Health impact of radon in Europe
Moderator:Teemu R (see all) |
|
Upload data
|
Main message: |
---|
Question:
Radon gas in homes is a major environmental health hazard causing lung cancer. Good building policies can reduce radon concentrations in indoor air in homes. What are the effects of different plausible building policies on radon in homes and consequently on lung cancer mortality in Europe between 2010 and 2050? There are currently 43000 (95 % CI 7186-104660) lung cancer deaths due to indoor radon in Europe. This number is likely to increase in the future due to decreased ventilation in aim to reduce energy consumption, if other measures are not taken. It is important to maintain good air exchange conditions and proper building insulation even when energy saving measures are taken. More should be known about practical conditions and potential to radon reduction, as well as about the knowledge level, preparedness, and practical hindrances to take such actions by building owners. Value of information analysis shows that this information is so valuable that putting resources in this research is clearly cost-effective. The ultimate aim should be to reduce energy consumption, greenhouse gas emissions, and indoor radon in a cost-effective and synergistic way. |
Scope
Purpose
What are the health impacts of radon in the indoor air of residential buildings in Europe?
Boundaries etc
Boundaries, scenarios, intended users, and participants are the same as in the Mega case study.
Definition

- Decision variables
- Other variables
- Population of Europe by Country
- WHO mortality data
- heande:HI:Air exchange rate for European residences
- Radon concentrations in European residences
- ERF for long-term indoor exposure to radon and lung cancer
- Indicators
Analyses
Indoor radon case study
- Estimates health impacts of radon in indoor air of residences.
- Nation-wide radon concentration distributions were obtained from EnVIE project and UNSCEAR 2000 report.
- United Kingdom, Czech Republic, and Slovenia were rejected because of lack of data.
- Exposure-response function was 16 % increase of lung cancer incidence due to 100 Bq/m3 increase of radon (Darby 2004 and 2005).
- The same lung cancer background rate was assumed for the whole Europe: 58.2 cases/100000 person-years (Globocan 2008).
- Linear no-threshold ERF assumed for the whole population in each country.
- The model development, data storage, and model runs were all performed in Opasnet using R software and Opasnet Base.
- The main page of the sub-assessment is http://en.opasnet.org/w/Health_impact_of_radon_in_Europe
Outcomes of interest of radon sub-assessment
- Main health impact: number of lung cancer cases attributable to radon.
- Preliminary estimate of DALYs attributable to radon (only draft estimates for duration of disease).
- Disability weight 0.146 (from WHO) used for disease duration.
- Duration of disease estimated at 2-36 months; life-years lost due to lung cancer death estimated at 1-15 years.
- Preliminary estimate of monetary impact was obtained indirectly by converting DALYs into euros; other cost types were ignored.
- One DALY estimated to be worth 30-60 k€.
- Methodological outcome: proof of concept for running assessment models via open internet interface.
R code
- This code features R functions described on Opasnet Base Connection for R and Operating intelligently with multidimensional arrays in R.
