Goherr: Fish consumption study: Difference between revisions
Line 469: | Line 469: | ||
* [https://stats.stackexchange.com/questions/8661/logistic-regression-in-r-odds-ratio Calculate odds ratios in logistic regression] | * [https://stats.stackexchange.com/questions/8661/logistic-regression-in-r-odds-ratio Calculate odds ratios in logistic regression] | ||
* [https://www3.nd.edu/~rwilliam/stats3/ordinalindependent.pdf You might treat independent ordinal variables as continuous] | * [https://www3.nd.edu/~rwilliam/stats3/ordinalindependent.pdf You might treat independent ordinal variables as continuous] | ||
* [https://www.color-blindness.com/coblis-color-blindness-simulator/ Color blindness simulator to adjust colors for the color blind and for black and white printing] | |||
<rcode graphics=1> | <rcode graphics=1> | ||
Line 477: | Line 478: | ||
library(reshape2) | library(reshape2) | ||
library(MASS) | library(MASS) | ||
#library(thlVerse) | |||
#library(car) | #library(car) | ||
#library(vegan) | #library(vegan) | ||
BS <- 24 | BS <- 24 | ||
localcomp <- TRUE | |||
# Set colours | |||
#library(thlVerse) | |||
#library(tidyverse) | |||
#colors <- c( | |||
# thlColors(6,"quali","bar",thin=0.8), | |||
# thlColors(6,"quali","bar",thin=1) | |||
#)[c(1,8,3,10,11,6)] | |||
#ggplot(tibble(A=as.character(1:6),B=1),aes(x=A,weight=B,fill=A))+ | |||
# geom_bar()+ | |||
# scale_fill_manual(values=colors) | |||
colors <- c("#74AF59FF","#2F62ADFF","#D692BDFF","#29A0C1FF","#BE3F72FF","#FBB848FF") | |||
########################## Functions | ########################## Functions | ||
Line 532: | Line 549: | ||
tmp <- ggplot(tmp@output, aes(x=Reason, y=Result,colour=Country, group=Country))+ | tmp <- ggplot(tmp@output, aes(x=Reason, y=Result,colour=Country, group=Country))+ | ||
geom_point(shape=21, size= | geom_point(shape=21, size=5, fill="Grey", stroke=2)+ | ||
geom_line()+ | geom_line(size=1.2)+ | ||
coord_flip()+ | coord_flip()+ | ||
theme_gray(base_size=BS)+ | theme_gray(base_size=BS)+ | ||
scale_y_continuous(labels=scales::percent_format())+ | scale_y_continuous(labels=scales::percent_format())+#accuracy=1))+ | ||
scale_colour_manual(values=colors)+ | |||
labs( | labs( | ||
title=title, | title=title, | ||
Line 600: | Line 618: | ||
theme_gray(base_size=BS)+ | theme_gray(base_size=BS)+ | ||
theme(legend.position = "bottom")+ | theme(legend.position = "bottom")+ | ||
scale_fill_manual(values=c("Grey", | scale_fill_manual(values=c("Grey",colors[c(1,6,5)]))+ | ||
scale_y_continuous(breaks=c(0.5,1),labels=scales::percent_format())+ | scale_y_continuous(breaks=c(0.5,1),labels=scales::percent_format())+ | ||
labs( | labs( | ||
Line 745: | Line 763: | ||
geom_bar(aes(y=..prop.., fill=Country), position="dodge")+ | geom_bar(aes(y=..prop.., fill=Country), position="dodge")+ | ||
theme_gray(base_size=BS)+ | theme_gray(base_size=BS)+ | ||
scale_fill_manual(values=colors)+ | |||
scale_y_continuous(labels=scales::percent_format())+ | scale_y_continuous(labels=scales::percent_format())+ | ||
labs(title="Fraction of fish eaters within population") | labs(title="Fraction of fish eaters within population") | ||
Line 750: | Line 769: | ||
############## Answers to open questions | ############## Answers to open questions | ||
if(FALSE){ | if(FALSE){ | ||
cat("Number of open-ended answers per question and country\n") | cat("Number of open-ended answers per question and country\n") | ||
tmp <- survey1[c(1,3,27:28,36:37,44:45,60:61,71:72,83:84,93:94,109:110,121:122,133:134,154,157:158)] | tmp <- survey1[c(1,3,27:28,36:37,44:45,60:61,71:72,83:84,93:94,109:110,121:122,133:134,154,157:158)] | ||
tmp <- reshape( | tmp <- reshape( | ||
tmp, | |||
