.7.2019 [1]
Municipality-specific data
- Op_fi2603 op_fi:Arviointi juomaveden laadun terveysvaikutuksista/Kuopio (vedenpuhdistamon tietoja)
- heande:Polaris:Raakaveden patogeenipitoisuudet/Lahti
- heande:Polaris:Raakaveden patogeenipitoisuudet/Mikkeli
- heande:Polaris:Raakaveden patogeenipitoisuudet/Kirkkonummi
- heande:Polaris:Paikkakuntakohtaiset tiedot talousveden käsittelyyn/Lahti
- heande:Polaris:Paikkakuntakohtaiset tiedot talousveden käsittelyyn/Mikkeli
- heande:Polaris:Paikkakuntakohtaiset tiedot talousveden käsittelyyn/Kirkkonummi
- heande:Polaris:Juomaveden desinfiointi/Lahti
- heande:Polaris:Juomaveden desinfiointi/Mikkeli
- heande:Polaris:Juomaveden desinfiointi/Kirkkonummi
- heande:Polaris:Vedenkäsittelyn tehokkuus/Lahti
- heande:Polaris:Vedenkäsittelyn tehokkuus/Mikkeli
- heande:Polaris:Vedenkäsittelyn tehokkuus/Kirkkonummi
References
![]() |
![]() |