library(ggplot2) cancer <- op_baseGetData("opasnet_base", "Op_en4715", exclude = 48823) array <- DataframeToArray(cancer) array <- array[,,,c(2,1,3,4),,] ##### Cases ##### means <- apply(array, c(2,3,4), mean, na.rm=TRUE) means <- apply(means, c(2,3), sum, na.rm=TRUE) plot1 <- as.data.frame(as.table(means)) plot1 <- ggplot(plot1[plot1[,"Freq"]!=0,], aes(Year, weight=Freq, fill=policy)) + geom_bar(position="dodge") + scale_x_discrete("Year") + scale_y_continuous("Cases") plot1 ci <- apply(apply(array, c(1,3,4), sum, na.rm=TRUE), c(2,3), quantile, probs=c(0.025,0.975)) final1 <- means final1[,] <- paste(round(means), " (", round(ci[1,,]), "-", round(ci[2,,]), ")", sep="") final1[c(2:4,7:8,11:12)] <- NA final1 ##### DALYs ##### DALY <- array(NA, dim = c(dim(array), 3), dimnames = dimnames(array)) DALY[,,,,1] <- array DALY[,,,,2] <- runif(dim(array)[1],2,36)/12 DALY[,,,,3] <- runif(dim(array)[1],1,15) DALY <- DALY[,,,,1]*0.146*DALY[,,,,2]+DALY[,,,,1]*DALY[,,,,3] means <- apply(DALY, c(2,3,4), mean, na.rm=TRUE) means <- apply(means, c(2,3), sum, na.rm=TRUE) plot2 <- as.data.frame(as.table(means)) plot2 <- ggplot(plot2[plot2[,"Freq"]!=0,], aes(Year, weight=Freq, fill=policy)) + geom_bar(position="dodge") + scale_x_discrete("Year") + scale_y_continuous("DALYs") plot2 ci <- apply(apply(DALY, c(1,3,4), sum, na.rm=TRUE), c(2,3), quantile, probs=c(0.025,0.975)) final2 <- means final2[,] <- paste(round(means), " (", round(ci[1,,]), "-", round(ci[2,,]), ")", sep="") final2[c(2:4,7:8,11:12)] <- NA final2 ##### Cost ##### mpdaly <- op_baseGetData("opasnet_base", "Op_en4858") cost <- IntArray(mpdaly, DALY, "DALYs") cost <- data.frame(cost[,c("obs","Country","policy","Year")], Result=cost[,"Result"]*cost[,"DALYs"]) cost <- DataframeToArray(cost) cost <- cost[,,c(2,1,3,4),] means <- apply(cost, c(2,3,4), mean, na.rm=TRUE) means <- apply(means, c(2,3), sum, na.rm=TRUE)/10^6 plot3 <- as.data.frame(as.table(means)) plot3 <- ggplot(plot3[plot3[,"Freq"]!=0,], aes(Year, weight=Freq, fill=policy)) + geom_bar(position="dodge") + scale_x_discrete("Year") + scale_y_continuous("Cost (M€)") plot3 ci <- apply(apply(cost, c(1,3,4), sum, na.rm=TRUE), c(2,3), quantile, probs=c(0.025,0.975))/10^6 final3 <- means final3[,] <- paste(round(means), " (", round(ci[1,,]), "-", round(ci[2,,]), ")", sep="") final3[c(2:4,7:8,11:12)] <- NA final3 ##### Probability density plot ##### costdf <- as.data.frame(as.table(apply(cost, c(1,3,4), sum)/1e9)) costdf <- costdf[is.na(costdf[,"Freq"])==FALSE,] plot4 <- ggplot(costdf, aes(x=Freq, y=..density.., fill=policy)) + geom_density(alpha=0.2, adjust=4) + scale_x_continuous(expression("Cost ("*10^9*"€)"), limits = c(-50,300)) + scale_y_continuous("Density") + facet_wrap(~Year) plot4 ##### Expected Value of Perfect Information ##### evpi <- (apply(apply(cost, c(2,3,4), mean, na.rm=TRUE), c(1,3), min, na.rm=TRUE) - apply(apply(cost, c(1,2,4), min, na.rm=TRUE), c(2,3), mean, na.rm=TRUE))/1e6 plot5 <- as.data.frame(as.table(apply(evpi, 2, sum))) plot5 <- ggplot(plot5, aes(Var1, weight=Freq)) + geom_bar(position="dodge") + scale_x_discrete("Year") + scale_y_continuous("Value of perfect information (M€)") plot5 ##### Expected Value of Partial Perfect Information ##### #Same as that of perfect information, because of only one decision variable ae <- op_baseGetData("opasnet_base", "Erac2499") aer <- DataframeToArray(ae) aer <- aer[,,c(2,1,4,5),] dropnonmax <- function(x) { x[x<max(x, na.rm = TRUE)] <- NA return(x) } aer <- apply(aer, c(1,2,4), dropnonmax) aer <- as.data.frame(as.table(aer)) aer <- aer[,c(2,3,1,4,5)] colnames(aer)[3] <- "policy" aer <- aer[is.na(aer[,"Freq"])==FALSE,] aer <- IntArray(aer, cost, "Cost") aer <- DataframeToArray(aer[,c("obs","Country","Year","Cost")],"Cost") test2 <- (apply(apply(cost, c(2,3,4), mean, na.rm=TRUE), c(1,3), min, na.rm=TRUE) - apply(aer, c(2,3), mean))/1e6 plot6 <- as.data.frame(as.table(apply(test2, 2, sum))) plot6 <- ggplot(plot6, aes(Var1, weight=Freq)) + geom_bar(position="dodge") + scale_x_discrete("Year") + scale_y_continuous("Value of perfect information (M€)") plot6 test2==evpi #Test if the values are the same test2-evpi # It seems that for some reason aer evppi is slightly smaller than evpi, # although it should be the only variable that varies in the "policy" dimension
Result
Results
Lung cancer cases due to radon in Europe: {{#opasnet_base_link:Op_en4715}}
- Results for the Biomass scenario are wrong and the scenario is perhaps irrelevant because biomass usage does not affect air exchange rates which this assessment is concerned with, so it should be ignored.