varying=list( | |||
Condition = colnames(tmp)[grepl("other",tolower(colnames(tmp)))], | |||
Text = colnames(tmp)[grepl("open",colnames(tmp))] | |||
), | |||
times = colnames(tmp)[grepl("other",tolower(colnames(tmp)))], | |||
direction="long" | |||
) | ) | ||
tmp <- tmp[tmp$Why.not.fish.open!="" , ] | tmp <- tmp[tmp$Why.not.fish.open!="" , ] | ||
oprint(table(tmp$time,tmp$Country)) | oprint(table(tmp$time,tmp$Country)) | ||
oprint(tmp) | oprint(tmp) | ||
} # End if(FALSE) | } # End if(FALSE) | ||
Line 782: | Line 801: | ||
"Species used among salmon consumers" | "Species used among salmon consumers" | ||
) | ) | ||
reasons( | reasons( | ||
survey1[survey1$Baltic.salmon=="Yes"& !is.na(survey1$Baltic.salmon),c(1,50:59,154,157)], # 1 Country, 17 Why Baltic salmon | survey1[survey1$Baltic.salmon=="Yes"& !is.na(survey1$Baltic.salmon),c(1,50:59,154,157)], # 1 Country, 17 Why Baltic salmon | ||
"Reasons to eat among Baltic salmon consumers" | "Reasons to eat among Baltic salmon consumers" | ||
) | ) | ||
if(localcomp) ggsave("Figure 6.pdf", width=12,height=7) | |||
reasons( | reasons( | ||
survey1[survey1$Baltic.salmon=="No"& !is.na(survey1$Baltic.salmon),c(1,62:70,154,157)], # 1 Country, 18 Why not Baltic salmon | survey1[survey1$Baltic.salmon=="No"& !is.na(survey1$Baltic.salmon),c(1,62:70,154,157)], # 1 Country, 18 Why not Baltic salmon | ||
"Reasons not to eat | "Reasons not to eat, Baltic salmon non-consumers" | ||
) | ) | ||
impacts. | if(localcomp) ggsave("Figure 7.pdf", width=12,height=7) | ||
impacts.sal <- impacts( | |||
survey1[survey1$Baltic.salmon=="Yes"& !is.na(survey1$Baltic.salmon),c(1,73:82,154)], | survey1[survey1$Baltic.salmon=="Yes"& !is.na(survey1$Baltic.salmon),c(1,73:82,154)], | ||
"Effect on Baltic salmon consumption", | "Effect on Baltic salmon consumption", | ||
Line 806: | Line 830: | ||
theme(legend.position = "bottom")+ | theme(legend.position = "bottom")+ | ||
scale_y_continuous(labels=scales::percent_format())+ | scale_y_continuous(labels=scales::percent_format())+ | ||
scale_fill_manual(values=colors)+ | |||
labs( | labs( | ||
title="Any herring consumption", | title="Any herring consumption", | ||
Line 819: | Line 843: | ||
survey1$What <- factor( | survey1$What <- factor( | ||
survey1$What, | survey1$What, | ||
levels=c("Yes","No","Other fish","I don't know"), | levels=rev(c("Yes","No","Other fish","I don't know")), | ||
labels=c("Baltic herring","Other herring","Other fish","I don't know") | labels=rev(c("Baltic herring","Other herring","Other fish","I don't know")) | ||
) | ) | ||
Line 831: | Line 855: | ||
theme(legend.position = "bottom")+ | theme(legend.position = "bottom")+ | ||
scale_y_continuous(labels=scales::percent_format())+ | scale_y_continuous(labels=scales::percent_format())+ | ||
scale_fill_manual(values=c("gray",colors))+ | |||
guides(fill=guide_legend(reverse=TRUE))+ | |||
labs( | labs( | ||
title="Baltic herring consumption", | title="Baltic herring consumption", | ||
y="Fraction of fish consumers" | y="Fraction of fish consumers" | ||
) | ) | ||
if(localcomp) ggsave("Figure 2.pdf",width=10, height=5) | |||
rown <- tmp$What[tmp$Country=="FI"] | rown <- tmp$What[tmp$Country=="FI"] | ||
Line 841: | Line 868: | ||
colnames(tmp[[2]]) <- rown | colnames(tmp[[2]]) <- rown | ||
oprint(as.matrix(tmp)) | oprint(as.matrix(tmp)) | ||
ggplot(survey1[survey1$Eat.herring=="Yes",], | ggplot(survey1[survey1$Eat.herring=="Yes",], | ||
Line 848: | Line 876: | ||
theme(legend.position = "bottom")+ | theme(legend.position = "bottom")+ | ||
scale_y_continuous(labels=scales::percent_format())+ | scale_y_continuous(labels=scales::percent_format())+ | ||
scale_fill_manual(values=c(colors))+ | |||
labs( | labs( | ||
title="Baltic herring consumption", | title="Baltic herring consumption", | ||
Line 862: | Line 890: | ||
theme(legend.position = "bottom")+ | theme(legend.position = "bottom")+ | ||
scale_y_continuous(labels=scales::percent_format())+ | scale_y_continuous(labels=scales::percent_format())+ | ||
scale_fill_manual(values=colors)+ | |||
labs( | labs( | ||
title="Any salmon consumption", | title="Any salmon consumption", | ||
Line 876: | Line 904: | ||
theme(legend.position = "bottom")+ | theme(legend.position = "bottom")+ | ||
scale_y_continuous(labels=scales::percent_format())+ | scale_y_continuous(labels=scales::percent_format())+ | ||
scale_fill_manual(values=colors)+ | |||
labs( | labs( | ||
title="Baltic salmon consumption", | title="Baltic salmon consumption", | ||
Line 888: | Line 916: | ||
theme(legend.position = "bottom")+ | theme(legend.position = "bottom")+ | ||
scale_y_continuous(labels=scales::percent_format())+ | scale_y_continuous(labels=scales::percent_format())+ | ||
scale_fill_manual(values=colors)+ | |||
labs( | labs( | ||
title="Baltic salmon consumption", | title="Baltic salmon consumption", | ||
Line 903: | Line 931: | ||
theme(legend.position = "bottom")+ | theme(legend.position = "bottom")+ | ||
scale_y_continuous(labels=scales::percent_format())+ | scale_y_continuous(labels=scales::percent_format())+ | ||
scale_fill_manual(values=colors)+ | |||
labs(title="Awareness of food recommendations about Baltic fish") | labs(title="Awareness of food recommendations about Baltic fish") | ||
Line 912: | Line 940: | ||
"Reasons to eat among Baltic herring consumers" | "Reasons to eat among Baltic herring consumers" | ||
) | ) | ||
if(localcomp) ggsave("Figure 4.pdf", width=12, height=7) | |||
# Figure 5. Reasons for not to eat Baltic herring. | # Figure 5. Reasons for not to eat Baltic herring. | ||
Line 921: | Line 951: | ||
survey1$Country %in% c("FI","SE") & | survey1$Country %in% c("FI","SE") & | ||
!is.na(survey1$Eat.BH),c(1,111:120,154,157)], # 1 Country, 28 Why not Baltic herring | !is.na(survey1$Eat.BH),c(1,111:120,154,157)], # 1 Country, 28 Why not Baltic herring | ||
"Reasons not to eat | "Reasons not to eat, Baltic herring non-consumers" | ||
) | ) | ||
if(localcomp) ggsave("Figure 5.pdf", width=12, height=7) | |||
# Figure 9. The role of different determinants on Baltic salmon consumption | # Figure 9. The role of different determinants on Baltic salmon consumption | ||
impacts. | impacts.herr <- impacts( | ||
survey1[survey1$Eat.BH=="Yes"& !is.na(survey1$Eat.BH),c(1,123:132,154)], # 1 Country, 29 Influence of Baltic herring policies | survey1[survey1$Eat.BH=="Yes"& !is.na(survey1$Eat.BH),c(1,123:132,154)], # 1 Country, 29 Influence of Baltic herring policies | ||
title="Effect on Baltic herring consumption", | title="Effect on Baltic herring consumption", | ||
Line 943: | Line 975: | ||
) | ) | ||
# Figure | # Figure 8. | ||
ggplot(tmp, aes(x=Reason, weight=Result, fill=Response))+geom_bar()+ | ggplot(tmp, aes(x=Reason, weight=Result, fill=Response))+geom_bar()+ | ||
Line 949: | Line 981: | ||
theme_gray(base_size=BS)+ | theme_gray(base_size=BS)+ | ||
theme(legend.position = "bottom")+ | theme(legend.position = "bottom")+ | ||
scale_fill_manual(values=c(" | scale_fill_manual(values=c("gray",colors[c(1,6,5)]))+ | ||
scale_y_continuous(breaks=c(0.5,1),labels=scales::percent_format())+ | scale_y_continuous(breaks=c(0.5,1),labels=scales::percent_format())+#accuracy=1))+ | ||
guides(fill=guide_legend(reverse=TRUE))+ | |||
labs( | labs( | ||
title="Effect on Baltic fish consumption", | title="Effect on Baltic fish consumption", | ||
Line 978: | Line 1,011: | ||