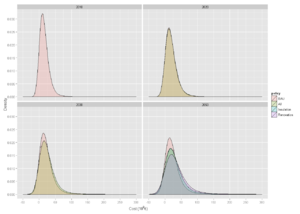

Year | ||||
---|---|---|---|---|
Policy | 2010 | 2020 | 2030 | 2050 |
BAU | 43074 (7186-104660) | 51801 (8934-129303) | 58716 (9427-155621) | 63718 (10407-178566) |
All | NA | 52660 (8892-130780) | 68086 (10544-180827) | 81022 (11983-235695) |
Insulation | NA | NA | NA | 80149 (11898-228747) |
Renovation | NA | NA | NA | 92783 (13365-275851) |
Year | ||||
---|---|---|---|---|
Policy | 2010 | 2020 | 2030 | 2050 |
BAU | 358244 (54193-940205) | 427824 (65203-1156026) | 483005 (70921-1335438) | 524154 (80215-1545369) |
All | NA | 433106 (65625-1140190) | 562607 (78874-1614252) | 663170 (89720-2045648) |
Insulation | NA | NA | NA | 664141 (88638-2108941) |
Renovation | NA | NA | NA | 773105 (107764-2392599) |
Year | ||||
---|---|---|---|---|
Policy | 2010 | 2020 | 2030 | 2050 |
BAU | 16147 (2519-42699) | 19250 (3039-53378) | 21770 (3121-60868) | 23585 (3590-70455) |
All | NA | 19464 (3009-53226) | 25219 (3682-71977) | 29715 (3973-94741) |
Insulation | NA | NA | NA | 29877 (4049-93146) |
Renovation | NA | NA | NA | 34810 (4748-106804) |
Country of observation | Mean | SD |
---|---|---|
Austria | 1071 | 1202 |
Belgium | 885 | 872 |
Bulgaria | 297 | 338 |
Switzerland | 1540 | 2764 |
Cyprus | 9 | 12 |
Germany | 4843 | 4207 |
Denmark | 373 | 400 |
Estonia | 218 | 245 |
Spain | 8460 | 18071 |
Finland | 812 | 802 |
France | 8320 | 12045 |
Greece | 813 | 880 |
Hungary | 1617 | 2161 |
Ireland | 552 | 636 |
Italy | 4952 | 4444 |
Lithuania | 236 | 277 |
Luxembourg | 69 | 64 |
Latvia | 226 | 294 |
Malta | 43 | 50 |
Netherlands | 523 | 350 |
Norway | 575 | 673 |
Poland | 2264 | 2151 |
Portugal | 1140 | 1214 |
Romania | 1236 | 1313 |
Sweden | 1375 | 1600 |
Slovakia | 626 | 678 |
Total | 43074 |
Conclusions
See also
- Radon
- ERF for long-term indoor exposure to radon and lung cancer
- An Overview of Radon Surveys in Europe. Joint Research Centre, 2005. ISBN 92-79-01066-2
- In Heande (password-protected)
- heande:HI:Radon Indoor Air Case
Keywords
Radon, indoor air, lung cancer, Europe
References
Related files
<mfanonymousfilelist></mfanonymousfilelist>