geom_bar()+facet_wrap(~Country)+ | geom_bar()+facet_wrap(~Country)+ | ||
theme_gray(base_size=BS)+ | theme_gray(base_size=BS)+ | ||
scale_fill_manual(values=colors)+ | |||
guides(fill=FALSE)+ | |||
theme(axis.text.x = element_text(angle = -90))+ | theme(axis.text.x = element_text(angle = -90))+ | ||
labs( | labs( | ||
title="Baltic herring consumption in subgroups", | title="Baltic herring consumption in subgroups", | ||
y="Average consumption (kg/year)") | y="Average consumption (kg/year)") | ||
if(localcomp) ggsave("Figure3.pdf", width=9, height=10) | |||
tmp$Result <- paste0(sprintf("%.1f",tmp$amountResult), " (",sprintf("%.1f", tmp$SD,1),")") | tmp$Result <- paste0(sprintf("%.1f",tmp$amountResult), " (",sprintf("%.1f", tmp$SD,1),")") | ||
Line 1,111: | Line 1,148: | ||
tmp <- printregr(dat2, j, "What explains the reason to not eat Baltic herring?") | tmp <- printregr(dat2, j, "What explains the reason to not eat Baltic herring?") | ||
} | } | ||
# tmp <- printregr(dat2, "Eat.salmon", "What explains whether people eat any salmon compared with other fish?") | # tmp <- printregr(dat2, "Eat.salmon", "What explains whether people eat any salmon compared with other fish?") | ||
# tmp <- printregr(dat2, "Baltic.salmon", "What explains whether people eat Baltic salmon compared with other salmon?") | # tmp <- printregr(dat2, "Baltic.salmon", "What explains whether people eat Baltic salmon compared with other salmon?") | ||
</rcode> | </rcode> | ||
<t2b name="Fish consumption as food in Finland" index="Origin,Species,Year" locations="1999,2000,2001,2002,2003,2004,2005,2006,2007,2008,2009,2010,2011,2012,2013,2014,2015,2016,2017" unit="kg/a per person"> | |||
domestic fish|Total|6.1|6.1|5.9|6.2|5.8|5.3|5.2|5.0|5.0|4.4|4.5|4.3|3.8|3.8|3.8|4.0|4.1|4.1|4.1 | |||
domestic fish|Kasvatettu kirjolohi|1.6|1.6|1.6|1.6|1.3|1.3|1.4|1.1|1.1|1.2|1.2|1.1|1.0|1.0|1.1|1.1|1.3|1.2|1.2 | |||
domestic fish|Silakka|0.8|1.2|1.1|1.1|0.9|0.8|0.7|0.5|0.4|0.4|0.4|0.3|0.3|0.3|0.3|0.3|0.3|0.3|0.3 | |||
domestic fish|Hauki|0.8|0.7|0.7|0.7|0.6|0.7|0.7|0.8|0.7|0.6|0.6|0.6|0.5|0.4|0.4|0.5|0.5|0.4|0.4 | |||
domestic fish|Ahven|0.7|0.7|0.7|0.7|0.6|0.6|0.6|0.7|0.7|0.5|0.5|0.5|0.4|0.4|0.4|0.5|0.5|0.4|0.4 | |||
domestic fish|Muikku|0.7|0.7|0.7|0.7|0.8|0.8|0.7|0.6|0.6|0.6|0.6|0.7|0.6|0.6|0.6|0.6|0.6|0.5|0.6 | |||
domestic fish|Siika|0.4|0.4|0.4|0.4|0.3|0.3|0.3|0.3|0.5|0.3|0.3|0.3|0.3|0.3|0.3|0.3|0.2|0.3|0.3 | |||
domestic fish|Kuha|0.3|0.2|0.2|0.2|0.3|0.3|0.3|0.3|0.3|0.3|0.3|0.3|0.3|0.3|0.3|0.3|0.3|0.4|0.4 | |||
domestic fish|Muu kotimainen kala|0.8|0.6|0.5|0.8|1.0|0.5|0.5|0.7|0.7|0.5|0.6|0.5|0.4|0.5|0.4|0.5|0.4|0.5|0.5 | |||
imported fish|Total|6.0|6.2|7.0|7.1|8.0|8.6|7.9|8.6|9.7|9.7|9.3|10.2|11.1|10.9|10.8|10.9|10.2|9.1|9.8 | |||
imported fish|Kasvatettu kirjolohi|0.2|0.3|0.4|0.6|0.9|0.6|0.6|0.7|1.0|1.0|0.8|0.8|0.9|1.0|0.9|0.9|0.8|0.9|0.8 | |||
imported fish|Kasvatettu lohi|1.0|0.9|1.2|1.3|1.6|2.2|1.9|2.0|2.7|2.6|2.9|3.1|3.9|4.2|4.0|4.4|4.1|3.5|4.0 | |||
imported fish|Tonnikala (säilykkeet ja valmisteet)|1.0|1.2|1.4|1.4|1.5|1.6|1.6|1.5|1.7|1.7|1.6|1.7|1.7|1.6|1.9|1.7|1.6|1.4|1.5 | |||
imported fish|Seiti (pakastefile)|0.7|0.6|0.7|0.7|0.7|0.6|0.4|0.5|0.5|0.5|0.5|0.5|0.6|0.5|0.5|0.5|0.5|0.4|0.4 | |||
imported fish|Katkarapu|0.5|0.4|0.5|0.5|0.5|0.5|0.5|0.5|0.6|0.6|0.6|0.7|0.7|0.6|0.6|0.5|0.5|0.4|0.4 | |||
imported fish|Silli ja silakka (säilykkeet)|0.6|0.5|0.6|0.6|0.5|0.4|0.5|0.6|0.5|0.6|0.3|0.5|0.4|0.3|0.4|0.5|0.5|0.5|0.5 | |||
imported fish|Muu tuontikala|2.0|2.3|2.2|2.0|2.3|2.7|2.4|2.8|2.7|2.7|2.6|2.9|2.9|2.7|2.5|2.3|2.3|1.9|2.2 | |||
</t2b> | |||
==== Descriptive statistics ==== | ==== Descriptive statistics ==== |
Revision as of 12:58, 22 March 2019
This page is a study.
The page identifier is Op_en7749 |
---|
Moderator:Arja (see all) |
This page is a stub. You may improve it into a full page. |
Upload data
|
Question
How Baltic herring and salmon are used as human food in Baltic sea countries? Which determinants affect on people’s eating habits of these fish species?
Answer
Original questionnaire analysis results
- 13.3.2017 ----#: . These should be presented somewhere --Arja (talk) 07:39, 26 April 2017 (UTC) (type: truth; paradigms: science: comment)
Consumption amount estimates
- Model run 21.4.2017 [1] first distribution
- Model run 18.5.2017 with modelled data; with direct survey data
Rationale
Survey of eating habits of Baltic herring and salmon in Denmark, Estonia, Finland and Sweden has been done in September 2016 by Taloustutkimus oy. Content of the questionnaire can be accessed in Google drive. The actual data can be found from the link below (see Data).
Data
Questionnaire
Original datafile File:Goherr fish consumption.csv.
Show details | ||||||||||||||||||||||||||||||||||||||||||||||||||||||||||||||||||||||||||||||||||||||||||||||||||||||||||||||||||||||||||||||||||||||||||||||||||||||||||||||||||||||||||||||||||||||||||||||||||||||||||||||||||||||||||||||||||||||||||||||||||||||||||||||||||||||||||||||||||||||||||||||||||||||||||||||||||||||||||||||||||||||||||||||||||||||||||||||||||||||||||||||||||||||||||||||||||||||||||||||||||||||||||||||||||||||||||||||||||||||||||||||||||||||||||||||||||||||||||||||||||||||||||||||||||||||||||||||||||||||||||||||||||||||||||||||||||||||||||||||||||||||||||||||||||||||||||||||||||||||||||||||||||||||||||||||||||||||||||||||||||||||||||||||||||||||||||||||||||||||||||||||||||||||||||||||||||||||||||||||||||||||||||||||||||||||||||||||||||||||||||||||||||||||||||||||||||||||||||||||||||||||||||||||||||||||||||||||||||||||||||||||||||||||||||||||||||||||||||||||||
---|---|---|---|---|---|---|---|---|---|---|---|---|---|---|---|---|---|---|---|---|---|---|---|---|---|---|---|---|---|---|---|---|---|---|---|---|---|---|---|---|---|---|---|---|---|---|---|---|---|---|---|---|---|---|---|---|---|---|---|---|---|---|---|---|---|---|---|---|---|---|---|---|---|---|---|---|---|---|---|---|---|---|---|---|---|---|---|---|---|---|---|---|---|---|---|---|---|---|---|---|---|---|---|---|---|---|---|---|---|---|---|---|---|---|---|---|---|---|---|---|---|---|---|---|---|---|---|---|---|---|---|---|---|---|---|---|---|---|---|---|---|---|---|---|---|---|---|---|---|---|---|---|---|---|---|---|---|---|---|---|---|---|---|---|---|---|---|---|---|---|---|---|---|---|---|---|---|---|---|---|---|---|---|---|---|---|---|---|---|---|---|---|---|---|---|---|---|---|---|---|---|---|---|---|---|---|---|---|---|---|---|---|---|---|---|---|---|---|---|---|---|---|---|---|---|---|---|---|---|---|---|---|---|---|---|---|---|---|---|---|---|---|---|---|---|---|---|---|---|---|---|---|---|---|---|---|---|---|---|---|---|---|---|---|---|---|---|---|---|---|---|---|---|---|---|---|---|---|---|---|---|---|---|---|---|---|---|---|---|---|---|---|---|---|---|---|---|---|---|---|---|---|---|---|---|---|---|---|---|---|---|---|---|---|---|---|---|---|---|---|---|---|---|---|---|---|---|---|---|---|---|---|---|---|---|---|---|---|---|---|---|---|---|---|---|---|---|---|---|---|---|---|---|---|---|---|---|---|---|---|---|---|---|---|---|---|---|---|---|---|---|---|---|---|---|---|---|---|---|---|---|---|---|---|---|---|---|---|---|---|---|---|---|---|---|---|---|---|---|---|---|---|---|---|---|---|---|---|---|---|---|---|---|---|---|---|---|---|---|---|---|---|---|---|---|---|---|---|---|---|---|---|---|---|---|---|---|---|---|---|---|---|---|---|---|---|---|---|---|---|---|---|---|---|---|---|---|---|---|---|---|---|---|---|---|---|---|---|---|---|---|---|---|---|---|---|---|---|---|---|---|---|---|---|---|---|---|---|---|---|---|---|---|---|---|---|---|---|---|---|---|---|---|---|---|---|---|---|---|---|---|---|---|---|---|---|---|---|---|---|---|---|---|---|---|---|---|---|---|---|---|---|---|---|---|---|---|---|---|---|---|---|---|---|---|---|---|---|---|---|---|---|---|---|---|---|---|---|---|---|---|---|---|---|---|---|---|---|---|---|---|---|---|---|---|---|---|---|---|---|---|---|---|---|---|---|---|---|---|---|---|---|---|---|---|---|---|---|---|---|---|---|---|---|---|---|---|---|---|---|---|---|---|---|---|---|---|---|---|---|---|---|---|---|---|---|---|---|---|---|---|---|---|---|---|---|---|---|---|---|---|---|---|---|---|---|---|---|---|---|---|---|---|---|---|---|---|---|---|---|---|---|---|---|---|---|---|---|---|---|---|---|---|---|---|---|---|---|---|---|---|---|---|---|---|---|---|---|---|---|---|---|---|---|---|---|---|---|---|---|---|---|---|---|---|---|---|---|---|---|---|---|---|---|---|---|---|---|---|---|---|---|---|---|---|---|---|---|---|---|---|---|---|---|---|---|---|---|---|---|---|---|---|---|---|---|---|---|---|---|---|---|---|---|---|---|---|---|---|---|---|---|---|---|---|---|---|---|---|---|---|---|---|---|---|---|---|---|---|---|---|---|---|---|---|---|---|---|---|---|---|---|---|---|---|---|---|---|---|---|---|---|---|---|---|---|---|---|---|---|---|---|---|---|---|---|---|---|---|---|---|---|---|---|---|---|---|---|---|---|---|---|---|---|---|---|---|---|---|---|---|---|---|---|---|---|---|---|---|---|---|---|---|---|---|---|---|---|---|---|---|---|---|---|---|---|---|---|---|---|---|---|---|---|---|---|---|---|---|---|
|
Assumptions
The following assumptions are used:
Obs | Variable | Value | Unit | Result | Description |
---|---|---|---|---|---|
1 | freq | 1 | times /a | 0 | Never |
2 | freq | 2 | times /a | 0.5 - 0.9 | less than once a year |
3 | freq | 3 | times /a | 2 - 5 | A few times a year |
4 | freq | 4 | times /a | 12 - 36 | 1 - 3 times per month |
5 | freq | 5 | times /a | 52 | once a week |
6 | freq | 6 | times /a | 104 - 208 | 2 - 4 times per week |
7 | freq | 7 | times /a | 260 - 364 | 5 or more times per week |
8 | amdish | 1 | g /serving | 20 - 70 | 1/6 plate or below (50 grams) |
9 | amdish | 2 | g /serving | 70 - 130 | 1/3 plate (100 grams) |
10 | amdish | 3 | g /serving | 120 - 180 | 1/2 plate (150 grams) |
11 | amdish | 4 | g /serving | 170 - 230 | 2/3 plate (200 grams) |
12 | amdish | 5 | g /serving | 220 - 280 | 5/6 plate (250 grams) |
13 | amdish | 6 | g /serving | 270 - 400 | full plate (300 grams) |
14 | amdish | 7 | g /serving | 400 - 550 | overly full plate (500 grams) |
15 | ingredient | fraction | 0.1 - 0.3 | Fraction of fish in the dish | |
16 | amside | 1 | g /serving | 20 - 70 | 1/6 plate or below (50 grams) |
17 | amside | 2 | g /serving | 70 - 130 | 1/4 plate (100 grams) |
18 | amside | 3 | g /serving | 120 - 180 | 1/2 plate (150 grams) |
19 | amside | 4 | g /serving | 170 - 230 | 2/3 plate (200 grams) |
20 | amside | 5 | g /serving | 220 - 280 | 5/6 plate (250 grams) |
21 | change | 1 | fraction | -1 - -0.8 | Decrease it to zero |
22 | change | 2 | fraction | -0.9 - -0.5 | Decrease it to less than half |
23 | change | 3 | fraction | -0.6 - -0.1 | Decrease it a bit |
24 | change | 4 | fraction | 0 | No effect |
25 | change | 5 | fraction | 0.1 - 0.6 | Increase it a bit |
26 | change | 6 | fraction | 0.5 - 0.9 | Increase it over by half |
27 | change | 7 | fraction | 0.8 - 1.3 | Increase it over to double |
28 | change | 8 | fraction | -0.3 - 0.3 | Don't know |
Preprocessing
This code is used to preprocess the original questionnaire data from the above .csv file and to store the data as a usable variable to Opasnet base. The code stores a data.frame named survey.
- Model run 11.7.2018 [2]
Analyses
- Sketches about modelling determinants of eating (spring 2018) [3]
Figures, tables and stat analyses for the first manuscript
- Model run 15.6.2018 [4]
- Model run 16.6.2018 [5]
- Model run 20.6.2018 [6]
- Model run 11.7.2018 [7]
- Model run 9.8.2018 [8]
- Model run 12.8.2018 [9]
- Model run 12.9.2018 with country-specific regression analyses, open-ended answers and fig about eating any herring [10]
- Model run 5.2.2019 [11]
- Model run 6.2.2019 [12]
- Model run 7.2.2019 with systematic analyses of reasons to eat and not to eat Baltic herring [13]
- Model run 20.3.2019 with country-specific regression analyses [14]: printregr(countries=TRUE)
Methodological concerns:
- Is the warning in logistic regression important?
- Goodness of fit in logistic regression
- Calculate odds ratios in logistic regression
- You might treat independent ordinal variables as continuous
- Color blindness simulator to adjust colors for the color blind and for black and white printing
Obs | Origin | Species | 1999 | 2000 | 2001 | 2002 | 2003 | 2004 | 2005 | 2006 | 2007 | 2008 | 2009 | 2010 | 2011 | 2012 | 2013 | 2014 | 2015 | 2016 | 2017 |
---|---|---|---|---|---|---|---|---|---|---|---|---|---|---|---|---|---|---|---|---|---|
1 | domestic fish | Total | 6.1 | 6.1 | 5.9 | 6.2 | 5.8 | 5.3 | 5.2 | 5.0 | 5.0 | 4.4 | 4.5 | 4.3 | 3.8 | 3.8 | 3.8 | 4.0 | 4.1 | 4.1 | 4.1 |
2 | domestic fish | Kasvatettu kirjolohi | 1.6 | 1.6 | 1.6 | 1.6 | 1.3 | 1.3 | 1.4 | 1.1 | 1.1 | 1.2 | 1.2 | 1.1 | 1.0 | 1.0 | 1.1 | 1.1 | 1.3 | 1.2 | 1.2 |
3 | domestic fish | Silakka | 0.8 | 1.2 | 1.1 | 1.1 | 0.9 | 0.8 | 0.7 | 0.5 | 0.4 | 0.4 | 0.4 | 0.3 | 0.3 | 0.3 | 0.3 | 0.3 | 0.3 | 0.3 | 0.3 |
4 | domestic fish | Hauki | 0.8 | 0.7 | 0.7 | 0.7 | 0.6 | 0.7 | 0.7 | 0.8 | 0.7 | 0.6 | 0.6 | 0.6 | 0.5 | 0.4 | 0.4 | 0.5 | 0.5 | 0.4 | 0.4 |
5 | domestic fish | Ahven | 0.7 | 0.7 | 0.7 | 0.7 | 0.6 | 0.6 | 0.6 | 0.7 | 0.7 | 0.5 | 0.5 | 0.5 | 0.4 | 0.4 | 0.4 | 0.5 | 0.5 | 0.4 | 0.4 |
6 | domestic fish | Muikku | 0.7 | 0.7 | 0.7 | 0.7 | 0.8 | 0.8 | 0.7 | 0.6 | 0.6 | 0.6 | 0.6 | 0.7 | 0.6 | 0.6 | 0.6 | 0.6 | 0.6 | 0.5 | 0.6 |
7 | domestic fish | Siika | 0.4 | 0.4 | 0.4 | 0.4 | 0.3 | 0.3 | 0.3 | 0.3 | 0.5 | 0.3 | 0.3 | 0.3 | 0.3 | 0.3 | 0.3 | 0.3 | 0.2 | 0.3 | 0.3 |
8 | domestic fish | Kuha | 0.3 | 0.2 | 0.2 | 0.2 | 0.3 | 0.3 | 0.3 | 0.3 | 0.3 | 0.3 | 0.3 | 0.3 | 0.3 | 0.3 | 0.3 | 0.3 | 0.3 | 0.4 | 0.4 |
9 | domestic fish | Muu kotimainen kala | 0.8 | 0.6 | 0.5 | 0.8 | 1.0 | 0.5 | 0.5 | 0.7 | 0.7 | 0.5 | 0.6 | 0.5 | 0.4 | 0.5 | 0.4 | 0.5 | 0.4 | 0.5 | 0.5 |
10 | imported fish | Total | 6.0 | 6.2 | 7.0 | 7.1 | 8.0 | 8.6 | 7.9 | 8.6 | 9.7 | 9.7 | 9.3 | 10.2 | 11.1 | 10.9 | 10.8 | 10.9 | 10.2 | 9.1 | 9.8 |
11 | imported fish | Kasvatettu kirjolohi | 0.2 | 0.3 | 0.4 | 0.6 | 0.9 | 0.6 | 0.6 | 0.7 | 1.0 | 1.0 | 0.8 | 0.8 | 0.9 | 1.0 | 0.9 | 0.9 | 0.8 | 0.9 | 0.8 |
12 | imported fish | Kasvatettu lohi | 1.0 | 0.9 | 1.2 | 1.3 | 1.6 | 2.2 | 1.9 | 2.0 | 2.7 | 2.6 | 2.9 | 3.1 | 3.9 | 4.2 | 4.0 | 4.4 | 4.1 | 3.5 | 4.0 |
13 | imported fish | Tonnikala (säilykkeet ja valmisteet) | 1.0 | 1.2 | 1.4 | 1.4 | 1.5 | 1.6 | 1.6 | 1.5 | 1.7 | 1.7 | 1.6 | 1.7 | 1.7 | 1.6 | 1.9 | 1.7 | 1.6 | 1.4 | 1.5 |
14 | imported fish | Seiti (pakastefile) | 0.7 | 0.6 | 0.7 | 0.7 | 0.7 | 0.6 | 0.4 | 0.5 | 0.5 | 0.5 | 0.5 | 0.5 | 0.6 | 0.5 | 0.5 | 0.5 | 0.5 | 0.4 | 0.4 |
15 | imported fish | Katkarapu | 0.5 | 0.4 | 0.5 | 0.5 | 0.5 | 0.5 | 0.5 | 0.5 | 0.6 | 0.6 | 0.6 | 0.7 | 0.7 | 0.6 | 0.6 | 0.5 | 0.5 | 0.4 | 0.4 |
16 | imported fish | Silli ja silakka (säilykkeet) | 0.6 | 0.5 | 0.6 | 0.6 | 0.5 | 0.4 | 0.5 | 0.6 | 0.5 | 0.6 | 0.3 | 0.5 | 0.4 | 0.3 | 0.4 | 0.5 | 0.5 | 0.5 | 0.5 |
17 | imported fish | Muu tuontikala | 2.0 | 2.3 | 2.2 | 2.0 | 2.3 | 2.7 | 2.4 | 2.8 | 2.7 | 2.7 | 2.6 | 2.9 | 2.9 | 2.7 | 2.5 | 2.3 | 2.3 | 1.9 | 2.2 |
Descriptive statistics
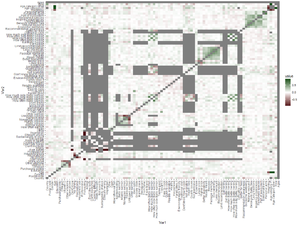
Model must contain predictors such as country, gender, age etc. Maybe we should first study what determinants are important? Model must also contain determinants that would increase or decrease fish consumption. This should be conditional on the current consumption. How? Maybe we should look at principal coordinates analysis with all questions to see how they behave.
Also look at correlation table to see clusters.
Some obvious results:
- If reports no fish eating, many subsequent answers are NA.
- No vitamins correlates negatively with vitamin intake.
- Unknown salmon correlates negatively with the types of salmon eaten.
- Different age categories correlate with each other.
However, there are also meaningful negative correlations:
- Country vs allergy
- Country vs Norwegian salmon and Rainbow trout
- Country vs not traditional.
- Country vs recommendation awareness
- Allergy vs economic wellbeing
- Baltic salmon use (4 questions) vs Don't like taste and Not used to
- All questions between Easy to cook ... Traditional dish
Meaningful positive correlations:
- All questions between Baltic salmon ... Rainbow trout
- How often Baltic salmon/herring/side salmon/side herring
- How much Baltic salmon/herring/side salmon/side herring
- Better availability ... Recommendation
- All questions between Economic wellbeing...Personal aims
- Omega3, Vitamin D, and Other vitamins
Model runs
- Model run 13.3.2017
- Model run 21.4.2017 [15] old code from Answer merged to this code and debugged
Bayes model
- Model run 3.3.2017. All variables assumed independent. [16]
- Model run 3.3.2017. p has more dimensions. [17]
- Model run 25.3.2017. Several model versions: strange binomial+multivarnormal, binomial, fractalised multivarnormal [18]
- Model run 27.3.2017 [19]
- Other models except multivariate normal were archived and removed from active code 29.3.2017.
- Model run 29.3.2017 with raw data graphs [20]
- Model run 29.3.2017 with salmon and herring ovariables stored [21]
- Model run 13.4.2017 with first version of coordinate matrix and principal coordinate analysis [22]
- Model run 20.4.2017 [23] code works but needs a safety check against outliers
- Model run 21.4.2017 [24] some model results plotted
- Model run 21.4.2017 [25] ovariables produced by the model stored.
- Model run 18.5.2017 [26] small updates
- 13.2.2018 old model run but with new Opasnet [27]
Initiate ovariables
jsp taken directly from data WITHOUT salmpling.
Amount estimated from a bayesian model.
- Model run 24.5.2017 [28]
Amount estimates directly from data rather than from a bayesian model.
- Initiation run 18.5.2017 [29]
- Initiation run 24.2.2018: sampling from survey rather than each respondent once [30]
Initiate other ovariables
- Code stores ovariables assump, often, much, oftenside, muchside, amount.
- Model run 19.5.2017 [31]
- Initiation run 24.5.2017 without jsp [32]
- Model run 8.6.2017 [33]
Other code
This is code for analysing EFSA food intake data about fish for BONUS GOHERR manuscript Ronkainen L, Lehikoinen A, Haapasaari P, Tuomisto JT. 2019.
Dependencies
The survey data will be used as input in the benefit-risk assessment of Baltic herring and salmon intake, which is part of the WP5 work in Goherr-project.
See also
- Useful information about Wishart distribution and related topics:
Keywords
References
Related files